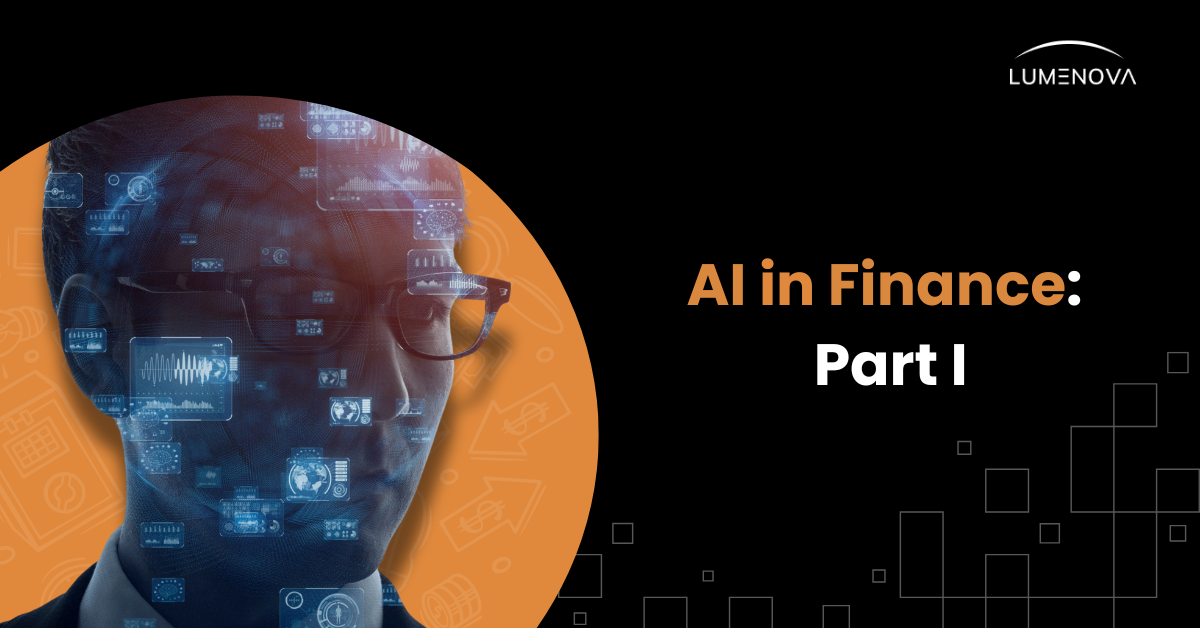
Contents
As artificial intelligence (AI) continues to evolve, its integration into financial services has become inevitable, with Retrieval Augmented Generation (RAG) emerging as a key focus in this transformation. By combining real-time data retrieval from industry-specific sources with generative language models, RAG delivers responses that are both accurate and contextually relevant.
However, while RAG holds immense promise, its application in finance must be handled carefully. Financial institutions rely on precise, real-time data, and improper implementation of RAG could expose them to significant risks, including compliance failures and security vulnerabilities.
This article is the first in a series exploring AI’s role in finance, with RAG as the centerpiece. Here, we’ll discuss how RAG can be implemented responsibly, considering its benefits and the potential risks. In the next installment, “AI in Finance: The Rise and Risks of AI Washing (Part II),” we’ll dive into the concept of AI washing, its parallels to greenwashing, why it’s problematic, and how it impacts corporate finance, offering actionable insights to identify and combat misleading AI claims.
Understanding RAG in Finance
RAG’s hybrid approach combines the capabilities of retrieval-based systems, which access real-time, domain-specific data, with generative models that produce coherent natural language responses. This is particularly well-suited in financial services due to RAG’s ability to draw from trusted data sources such as company filings, regulatory documents, and market reports, allowing it to generate insights that are not only timely but also highly relevant.
Key use cases of RAG in finance include:
1. Automated Customer Support
- Personalized Queries: By retrieving customer-specific information, such as transaction histories or policy details, RAG provides tailored responses for complex financial products. Citibank, for example, uses RAG internally to assist representatives in real-time, ensuring precise and responsive customer interactions.
- FAQ Handling: RAG models retrieve answers to frequently asked questions about loans, credit cards, and insurance products from a broad knowledge base, significantly reducing response times.
- Customer Service in Action: When customers inquire about specific products like mortgage rates or loan terms, RAG retrieves and summarizes policy documents, FAQs, and market trends to support representatives.
Best Practice: Ensure responses exclude sensitive customer details by using anonymization layers and regularly updating RAG with changing policies.
2. Risk Analysis & Management
- