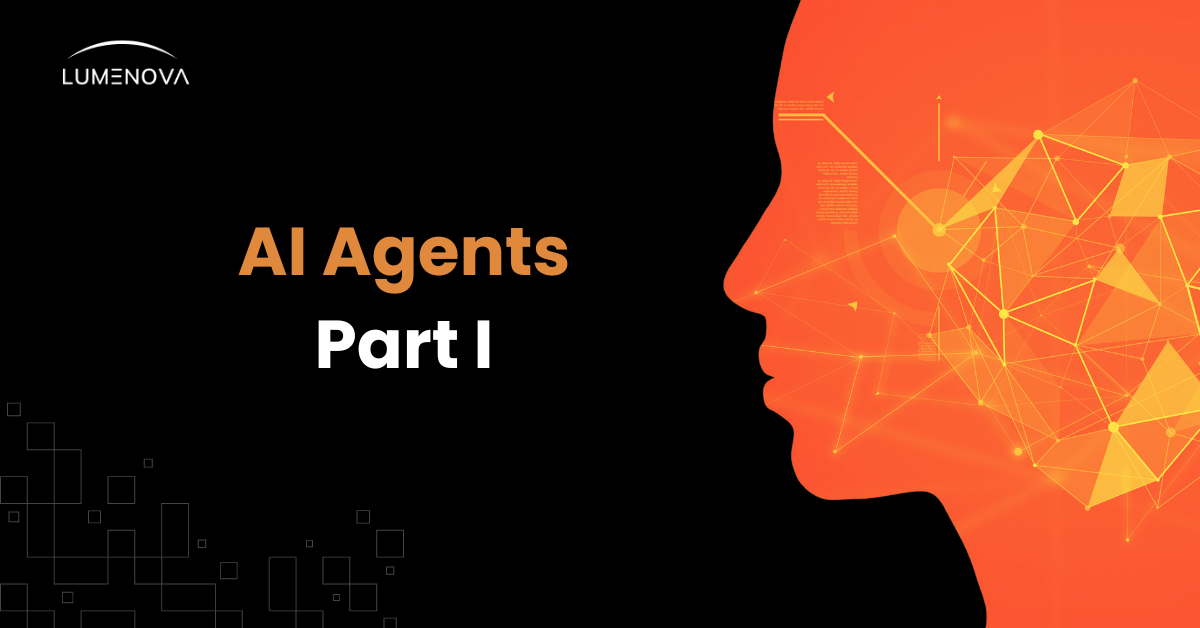
In 2023, the global generative AI (GenAI) market reached a valuation of approximately $45 billion, with GenAI applications creating $67 billion in revenue, or the equivalent of 2% of global IT revenue. By 2030, projections estimate that global GenAI market valuation will surpass 200 billion, with other notable estimates suggesting that GenAI-powered technologies and applications, when applied across industries, could add between $2.6 and $4.4 trillion in additional value to the global economy.
Despite realistic concerns about the possibility of an AI bubble in the AI investment ecosystem, GenAI innovations appear poised to continue transforming and disrupting the professional landscape, with roughly 60% of organizations (globally) justifying AI adoption efforts solely by reference to perceived productivity and efficiency gains. This technology is undoubtedly in its infancy, but industries like marketing and software development, which were among the first to adopt GenAI serve as promising early-stage case studies—over 70% of companies leverage GenAI for content creation and other marketing-related tasks, with more than two-thirds of marketers reporting positive ROIs and performance improvements, whereas 95% of developers regularly use GenAI applications to modify, refine, and generate code.
GenAI’s versatility, customizability, and in the case of general-purpose AI (GPAI) models like ChatGPT or Gemini, expansive and sophisticated capabilities repertoire, position the technology as one that easily transcends industries while permitting professionals with fundamentally different skills to optimize their workflow, skill sets, and key objectives. GenAI’s application across numerous diverse tasks ranging from content creation, risk assessment, personalized learning, and predictive maintenance to novel drug discovery, supply chain optimization, software testing, legal and scientific research, and contract analysis further drives this point home.
