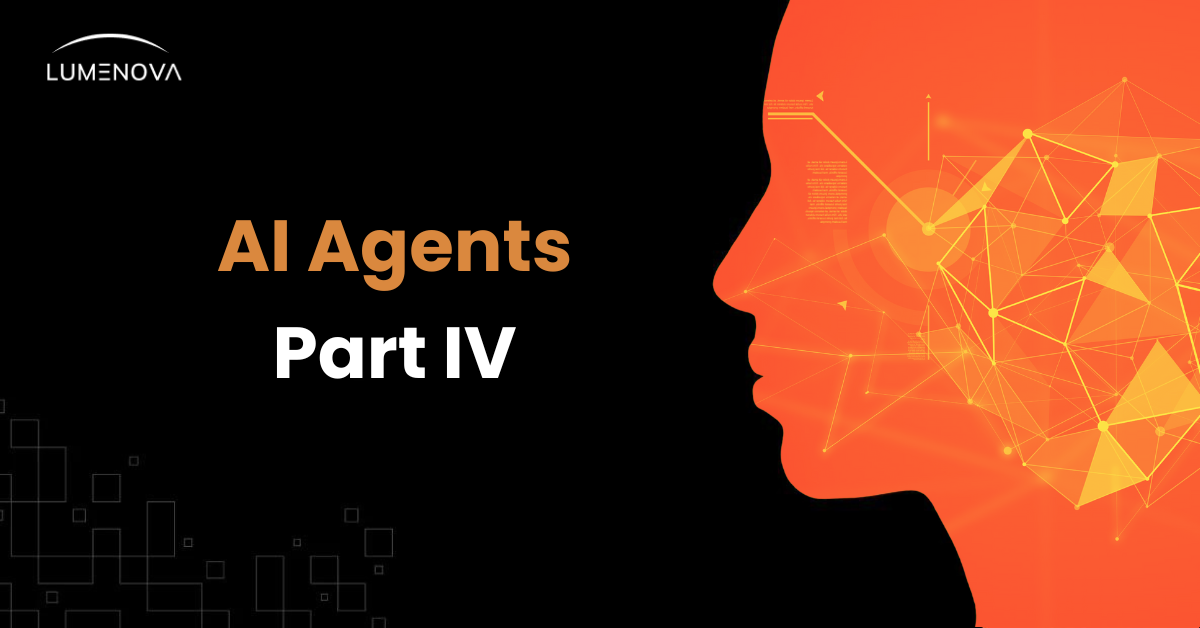
Contents
AI agents aren’t exactly a novel technology—they’ve been around for several decades in the form of symbolic and rule-based AI systems, which, historically, could only deliver limited value and utility throughout narrow contexts. Today, however, AI agents have evolved into far more sophisticated and practical systems, typically powered by generative models, and displaying capabilities that cover an increasingly complex and diverse variety of tasks across disparate domains. Nonetheless, we’re still in the early stages of the AI agent revival arc, and over the coming years (even by the end of the decade), we’re likely to witness profound changes in how this technology is applied in the real world and what it’s capable of.
Consequently, this post will speculate about what AI agents might look like in the near term—roughly ten years from now—laying out a series of predictions regarding specific use cases and capabilities improvements. Seeing as AI advances and proliferates exponentially, we advise readers to take our predictions with a grain of salt, examining them from a critical and creative point of view. Ultimately, we’re not as concerned with getting these predictions “right” as we are with prompting readers to seriously and critically envision the evolutionary trajectory of AI agents, especially in terms of how it might apply to their personal and professional lives.
Before we enter the predictive realm, we’d like to offer two important pieces of advice, which are instrumental to maintaining a realistic perspective on the evolution of AI agents, and for that matter, any AI technologies.
First, we remind readers that humans are notoriously bad at thinking non-linearly, meaning that our ability to grasp exponential innovation is limited. Overcoming this limitation, however, isn’t impossible. Via relatively straightforward tactics like analogies (e.g., doubling penny), data visualizations and interactive simulations, historical examples (e.g., Moore’s Law), reverse engineering (i.e., picking a point in the future where AI capabilities are extremely advanced and working backward), step-by-step analyses, and regularly monitoring improvements within the AI ecosystem, individuals can approximate their understanding of exponential growth while rooting it in real-world circumstances.
