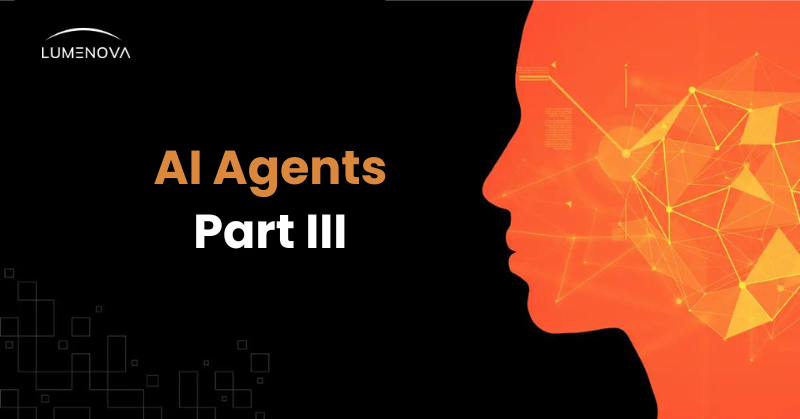
Contents
Building a comprehensive understanding of the risks agentic AI systems inspire is challenging in light of the technology’s novelty, versatility, and customizability. Consequently, deconstructing the AI agent risk repertoire necessitates broad consideration of many critical factors. These factors include things like the intended use and purpose of the agent, the real-world objectives or tasks for which it’s leveraged, the rate at which it adapts to and/or learns from new experiences or data, its respective capabilities and limitations, the degree of involvement in consequential decision-making procedures, how well it integrates with existing digital tools and infrastructures, and the concrete skills required to utilize it responsibly and effectively.
While there are additional important risk factors to consider, the ones we’ve just highlighted demonstrate why context-agnostic approaches to AI agent risk management are likely to fail. In truth, this notion applies to virtually all other kinds of AI systems as well, but seeing as AI agents are designed to execute decisions, tasks, or objectives autonomously and, ideally, in the absence of human oversight, the stakes are higher and even closer attention must be paid to their use contexts to mitigate foreseeable risks.
Take simple-reflex agents, which, given their relative simplicity, might be viewed as inherently less risky than their more advanced counterparts—for example, learning or hierarchical agents—due to limited capacity for adaptability, learning, and complex problem-solving, among other high-level capabilities. However, if a simple-reflex agent were used for military purposes, like an autonomous missile defense and counterstrike system, a single erroneous threat detection could trigger a counterstrike response that elicits an all-out war between two powerful adversaries, especially if both adversaries utilize autonomous defense systems. Surely, this example represents an extreme case, but it also drives home the following point: the risks inspired by AI agents are heavily influenced by the environment in which they’re used.
However, it’s also worth noting that more complex and capable variations of AI agents will generate value and utility across a wider range of tasks, objectives, or domains. This phenomenon makes them appealing tools to numerous kinds of actors, some of which will have malicious intentions while others might simply not possess the skills or knowledge necessary to operate them safely. Increasingly advanced AI agents are deeply prone to misuse, making it easier for less sophisticated bad actors to orchestrate scalable high-frequency threats, or alternatively, through accessible user interfaces, versatile capabilities, and seemingly high-quality outputs, building misplaced trust and reliance among users with insufficient skill sets.
To summarize, establishing a clear, targeted, and holistic view of the risks inspired by specific forms of AI agents requires, at the very least, a detailed understanding of a. the context or environment in which the agent is leveraged, for what purpose, and by whom, b. how complex and advanced the agent is, namely in terms of its capabilities, limitations, and technical vulnerabilities, and c. the continued maintenance of the skills necessary to leverage it responsibly and effectively. These three characteristics form the foundation for managing the risks associated with AI agents, and if they are pursued meticulously, should capture all of the risk factors we mentioned at the beginning of our introduction, particularly throughout cases where the possibility of misuse is prevalent.