However, the exponential rate at which AI innovates and proliferates makes it challenging for most to keep up with the latest AI trends and developments. 6 out of 10 leaders express concerns about their organization’s ability to integrate AI effectively whereas 55% worry that they won’t be able to source enough AI talent—in 2023, only 4% of executives believed their organization possessed the necessary skills to capitalize on AI adoption efforts. Returning to the software development industry, more than 70% of developers are worried about the ability to manage AI-generated code, whereas more broadly, a mere 25% of companies claim to have executed AI initiatives successfully. Between 2023 and 2024, AI spending rates also fell by a significant margin of 30%.
The bottom line: GenAI is currently in a fragile state. Real-world benefits are beginning to materialize, clearly demonstrating GenAI’s value as a high-impact and utility tool in professional settings. However, as AI hype cycles weaken, challenges to genAI integration like skills and knowledge gaps, misaligned investments, and growing concerns for AI safety and compliance risks are coming to the forefront of the AI discourse. Moreover, the problems GenAI causes within an organization will tend not to be localized, inspiring complex problem-solving scenarios that transcend organizational functions and domains. But, what if a new kind of GenAI technology were brewing, redefining the frontier of what GenAI is capable of while also introducing the possibility for innovative purpose-built solutions to several of the problems we’ve discussed thus far? Enter, AI agents.
What Are AI Agents and How Do They Work?
Despite their apparent novelty, rudimentary versions of AI agents have been around since the 90s and early 2000s—some may remember IBM Deep Blue, an expert system trained to play chess, which eventually beat chess world champion Gary Kasparov in 1997, or the Roomba, an autonomous vacuum cleaner created by the company iRobot, whose technological successors are still used today. The 2010s further witnessed the evolution of more sophisticated AI agents in the form of virtual integrated personal assistants like Apple’s Siri or Amazon’s Alexa and autonomous robots like Boston Dynamics’ Spot. By today’s standards, however, even these kinds of AI agents are rather unimpressive given their ability to only complete relatively simple tasks within narrow domains.
To understand why today’s AI agents are so much more capable than their predecessors, we need to understand a bit more about the history of AI. The origins of neural networks and deep learning, which form the foundation of today’s most advanced AI models, can be traced back to the 1930s, but only recently have breakthroughs in compute power and hardware, model architecture, and data processing and acquisition methodologies made this technology feasible and scalable in the real world. Before these advancements, most AI systems were expert—conditional rule-based systems trained on human-expert knowledge—or symbolic AI—symbolic reasoning systems that leveraged logical rules and knowledge representations to process information and make decisions—which limited their learning, adaptation, generalization, scalability, and probabilistic reasoning capabilities.
Today, the intent to create AI agents—autonomous generalist or purpose-built AI systems capable of orchestrating complex problem-solving and decision-making processes, manipulating their environment and controlling external digital devices, adapting to novel circumstances and learning from past experiences, and displaying a multi-modal and domain capabilities repertoire—has become a reality. In fact, platforms like OpenAI and Mistral AI now offer registered users the ability to build and customize their own versions of AI agents (OpenAI calls them “customizable GPTs”) for personal and professional use, whereas several other companies, including Lumenova AI, are developing purpose-built AI agents for a variety of high-impact functions.
To make things more concrete, today’s AI agents draw heavily from state-of-the-art foundation and generative AI models—they can ingest, process, and generate information across multiple domains (e.g., audio, visual, text, code, etc.), adapt to and learn from their environments both autonomously and via human feedback, understand complex relationships between different pieces of information, respond to simple and complex natural language inputs (e.g., prompts), and in some experimental cases, even interact with other AI agents.
The technologies that make this possible include neural networks and deep learning architectures, specifically generative pre-trained transformers (GPTs), natural language processing and understanding (NLP & NLU), reinforcement learning, knowledge representation, and semantic networks, as well as distributed computing and Internet-of-Things (IoT) technologies. There are many more deeply influential technological factors here, such as advancements in compute power per unit cost, supercomputing clusters, and data generation and acquisition methodologies, although the ones we’ve mentioned suffice to outline the foundation on which most modern AI agents are built.
Why Are AI Agents Useful?