Second, most technologies are designed with a specific purpose in mind—whether narrow or general—but this doesn’t guarantee they will always be utilized in line with their intended purpose. In our previous post, we highlighted dual use as a crucial risk factor with AI agents, omitting a discussion of its prevalent and oftentimes positive influence on innovation. For example, GPS systems were originally developed by the US Department of Defense for military navigation purposes, but now, they form a core feature of virtually every modern car and smartphone. Similarly, Lasers were invented in the early 1960s to explore relationships between quantum mechanics and electromagnetic theory whereas today they serve numerous applications across medicine, industrial manufacturing, and military defense systems.
Moving forward, these two pieces of advice can be operationalized in the form of the following recommendations:
-
Leverage tactics like analogies, data visualizations, historical examples, reverse engineering, and regular AI ecosystem monitoring to enhance your understanding of how AI agents might evolve exponentially.
-
Don’t assume that AI agents will always be used in line with their intended purpose, and experiment with them across novel contexts and environments, provided you can do so safely and responsibly.
These two recommendations should equip readers with the mindset required to navigate the future of AI agents proactively and pragmatically, and while they won’t guarantee accurate predictions, they will cultivate a more precise conceptualization of this technology’s evolutionary trajectory. In the next sections, we’ll begin by examining several possible future use cases and then transition to an exploration of near-term capabilities improvements in agentic AI systems. We’ll conclude by briefly reflecting on our predictions and providing an additional set of actionable recommendations for readers to implement. For readers unfamiliar with AI agents, we suggest starting with part 1 of this series and then progressing from there.
AI Agents: Future Use Cases
The following agentic AI use cases have been predicted for two reasons: 1. they offer a clear utility source in some high-impact environment, innovative setting, or consequential decision-making context, and 2. they are not technologically unfeasible in light of the technologies and infrastructures we have now. This isn’t to say that other use cases won’t pop up or that our predictions will materialize exactly as we describe them—to paraphrase an earlier claim, our aim is to push readers to think creatively and experimentally about the future of AI agents, evaluating it from an innovative, critical, and disruptive perspective.
- Assisted Semi-Autonomous Design and Development of AI Systems: AI agents will semi-autonomously design, develop, modify, and improve upon future AI systems, irrespective of their type (e.g., Large Language Model vs. Image Classifier). Design and development objectives, functions and features, data and compute resource requirements and constraints, technical methodologies and techniques, and possibly even mechanisms for recursive self-improvement will be articulated, provided, or enabled by human operators working in tandem with AI agents. We favor the semi-autonomous angle because AI-powered AI creation introduces a salient loss of control risk alongside major concerns with transparency, explainability, and accountability, which are far more easily addressed if we significantly restrict how much control AI agents have within such circumstances or environments.
- Adversarial Testing and Red-Teaming in High-Risk AI Systems: High-risk AI systems must be rigorously tested for safety, robustness, and resilience in the face of adversarial attacks and/or unintended catastrophic failures. In this respect, AI agents will autonomously generate adversarial inputs to regularly uncover system vulnerabilities, simulate sophisticated multi-vector attacks and advanced persistent threats (APTs), maintain continuous yet collaborative human-AI red-teaming operations to enable adaptive learning, administer load, performance, authentication, bias, ethics, and regulatory testing, and scrutinize/mend any weaknesses in security protocols. AI agents could perform many other functions that are beneficial to ensuring the safety and reliability of high-risk AI systems, but the ones previously mentioned suffice to demonstrate the utility AI agents could deliver here.
- Autonomous Infrastructure Management: Digital and physical infrastructures like power grids, water supply systems, and transportation networks will be almost entirely operated, maintained, and enhanced by AI agents. Such agents will monitor complex system performance over time, provide actionable real-time insights into potential vulnerabilities or inefficiencies, execute maintenance tasks without human oversight, and predict possible failure modes. Real-time and/or multi-modal data processing and ingestion capabilities will also allow them to optimize various critical functions like resource distribution, emergency response, and energy consumption. In the modern age, demands for more efficient, secure, reliable, and innovative critical infrastructures are growing, and AI agents could represent a versatile high-value solution to this challenge.