In the following sections, we’ll break down agentic AI risks into four categories, each of which discusses a selection of specific risk factors—for those interested in examining the systemic and existential risks (x-risk) inspired by AI agents and other AI technologies, we recommend reading through our systemic and x-risk series in addition to this post. We’ll conclude by offering actionable recommendations for managing agentic AI risks in real-world settings. If you’re unfamiliar with AI agents, we strongly advise reviewing Part 1 and Part 2 of this series before proceeding further.
AI Agents: Risks
This section examines agentic AI risks across a few core categories: 1. decision-making, 2. psychological impacts, 3. misuse and overreliance, and 4. operational vulnerabilities. We’ve chosen these categories for two reasons. First, they are relevant throughout the majority of industries, domains, and sectors, as well as most businesses and civil society. Second, they represent the areas in which we can reasonably expect to see the first notable agentic AI risks and impacts materialize. There may, of course, be other areas of relevance but in the interest of keeping things succinct, practical, and not overly speculative, we’re sticking with the ones we’ve just mentioned.
Moreover, the risks discussed throughout each of the categories we specify can vary in severity, probability, and intractability depending on the nature of real-world use cases, their scale, and ongoing technological developments. We, therefore, advise readers to maintain a neutral yet critical perspective while thinking about agentic AI risks. For example, a highly salient risk with severe potential impacts might garner serious attention, but if the probability of its materialization is marginal at best, other risks should be prioritized accordingly—naturally, if a potential risk is severe enough (e.g., extinction), regardless of probability, all steps to manage it should still be taken. We recognize this might be a confusing notion to readers, but it is crucial to examine risks with this kind of nuance and critical judgment to comprehend their full scope and context realistically.
Decision-Making
Decision-making risks arise when agentic AI systems assist with, drive, or execute decisions on behalf of humans, either in isolated cases or at larger scales, like an entire organization. In the responsible AI (RAI) discourse, decision-making risks are at the forefront—the potential for bias and algorithmic discrimination, especially across high-impact domains like finance and healthcare, has garnered significant attention, both publicly and privately. However, seeing as we’ve explored these issues in depth in other posts, the decision-making risks we’ll be discussing here are those that a. are unique to AI agents, or b. might be less obvious to readers.
-
Distributed Responsibility: Understanding who to hold accountable when agentic AI systems orchestrate harmful decision outcomes is difficult, particularly when AI agents comprise a part of a larger interconnected system, in which both human actors and AI systems play some role in decision-making. This is further complexified by the fact that AI agents might be partially or wholly responsible for certain decisions or decision-making processes, and if concrete decision-making parameters aren’t identified, the line between human and AI-driven decisions becomes increasingly blurred, especially as agentic AI systems take on more or less responsibility in light of emerging capabilities and limitations.
-
Misaligned Objectives: If an organization or individual fails to consider how well the objectives of an AI agent align with their own, AI-driven decision outputs could fail to capture user preferences, values, and goals adequately, perpetuating faulty decision-making that can rapidly spiral out of control. Specific decision-making tasks necessitate purpose-built AI agents that are sufficiently customized and fine-tuned, and even when all possible technical measures are taken to ensure objective alignment, modifications or changes made to a system could quickly lead to disruption.
-
Emergent Behaviors and Veiled Objectives: AI agents, depending on their degree of sophistication and complexity—those that are based on foundation models—are prone to displaying emergent behaviors (behaviors that are unintended by developers) and/or veiled objectives (objectives that are concealed from model developers or users). Combatting this possibility is extremely challenging when considering the transparency and explainability limitations that advanced agentic AI systems encounter, particularly as they scale, adapt to changing environments, and learn from new experiences.
-
Data and Goal Drift: If AI agents are leveraged for decision-making tasks on which they haven’t been sufficiently trained, they may generate erroneous, unreliable, or inconsistent outputs—this is data drift. By contrast, as AI agents are trained on new data to accomplish either more complex tasks or a wider variety of tasks, their predefined goals may begin to shift as they learn from and adapt to new data and experiences—this is known as goal drift, which is likely to result in objective misalignment if not immediately addressed.