By now, it’s perhaps obvious why AI agents can serve as useful tools in many contexts. Their versatility, adaptability, sophistication, and customization make them appealing to numerous kinds of users, both technical and non-technical, while their relatively low cost and accessibility suggest they’ll be adopted across sectors and businesses of all sizes. To highlight the utility of AI agents, we describe a series of early-stage emerging use cases below, paired with real-world examples, which further demonstrate how many disparate and high-utility forms this technology can take:
- State-of-the-Art Virtual Assistants and Chatbots: One of the most common and advanced forms of AI agents, virtual assistants and chatbots like ChatGPT, Perplexity, and Gemini, can engage in complex human-AI interactions, assist with in-depth research and writing-related tasks, generate novel multi-modal content, while also performing technical tasks like code generation and debugging, data analysis and visualization, and website development, among many others. These systems possess the broadest range of capabilities, can typically be customized and fine-tuned via open APIs or built-in features, and are known to sometimes display emergent capabilities unanticipated by model developers.
- Autonomous Vehicles (AVs): While not as obvious as Chatbots, AVs can also be understood as AI agents due to their autonomous capabilities, particularly within complex environments. For example, Tesla’s Full Self-Driving (FSD) system leverages computer vision-based neural networks to recognize and classify objects, interpreting visual data to make split-second driving decisions. Similarly, Waymo’s AVs utilize LIDAR in conjunction with computer vision capabilities to navigate complex urban environments, and both vehicles can learn and adapt to new data over time to improve autonomous capabilities.
- Personalized Learning: AI-powered personalized learning is already beginning to revolutionize the educational landscape, allowing educators to obtain real-time insights into individual student performance and learning trends, develop need-based lesson and evaluation plans, and administer targeted learning interventions while streamlining busy work like grading tests and essays. Khan Academy, a well-known online learning platform, now offers educators, students, and parents the opportunity to leverage Khanmigo, which they define as a versatile personal tutor and teaching assistant. Additional interest in personal AI tutors is growing in the special education space, particularly for students with ADHD and Autism.
- Content Creation: While generalist AI agents like ChatGPT and Gemini possess content creation capabilities, purpose-built text-to-image tools like DALL-E, Midjourney, and Stable Diffusion allow users to hone in on specific image creation tasks to generate hyper-realistic photos and artistic works, advertisements and marketing materials, logos, and even pitch decks and data visualizations, all via natural language prompts. On the writing and management side, Jasper.ai has also emerged as a promising AI agent with an advanced feature suite, offering semi-autonomous project management, multi-channel communication, writing analysis and feedback, knowledge consolidation, and content generation capabilities. Sora, OpenAI’s text-to-video model, while not yet commercially available due to safety concerns, is poised to revolutionize video creation, evidenced by groundbreaking early demos.
- Scientific Research: As a high-impact domain, scientific research will benefit enormously from AI agents, both in terms of their ability to analyze, interpret, and synthesize massive amounts of structured and unstructured empirical data and perhaps more impressively, discover new science. For instance, Deepmind’s AlphaFold, a system designed to predict 3D protein structures from amino acid sequences has already predicted over 200 million new proteins. Some have even gone so far as to suggest that the future of science will involve co-collaboration between human and AI scientists, agentic AI systems that do much more than data analysis and synthesis, designing and administering novel experimental paradigms, autonomously adapting to and bridging scientific knowledge gaps, and writing and researching scientific papers from end-to-end.
- Sustainability: AI agents are making substantial impacts across various sustainability-oriented sectors, including environmental impact management, disaster prediction, sustainable agriculture, and energy-efficient building and design processes, to name a few. Bayer, a German life-sciences company, has developed an “agronomic advisor”, an LLM trained on years' worth of aggregated proprietary agricultural data and expertise, providing farmers with agronomic recommendations on materials and resource selections alongside other sustainable farming methodologies. Alternatively, the AI start-up Contilio is making major strides in the construction industry, offering a genAI-powered platform that enables builders to create 3D digital twins of their projects, to assess factors like CO2 emissions and maintenance costs.
- Gaming: While still in the early development stages, increasingly sophisticated digital environments now allow AI developers to experiment with gaming AI agents that can learn hundreds of different tasks within virtual settings via direct human input, game playing, and adaptive learning. In March of this year, Google announced SIMA, a generalist AI agent that can perform over 600 simple tasks within simulated environments—the future of gaming and virtual reality will likely involve multi-AI-agent scenarios in which AI characters autonomously interact with humans and other AIs, learning and adapting to their experiences.