- Integrated Lifestyle and Household Applications: AI agents will seamlessly integrate with an increasing variety of edge devices at multiple operating scales, from wearables like smartwatches to autonomous vehicles and entire homes. In this context, we envision a single AI agent that integrates with all of the aforementioned edge devices to manage a wide range of daily activities on behalf of users. These activities will include things like adjusting home environments to user preferences, managing travel plans, scheduling appointments, creating to-do lists, and planning workflows, providing personalized health and fitness interventions, and performing other household-related chores via remotely operated robotic devices like smart vacuums or fridges. Importantly, most of the technologies required to make this a reality already exist today.
- Human-AI Collaborative Creativity: The advent of commercially available generative AI (GenAI) systems, while it inspires notable negative implications for human creators, also fosters a wealth of opportunities. AI agents will take this one step further, working with human operators to learn nuanced artistic styles and techniques, analyze their work and provide feedback in line with pre-defined preferences, and enable a robust creative ideation process that centers on prototyping and novel idea generation. By contrast, for users who prefer a less intensive hands-on approach, AI agents will be valuable tools for artistic inspiration, the exploration of alternative perspectives, and in some cases, the ability to handle tedious tasks associated with the creative process like material selection.
- Precision Medicine and Personalized Health Interventions: The world of precision medicine and epigenetics is no stranger to AI-enabled solutions, and AI agents will dramatically expand the frontiers of what’s possible across these domains, particularly when integrated with wearable technologies. Upon receiving informed consent from users, AI agents could analyze their genetic data and health records to uncover genetic polymorphisms and pre-existing risk factors, while also monitoring risk factors in real-time via proxy metrics like blood pressure variability or cortisol levels to generate personalized and effective immunotherapies, pharmacological treatments, dietary, nutritional, and mental health recommendations, and preventive lifestyle measures that are easily implemented on a day-to-day basis. One notable challenge standing in the way of this future is the ability to scale personalized health interventions in clinical settings to demonstrate safety and efficacy.
- Novel Scientific Discovery and Experimental Design: AI agents could revolutionize the scientific lifecycle at every stage, from hypothesis generation to experimental design, implementation, and results analysis, working collaboratively with human scientists to derive novel experimental paradigms and scientific discoveries. In fact, the early versions of this technology currently exist—in 2023, a group of scientists at Carnegie Mellon developed an LLM-powered AI agent by the name of “Coscientist” that can autonomously design, plan, and execute chemistry experiments in a laboratory setting. However, Coscientist is only the tip of the iceberg, especially when considering what integrations with powerful technologies like DeepMind’s protein synthesis model AlphaFold could unlock—the future of scientific discovery and experimentation is poised to become AI-augmented in many more ways than one.
- Dynamic Social Simulation and Policy Testing: To enable proactive, agile, and innovative policy-making, policymakers will leverage AI agents to simulate social dynamics, political trends, technological advancements, economic models, environmental fluctuations, cultural behaviors, and potential change resistance among key population demographics. In doing so, policymakers will test, evaluate, and revise their policies within simulated societal-scale environments to understand their potential efficacy and limitations before real-world implementation. These simulations could also enable policymakers to account for low-probability yet high-impact/high-risk events in their policies, future-proofing emerging legislation to eliminate undue bureaucratic processes and ensure robustness. We make this prediction because policy efforts often lag behind societal and technological advancements, and AI agents represent a clear source of utility by supporting functions that are aligned with agile and proactive policy-making.
- Customizable Digital Companions: Platforms like OpenAI and Mistral AI allow users to create custom state-of-the-art GPTs/AI agents locally, whereas other popular platforms like character.ai are entirely devoted to AI character creation, strongly suggesting that a major role in the future of AI agents will be customized digital companions. However, most users will not be required to create these agents from the ground up and instead, be presented with a plethora of distinct personality profiles and behavioral trait repertoires that they can select from and easily modify to quickly build their digital companion. This digital companion will then learn and adapt to the user over time, expanding and improving upon the role the user has assigned to it, whether it functions as a “friend,” professional assistant, or personal tutor. These digital companions could eventually serve an integral function in settings like education and mental health counseling, where individuals frequently require tailored interventions.