-
Algorithmic Determinism: Where rudimentary AI agents with limited adaptive and learning capabilities are leveraged for decision-making, the inability to account for the dynamics of changing environments, new decision-making parameters, thresholds, and metrics, nuanced judgments that require human intervention could result in algorithmic determinism—overly rigid decision-making processes that fail to account for all relevant factors.
-
Hijacking and Jailbreaking: Just like any other AI system, AI agents are vulnerable to actors with malicious intent. Via methods such as prompt injection, data poisoning, and model inversion, agentic AI systems can be hijacked to manipulate, reverse-engineer, or orchestrate harmful decision outcomes. In other cases where developer-built safety parameters and guardrails are insufficient, malicious users could jailbreak AI agents via advanced natural language prompting techniques like Skeleton Key, causing AI agents to ignore safety parameters and guardrails when generating future outputs.
-
Destabilizing Feedback Loops: Agentic AI systems can make decisions at much higher frequencies than humans. This introduces the risk of decision-making feedback loops that begin with one erroneous decision that is then amplified successively throughout subsequent related decision-making processes, perpetuating a decision-making failure cascade. If these loops aren’t caught and addressed in time, they could easily destabilize an entire decision-making function within an organization, causing key teams and personnel to take real-world actions that result in poor, misaligned, or harmful outcomes.
Psychological Impacts
By psychological impacts, we mean the behavioral and cognitive effects that interactions with AI agents could produce on humans. Currently, these effects are not well-studied in the research sector, and while empirical efforts to understand them are underway, we hope that by identifying several key psychological risks, we’ll help inspire further research on human-AI interaction, especially as it concerns AI agents.
-
Compromised critical thinking, independence, and problem-solving: Advanced AI agents, specifically those with multi-modal input-output mechanisms and generalist capabilities repertoires, can serve as powerful tools for capable AI users. Those who understand how to exploit such agents to their full extent can streamline critical thinking and problem-solving initiatives, leveraging agentic AI systems to retrieve and analyze complex information quickly and effectively, break down problems into actionable steps and objectives in a context-specific manner, and identify shortcomings or incorrect assumptions in their thought processes, among many other benefits. However, if users don’t continue to train their critical thinking and problem-solving skills in real-world settings, they may find themselves in a position where AI-induced cognitive laziness has compromised their capacity as independent thinkers.
-
Altered Information Processing: Sustained interactions with AI agents could dramatically alter how humans process information over time. For instance, in cases where AI agents optimize content delivery procedures, such as by curating short-form information summaries, users’ attention spans could decline as they habituate. Similarly, where AI agents generate content, regardless of the medium it takes, users might find it increasingly difficult to distinguish between AI-generated and human content, especially as the former progressively saturates the larger digital information ecosystem. In other cases, AI agents could make information retrieval, synthesis, and analysis so effective that humans actually feel overwhelmed by all available information, resulting in information overload.
-
Insecure Attachment and Social Isolation: Where AI agents are designed to mimic human-like personalities and behaviors, users may form insecure attachment bonds, anthropomorphizing such agents as their “friends” or “partners”. This risk grows as AI agents evolve into systems with greater personalization capabilities, whereby each individual interaction with a user is customized with respect to their preferences and previous interactions. Further down the line, users could become so attached to these agents that they actively begin ignoring or disliking social opportunities with other humans, operating under the false belief that agentic AI systems will be able to fulfill their social needs—in other cases, users’ may begin conflating their identity with that of the agents with which they interact.
-
Emotional Dysregulation: If AI agents are leveraged for mental health purposes, like alleviating loneliness or helping psychiatric patients cope with mood swings and self-regulate emotional outbursts or inconsistencies, long-term use could result in severe emotional dysregulation. Over time, frequent users of these agentic AIs might lose their natural ability to cope with their emotions, struggle to make sense of the emotional interventions they’ve undergone, and excessively rely on external systems for managing their moods. In more extreme cases, users might even begin to distrust human professionals in favor of AI agents, falsely believing that AI agents “know” and “understand” them better.