- Healthcare: AI affords a wide array of benefits within a healthcare setting from personalized treatment recommendations, enhanced image-based diagnostics, and novel drug discovery and development to medical training simulations, disease outbreak prediction, and the streamlining of administrative tasks like billing and claims processes. More recently, companies like CodyMD and Docus, have built interactive AI doctors that potential patients can leverage to understand and treat their symptoms from home, locate and connect with local specialists, human doctors, and medical providers, and order prescriptions. These applications are intended to help people quickly gain the medical advice and insights they need, and in cases where human intervention is necessary, provide individuals with avenues through which to access the healthcare services they require.
- Cybersecurity: Cybersecurity is a sector that will continue to reap enormous benefits from advanced agentic AI systems, leveraging them for predictive threat intelligence, automated incident response procedures, phishing detection, vulnerability management and adversarial testing, and security information and event management (SIEM), including several other functions. For example, IBM’s AI-powered security platform can autonomously analyze cyber security incidents and quickly provide actionable incident response recommendations—Darktrace’s Active AI Security platform performs real-time analysis of network traffic patterns to proactively pinpoint potential cybersecurity threats and develop relevant solutions—Zimperium’s Mobile Threat Defense (MTD) system performs real-time threat intelligence analytics via on-device monitoring to detect and respond to phishing attempts, device attacks, malicious applications, and network vulnerabilities.
- Virtual Societies: As AI agents are increasingly popularized in gaming and virtual reality sectors, and prominent AI platforms like Character.ai continue to provide users with the opportunity to create AI agents with specific personalities and behaviors, we may begin witnessing the emergence of virtual AI societies in which humans and/or AIs communicate with and form relationships with other AI agents. Setting aside palpable ethical concerns for a moment, Stanford and Google actually conducted a virtual society experiment in 2023, where using ChatGPT, they created 25 AI agents with distinct personalities and behaviors, leaving them to their own devices for two days. Upon reevaluation, they found that the characters had formed relationships with each other, had opinions and gossiped, performed daily duties diligently, remembered past events, and planned for future ones.
The domains we’ve covered here don’t even come close to representing all the possible application contexts for AI agents, however, they strongly highlight the versatility and utility of this technology across disparate sectors and domains.
It’s also worth noting that Lumenova AI is currently pioneering AI-powered custom solutions for AI lifecycle risk management and compliance, and AI model technical evaluation, monitoring, and improvement. Our solutions target multiple industries including health and life sciences, insurance, banking and investment, technology and telecommunications, HR and recruitment, and consumer goods. Moreover, just this month, Lumenova also announced its AI risk assessment agent, directly addressing a crucial emerging need among organizations of all sizes for robust and easy-to-use AI risk assessment, management, and mitigation tools.
Conclusion
After reading this post, readers should have a pragmatic understanding of the technological foundations on which AI agents are built, their core properties, and their overall utility and versatility. AI agents, while they draw from several other kinds of AI technologies and techniques, are a specific form of generative AI technology, loosely defined by their ability to autonomously navigate, learn from, and adapt to complex and evolving decision-making scenarios and environments. By now, it should also be clear that the purpose of AI agents isn’t to replace humans—in all of the real-world use cases we covered in the previous section, AI agents assumed the role of advisors, assistants, tutors, or simply systems with which humans can interact in a human-like fashion to gain actionable insights, recommendations, or solutions for the problems they face (we’ll discuss AI agent risks in part 3 of this series, so stay tuned).
To this point, our next piece in this series will explore, in detail, a selection of potential AI agent use cases throughout various domains. Although hypothetical, these use cases will not make any technological assumptions that aren’t rooted in currently available technologies, or alternatively, feasible in light of current AI developments. Furthermore, these use cases will examine at a granular level, what processes, procedures, or tasks an AI agent might perform in targeted high-impact contexts.
For readers interested in exploring other topics in the generative AI landscape, alongside additional developments in the responsible AI (RAI) ecosystem, AI risk management, and AI governance, we recommend following Lumenova AI’s blog, where you can regularly access in-depth material on all these topics.
For those who are beginning to explore, develop, and implement AI governance and risk management strategies, protocols, or frameworks, we invite you to consider Lumenova AI’s RAI platform and book a product demo today.