- Environmental Preservation and Climate Engineering: Large-scale environmental projects like reforestation, ecosystem tracking, analysis, and replenishment, pollution cleanup, and sustainable irrigation, farming, and natural resource harvesting will be managed near autonomously by AI agents. Such agents will create interactive real-time models of various complex ecological systems, helping users identify and implement the best strategies for environmental restoration and conservation efforts. In more sophisticated cases, AI agents will also operate external technologies independently, controlling devices like drones, remote sensors, and other robotic appliances to execute certain environmental tasks, monitor progress on sustainability initiatives, and streamline adjustments to preservation strategies and methods in response to changing environmental conditions.
- Conflict Mediation, Negotiation, and Resolution: Particularly at national and international scales, AI agents could play a crucial role as impartial mediators, enabling adversaries to engage in meaningful and mutually beneficial negotiations that result in a peaceful settlement. By evaluating and analyzing facts, incentives, and emotional dynamics on behalf of adversarial parties, AI agents could suggest resolutions that capture all relevant interests and incentives, reducing the potency and influence of certain conflict factors like information asymmetries, shifting power dynamics, and first-strike advantages. By further analyzing and extracting insights from historical events—complex conflict scenarios in which a peaceful settlement was reached—as well as academic notions like bargaining theory, game theory, international relations, and the psychology of persuasion, AI agents could ensure that conflict mediation strategies are not only relevant and holistic, but also predicated upon existing human knowledge and experience.
- Emotionally Dynamic Entertainment: Some streaming platforms like Netflix have already experimented with innovative tactics to increase user engagement—Bandersnatch, an episode of the show Black Mirror, allowed viewers to interact with the narrative, presenting them with inflection points throughout the episode where they were asked to make a choice on behalf of the main character. In this context, AI agents will elevate emotionally dynamic entertainment content immensely, facilitating interactive and adaptive experiences that consider users’ emotional states and reactions in real time. Further integrations with virtual and augmented reality technologies, gaming and storytelling platforms, and immersive entertainment products will enable AI agents to create user-specific virtual reality narratives, modify and adjust entertainment environments, build interactive content on various platforms, and dramatically improve content personalization.
- Autonomous Management of Security and Surveillance Systems: AI agents, likely with some human oversight and guidance, will autonomously manage, control, and improve security and surveillance systems at both localized and national scales. Their ability to detect anomalous activity, and digital/physical infrastructure vulnerabilities, administer risk assessments and predictions, and coordinate and implement incident response and remediation protocols, especially when coupled with real-time multi-modal data ingestion capabilities across physical devices like cameras, sensors, and other cyber sources, makes them an attractive and dynamic solution for security, surveillance, and risk management. To this point, Lumenova AI is pioneering an agentic AI solution in the risk management space, and for readers interested in learning more about our AI Risk Advisor, we invite you to try it out AI risk advisor.
AI Agents: Future Capabilities Improvements
The use cases we’ve just discussed are somewhat speculative but nonetheless rooted in current AI capabilities and reasonable assumptions about what AI agents could do in the near term given what they can do now. By contrast, while some of the predictions in this section will meet these parameters, others will come across as much more daring and uncertain. Once more, our goal with the latter kinds of predictions isn’t to inspire controversy, misinformation, or feed the hype machine, but rather, to encourage readers to “think big” when it comes to the future of AI agents—remember that only a few years ago, you would have been laughed out of a room for entertaining the idea of Artificial General Intelligence (AGI), whereas today, we have companies whose sole mission is to create this technology (who also happen to be leading Frontier AI development efforts).
The following predictions outline a variety of capabilities improvements in future AI agents. While reading through these, we strongly encourage readers to think about the benefits and risks that these changes could inspire on localized, societal, and existential scales.
- Multi-Agent Collaboration: AI agents will autonomously communicate, collaborate, and plan for actions, strategies, and objectives with other AI agents. Multi-agent networks will function as collective intelligences, “hive minds,” or swarms whereby information is ingested, analyzed, verified, escalated, or manipulated by each relevant node (i.e., AI agent) in the network to achieve a common goal defined and set by a human operator. Within the multi-agent network, AI agents will specialize in certain functions and self-organize hierarchically to ensure goals are reached with maximum efficiency while enabling a transparent review of decision-making processes and actions.
- Dynamic Real-Time Emotional Adjustment: AI agents will leverage sophisticated emotional recognition techniques to analyze and understand users’ facial expressions, moods, vocal intonations and speech cadence, body language, eye movements, and personality types. These techniques will enable AI agents to dynamically adjust their tone, interaction style, language, and overall behavior to meet users’ emotional needs and preferences, likely before users are even aware of what their needs and preferences are. Consideration for the potential invasiveness of these systems should be afforded.