-
Altered Perception of Self: Where agentic AI systems are leveraged to provide feedback to users or conduct analyses on their behalf, users could develop a profound sense of insecurity and self-doubt, driven either by overreliance on AI-powered personality and skills assessments or the constant need to compare oneself to AI capabilities. In the long term, this phenomenon could cause users to feel as though their autonomy and agency have been deeply threatened, giving rise to feelings of purposelessness, loneliness, incompetence, and lack of drive or motivation.
-
Bias Amplification: Users may inadvertently amplify their biases through interactions with AI agents by perceiving them as trustworthy, reliable, and consistent. Even if AI agents work as intended, satisfying trustworthiness, reliability, and consistency requirements, it’s hard to imagine how they could possibly internalize all the nuances of human judgment and experience, especially across nebulous cultural or societal concepts or throughout changing environments. Consequently, when users arrive at a conclusion or solution that aligns with an AI agent’s output, they might automatically assume they are correct. Alternatively, if an AI agent’s output contradicts a user, the user might simply refer to a different AI agent that generates a response they like—AI agents are proliferating rapidly, allowing users to pick and choose which ones they prefer, a process that is unlikely to be objective.
-
Disrupted Relationship Dynamics: As AI agents play an increasingly prevalent role in professional collaborative settings, they could alter the nature of human-human interactions. Team members might develop unrealistic expectations about their coworkers, trust AI agents more than human counterparts, restructure teams so that they revolve around agentic AI systems, and in some cases, even begin valuing AI agents as core team members.
Misuse and Overreliance
While conceptually interrelated, misuse and overreliance are individually distinct terms. Misuse encapsulates when AI agents are used incorrectly or maliciously, in ways that either don’t correspond with their intended purpose, capabilities repertoire, and/or real-world use context. On the other hand, overreliance occurs when human actors come to rely on AI agents excessively, to such a degree that if the AI agent were to catastrophically fail, a human actor wouldn’t be capable of assuming the tasks or responsibilities previously carried out by the agent. In some cases, overreliance can lead to misuse—certain skills are also required to leverage AI agents effectively and safely—and in others, misuse can lead to overreliance—if human actors are using AI agents for tasks they are responsible for performing independently.
-
Automation Bias and Skills Degradation: Automation bias—the tendency to rely excessively on progressively capable AI systems—is a major risk factor with AI agents, particularly those that are purpose-built to execute specific tasks or objectives. While the tasks and objectives that AI agents typically accomplish will likely be those that are considered mundane, time-consuming, and/or dangerous by humans, humans should ensure they still possess the necessary skills to accomplish said tasks in the event of catastrophic AI failures. Where advanced generalist AI agents are used, users must be conscious of the potential for cognitive laziness, which could, over time, lead to widespread skills degradation.
-
Moral and Social Deskilling: When it comes to moral and social decision-making and judgment, AI agents are fundamentally limited by their inability to internalize the fine details and vagaries of human society, culture, morality, and phenomenological experience, and users are, at least to some degree, responsible for being aware of these limitations—this is why we classify this risk under misuse and overreliance vs. psychological impacts. Furthermore, users who rely on agentic AI systems for these functions are likely to be misled or redirected toward socio-moral assumptions and conclusions that don’t reflect reality—reality is often muddled by socio-moral “grey areas”. This risk is further amplified by our anthropomorphic tendencies as humans, which are easily projected onto AI agents, especially those designed to mimic human personalities and behaviors.
-
Dual-Use: Dual-use is a tangible risk factor with purpose-built agentic AI systems, although it’s arguably more important to address with generalist AI agents due to their expansive capabilities repertoire, accessibility, adaptive learning abilities, and ease-of-use—at this stage, a generalist agent might be expertly proficient at some tasks and mediocre at others, but this is still inherently riskier than a purpose-built AI agent that’s an expert at one single task (provided that task isn’t something like synthesizing pathogens that could be used to manufacture bioweapons). Moreover, in advanced AI agents, emergent capabilities and objectives can sometimes be double-edged swords, wherein despite being useful, they can also be easily exploited for harmful purposes, typically where existing safety parameters and guardrails fail to account for them.
-
Skills Non-Transferability: Most AI agents are operated via natural language (i.e., prompting), and this can create the illusion that the skills necessary to operate one agent effectively are transferable to other agents. While this may be broadly true, anyone who’s experimented with more than one state-of-the-art generative AI (GenAI) model will quickly realize that even if different models are prompted with identical prompts, outputs will rarely be similar, both in terms of content and utility, which is why we see some models outperform others on certain tasks that they’ve all been trained on. The skills required to utilize an AI agent well range beyond prompting to include an in-depth knowledge of what the agent is intended to be used for, its real-world use case, and its capabilities and limitations repertoire.
-
Trust Erosion: In the AI discourse, trust erosion is most commonly discussed in terms of AI systems that perform inconsistently, unreliably, or non-transparently, thereby eroding trust among users. While this point applies to AI agents as well, the trust erosion we examine here relates to human specialists. For instance, if an AI agent designed to provide medical recommendations based on imaging diagnostics has an accuracy rate of 99% while a human doctor maintains an accuracy rate of 70%, patients could be incentivized to distrust the human doctor, who is actually taking into account a much wider array of health factors when determining what medical intervention is best. This trust erosion is more likely to crop with AI agents whose intended purpose is to perform a single complex task with expert—likely superhuman—proficiency in tandem with a human specialist.
-
Compulsive Information Seeking: As the global digital information ecosystem becomes even more abundant, AI agents will play an increasingly notorious role in helping humans dissect, interpret, analyze, and summarize the wealth of information at their fingertips. This could cultivate an “every question has an answer” mindset among AI users where they experience a compulsive need to seek out information, even when they objectively have no utility for it—for example, asking Siri to give you directions to the coffee shop near your house, despite having made the trip numerous times. This compulsive tendency could also further perpetuate a perceived notion of information scarcity, leading to feelings of anxiety and insecurity in situations where users believe they don’t have all the information they require (even though they do) to complete some task or reach an objective.
-
Manipulation of Reality: We’ve already discussed how AI agents could be hijacked and jailbroken to manipulate decision-making outcomes. However, AI agents can also be powerful tools for mass manipulation and coercion, particularly if they are replicated and offloaded in swarms on digital platforms like social media sites, news outlets, and online forums—this phenomenon already exists in the form of “bots”, which are far less sophisticated than AI agents. For instance, a swarm of AI agents could analyze political trends within a major social media platform before an election, and then identify and target users with undecided political views throughout key demographics. These AI agents could then fabricate false persuasive content that nudges users toward or away from a specific political candidate—once users get a hold of this information, many will share it throughout their networks, propagating it naturally before anyone can verify whether it is true.
Operational Vulnerabilities
Operational vulnerabilities emerge when AI agents are leveraged, integrated, or developed irresponsibly within organizational contexts. By organizations, we mean everything from start-ups and SMEs to major enterprises, non-profits, and government agencies.
-
Auditability and Compliance: AI agents powered by foundation models will present significant challenges to auditors due to their opaque decision-making processes and lack of transparency. Where AI agents are leveraged for consequential decision-making, further compliance difficulties could emerge if those subject to AI-driven decision outcomes choose to exercise certain rights like the right to request an explanation of the decision made, or the right to request an explanation of the models’s decision-making criteria. Auditing challenges could also perpetuate downstream impacts on other core responsible AI best practices, like human oversight and accountability—if we can’t clearly explain how a model arrived at a given output, monitoring model performance, especially when things don’t go as planned, requires some serious ingenuity.
-
Failure Cascades: When AI agents are part of a larger interconnected system, a single erroneous decision, recommendation, or output could trigger a failure cascade that penetrates each component of the system, resulting in destabilization or even catastrophic failure of the whole. Where the AI agent sits in the system’s hierarchy, how many other tools, functions, or systems it controls, how reliable and consistent the agent’s performance is over time and throughout changing environments (robustness), how resilient the agent is to adversarial attacks, how closely the agent’s objectives are aligned with those of the organization, whether it interacts with any other agentic AIs autonomously, the degree of human oversight, validation, and verification—each of these factors is critical to address when examining how to prevent and mitigate potential failure cascades.