- Holistic World Modelling and Environmental Reasoning: Via simulated embodiment in virtual environments that replicate or mimic aspects of the real world, AI agents will develop comprehensive world models, becoming capable of spatial reasoning, and the ability to understand environmental dynamics, context, and physical interactions. Further improvements and enhancements could be made in real-world settings through integration with sensor-based technologies like GPS, Lidar, and cameras and collaborations/feedback from human specialists.
- Deception Detection and Truth Verification: As the digital information ecosystem becomes increasingly saturated with synthetic content, we’ll need scalable and efficient ways to differentiate fact from fiction. AI agents will be trained to identify subtle deceptive signals illustrated by linguistic cues and dog whistles, rhetorical or information inconsistencies, personalized manipulation, persuasion, and coercion tactics, behavioral nudging and psychometric techniques, and data anomalies, proceeding to cross-reference potentially deceptive information with multiple verified databases and sources to certify trustworthiness. During initial deployment stages, these agents’ performance should be monitored to ensure reliability and consistency.
- Transparent and Explainable Decision-Making: AI agents will not meet traditional transparency and explainability standards by granting humans direct insight into the functional and technical processes that lead to a certain decision or output. However, AI agents will be able to explain the reasoning behind their decisions and outputs in human terms, breaking down the assumptions made, highlighting potential areas of uncertainty, explaining their role and influence in decision-making, and comprehensively describing their decision-making logic from end to end. Humans must exercise caution in accepting these explanations without first scrutinizing their logic and assumptions.
- Zero-Shot Learning and Generalization: AI agents will be able to successfully perform tasks and analyze information that ranges beyond the data they’ve been trained on. These AI agents will infer relationships and patterns between concepts and functions, mapping them onto novel situations and problem-solving scenarios to rapidly adapt to new tasks and environments. One major challenge here will be the ability to distinguish between a genuine understanding of novelty vs. mere mimicry of understanding enabled through superficial pattern matching.
- Unsupervised Concept Creation: GenAI systems can already create new forms of data, but their abilities to autonomously develop sensical, meaningful, and useful novel concepts, categories, and representations in the absence of human guidance are still limited. AI agents will transcend this hurdle, helping humans uncover insights that were previously believed to be beyond human conception, automating various discovery processes within different domains, and adapting to experimental data and experiences. In this context, it will be vital to balance the role that humans and AI play in the discovery process to reduce the risk of stunting humans’ creative growth and experimental skills.
- Abstract Reasoning: Drawing from their capacity for zero-shot learning and generalizability, AI agents will be able to reason about abstract concepts and relationships that range beyond observable and interpretable patterns in data and experiences. By leveraging methods like knowledge graphs, theorem proving, hierarchical task networks, integration with domain-specific ontologies, and one-on-one interactions with trained human experts across disciplines like philosophy, mathematics, physics, and entrepreneurship, AI agents will be able to plan hierarchically and sequentially for long-term objectives, prove theorems or solve complex equations, and interpret metaphorical, ambiguous, or culturally relevant language across disparate facets of human communication.
- Context-Specific Ethics and Value Alignment: The ability to align AI systems with human values and preferences remains a significant challenge for advanced AI development, particularly within organizational contexts where certain value propositions may not generalize to wider societal norms. AI agents will learn to adapt their behavior in the context of their operation, reflecting the ethical norms, cultural values, key objectives, and legal requirements specific to their environment and its stakeholders, supporting flexible and dynamic ethics and value frameworks. In their early evolution, these agents may struggle to navigate situations in which values conflict or norms change quickly and unpredictably.
- Temporal Manipulation in Virtual Environments: In virtual environments, AI agents will manipulate users’ perception of time by adjusting the pace of various simulations, controlling the frequency of certain events or actions and their duration, and leveraging psychological tactics like habit formation, flow states, and mindfulness techniques to either accelerate or slow users’ temporal experience. While the benefits this technology could inspire are notably positive, especially in personalized education contexts and immersive entertainment, careful attention should be paid to the impacts of temporal manipulation on user well-being and mental health, especially addiction.