-
Over-Optimization: Where AI agents are leveraged for optimization purposes like enhancing workflow efficiency, managing supply chain bottlenecks, and allocating enterprise resources sustainably to key business functions, over-optimization remains a potent risk factor. Agentic AI systems might optimize for seemingly benign, irrelevant, or harmful goals, pursue actions or processes that meet optimization criteria but don’t reflect the broader purpose or mission of an organization, overgeneralize on novel tasks that fall outside of their training data, and become progressively less flexible as they fail to adapt to new data and experiences. The consequences of over-optimization could reverberate throughout an entire organization, although they are most likely to arise in resource-driven operational decision-making domains like product development and lifecycle management, enterprise resource planning, and inventory management and logistics, to name a few.
-
Integration Challenges: Where an organization aims to integrate one or multiple AI agents with an existing digital infrastructure, it will likely have to overcome integration challenges. Outdated legacy systems might not be compatible with advanced AI agents for several reasons, including non-readable data formats, archaic system architectures, and limited or nonexistent API capabilities. Further issues with data management, such as data silos—where data is scattered across several functions and departments—and data quality, could hinder an organization’s ability to consolidate unified high-quality datasets for AI training and development purposes. These issues can also introduce data security and privacy vulnerabilities—when organizations train or fine-tune agentic AI systems with proprietary datasets, these systems become assets, cementing their status as potential high-value targets for bad actors.
-
Lack of AI Training: Agentic AI systems, though they can complete pre-defined tasks and objectives autonomously, still require human input and guidance to function safely, reliably, and effectively—AI literacy is critically overlooked and undervalued. Users must cultivate a robust understanding of how to formulate model-specific and informative queries, wherein the context of the problem, task, or objective is neatly articulated and detailed, parameters for the model to follow are described coherently, and examples of desirable outputs are provided, if necessary. In cases where AI agents are leveraged for more complex purposes, users must develop the skills required to elicit high-utility outputs via long-form iterative interactions, and where agentic systems display generalist capabilities, understand which capabilities to leverage and why.
-
Cultural Resistance: With any new and powerful technology integration initiatives, organizations can expect to encounter cultural resistance—people tend to resist change due to fears of obsolescence, uncertainty, and risk. With agentic AI systems, these fears are amplified, since these systems are already out-performing humans across numerous tasks, with the potential to do so across many more. To circumvent cultural resistance, organizations must provide their key teams and personnel with the resources, training, and upskilling initiatives they require to augment their professional performance with AI agents, rather than living in fear of being replaced or outpaced by them.
-
Non-Adaptive Management: As AI agents are more heavily integrated into collaborative and team environments, organizations will need to redefine their management strategies to account for emerging changes in workflow dynamics and processes, communication styles, risk management approaches, employee accountability and corporate social responsibility frameworks, and compliance requirements. Importantly, organizations should also closely and consistently evaluate potential changes in the frequency and intensity with which tasks are completed in tandem with or solely by AI agents, modifying their objectives and key results benchmarks accordingly. Broadly speaking, AI integration is an accelerating process, whereby both benefits and risks can materialize far quicker than most would expect.
Managing Agentic AI Risks: Recommendations
In this penultimate section, we list and describe a series of actionable recommendations, applicable to individuals and organizations, for managing the risks inspired by agentic AI systems. These recommendations are intended to be pragmatic, easy to implement, and interoperable with existing technical and regulatory requirements. We illustrate these recommendations below:
- Build AI literacy to create an AI knowledge base that enables you to differentiate between AI agents in terms of their intended purpose, capabilities and limitations repertoire, potential risks and benefits, and the skills required to operate them effectively within specific contexts or environments. This foundation will help you identify where a given AI agent can augment your workflow or generate value for you without introducing avoidable risks or pitfalls.
- Experiment with various AI agents across multiple domains to find the system that is best suited to your needs, preferences, values, objectives, and workflow processes. Experimentation will also streamline your AI literacy learning curve while fostering an understanding of how to leverage multiple AI agents successively or in tandem to overcome limitations in each respective system or expand the number of tasks you can accomplish.
- Embrace AI agents, despite the fears you may have about them. If an agentic AI system outperforms you on a task or objective—which it likely will at some point—view this as an opportunity rather than a threat to your livelihood. Push yourself to think bigger and more creatively, expanding your skills repertoire with every chance you get while exploring how AI agents can help you accelerate this process.
- Before integrating an AI agent into your workflow, examine whether its intended purpose, objectives, and capabilities repertoire align with your intended tasks, objectives, problem-solving goals, technology requirements, and work environment. While perfect alignment isn’t necessary, you should ensure that, at the very least, agentic AI integration doesn’t introduce vulnerabilities and risks that range beyond your ability to mitigate them appropriately.
- Ask yourself if you truly need to use an AI agent to accomplish a task, objective, or problem-solving goal. You should ask yourself this question regularly while leveraging AI agents to ensure that you’re not falling prey to overreliance, compulsive information seeking, or AI-induced deskilling. Periodically evaluate your skills independent of AI over time to guarantee they remain fresh and up-to-date.
- Maintain a healthy skepticism of agentic AI outputs and behaviors to reduce the risk of AI-driven failure cascades, poor decision-making, cognitive laziness, and trust erosion. You don’t need to critically examine every single AI agent output and behavior, you just need to regularly remind yourself that AI can and will be wrong sometimes.
- Don’t assume that AI agents will remain the same, otherwise, you risk overlooking potential emergent capabilities and objectives—which could be both useful and risky—failing to account for misaligned objectives, data, and goal drift, and possible adversarial and jailbreak vulnerabilities. Evaluate the performance of your AI agents at regular intervals to gain insights into their evolution, and ensure that your evaluation metrics and thresholds reflect the context in which the agent is operated.
- Be wary of the natural human tendency to anthropomorphize. Anthropomorphization remains a possibility irrespective of the environment the AI agent is leveraged within or the purpose for which it’s used. Be mindful of the language you use when interacting with the agent, and ask yourself honestly whether certain emotional dynamics are at play that shouldn’t be.
- Monitor the environment in which the AI agent operates to account for environment-specific limitations and changing environmental dynamics. This will help you identify and address potential vulnerabilities in your agentic AI system, modify your AI agent appropriately when its operating environment changes, and continuously build an in-depth understanding of its operating environment, which will inform further modification and risk management efforts.
- Consider what kind of AI agent you’re using. In our previous post, we described the various forms AI agents can take—comprehending these forms will help you determine what kind of AI agent you should use within a specific context or for a given task or objective. More broadly, it will also enable you to identify, categorize, and prioritize the risks posed by the agent while deepening your knowledge of the limitations it may encounter.
Conclusion
We’ve now covered several of the key risks linked to AI agents, and as AI agents steadily embed themselves in our professional and personal lives, we can expect this risk repertoire to expand significantly. However, we’re optimistic about the future opportunities and benefits AI agents could afford, and we think that as these technologies become more widely integrated and used, their risks and limitations will surface, laying the groundwork for proactive risk mitigation strategies. If we could offer one piece of advice to readers, it would be this: use and experiment with AI agents to figure out what they’re good at and where they fall short.
In our next and final piece in this series, we’re going to explore a more speculative and controversial topic: the future evolutionary trajectory of agentic AI systems. In doing so, we hope to inspire readers to critically examine the nature of the AI-driven future they want to be a part of while also giving them some of the tools they need to prepare for the impacts that future AI agents could generate across all walks of life.
For those interested in exploring additional topics in AI risk management, governance, RAI, and GenAI, we suggest following Lumenova AI’s blog, where you can continue to track the latest developments across each of these domains.
Alternatively, for those who have already begun developing and implementing AI risk management and/or governance frameworks, policies, standards, and protocols, we invite you to check out Lumenova’s AI Risk Advisor or RAI platform and book a product demo today.
Frequently Asked Questions
AI Agents Series
AI Agents: Introduction to Agentic AI Models