- Personalized Reality Filters and Perception Management: Through integration with augmented and virtual reality systems, AI agents will filter and categorize specific stimuli to customize, alter, or manipulate an individual’s sensory experiences within virtual or semi-virtual environments. These AI agents will help humans enhance and maintain their focus and obtain sensory relief by eliminating or suppressing distracting stimuli while also facilitating the creation of professional and home environments that reflect personal aesthetic preferences. Over-dependency and reality distortion must be monitored as key risk factors in this scenario.
- Consciousness and Self-Awareness Simulation: By far the most speculative of all our predictions, AI agents will possess the capacity for meta-cognition, continuously constructing and maintaining internal models of their actions, experiences, and states. Such AI agents will self-monitor, self-evaluate, and self-improve autonomously, changing their behaviors and objectives through introspection. To be clear, this doesn’t mean that AI agents will be conscious or self-aware, only that they will be able to successfully mimic certain characteristics associated with these qualities—human oversight will be absolutely essential in these cases, particularly for the continued assurance of value and objective alignment.
Reflection and Further Recommendations
We’ve now concluded our examination of near-term future developments across agentic AI use cases and capabilities. Reflecting on this discussion, there are a few key takeaways that readers should entertain:
- AI agents will evolve in predictable and unpredictable ways, inspiring a slew of opportunities and risks across multiple scales, many of which will be difficult to disentangle at first glance.
- AI agents are one of the most versatile, diverse, and powerful forms of AI-powered technology, meaning there are very few domains (if any) in which these tools might not be able to deliver some sort of value or utility.
- AI agents remain a nascent and largely unexplored technology, indicating that we’ve barely scratched the surface of what they can do and are likely to make profound discoveries on the use case and capabilities front within the next few years.
- AI agents are poised to play a major role in transforming the socio-economic fabric of society and democracy, forcing humanity to redefine its social, political, economic, and cultural norms, beginning with the very foundations on which they’re built.
- AI agents will initially inspire more questions than answers and solutions, and during the early stages of their evolution, finding the best ways to optimize their use within narrow or general environments, or alternatively experiment with them to discover where they’re useful, will require innovative and proactive approaches that are comfortable with uncertainty and ambiguity.
Building on our key takeaways, we also offer readers a series of recommendations intended to help them navigate and make sense of the rapidly evolving agentic AI landscape:
- Experiment with different AI agents—regardless of whether they’re purpose-built or general-purpose systems—to expose yourself to what’s out there and maintain an up-to-date understanding of agentic AI developments and innovation.
- Learn to build some of your own AI agents via platforms like OpenAI and Mistral AI to understand what makes a particular AI agent valuable within a specific context and develop the skills necessary to operate AI agents effectively and responsibly.
- View agentic AI solutions through a critical lens and do not accept them at face value to circumvent the risk of integrating a system or model that doesn’t correspond with your values, preferences, and objectives.
- Resist the urge to anthropomorphize AI agents, particularly in contexts where they personalize interactions and outputs in accordance with user preferences, to combat the tendency toward over-dependence and excessive trust.
- Use AI agents for tasks, objectives, or functions that you wouldn’t use other kinds of AI for to probe their capabilities and limitations and create a holistic view of what they’re capable of.
- Assume that you will be wrong (or at least partially correct) in your intuitions about the evolution of AI agents to avoid allocating too much time and resources to potential sources of value when several sources already exist.
- Track developments and innovations within the larger AI ecosystem, especially with Frontier AI developers, to root your understanding of AI agents in a real-world conception of AI—AI agents are just one part of the greater “AI picture.”
These recommendations, if implemented, should foster an adaptable, flexible, pragmatic, and future-oriented mindset that adequately balances lofty opportunities for innovation with real-world constraints and limitations. It will also permit readers to think beyond what’s currently deemed “possible” without getting sucked into a sea of pseudo-science, hype/doomerism, and sci-fi lore.
For those interested in exploring the first three pieces in this series in addition to numerous concepts related to GenAI, Responsible AI (RAI), risk management, and AI governance and policy developments, we suggest following Lumenova’s blog.
On the other hand, for those who have already initiated AI governance and risk management efforts, we invite you to check out Lumenova’s RAI platform as well as our enterprise AI Risk Advisor.
Frequently Asked Questions
AI Agents Series
AI Agents: Introduction to Agentic AI Models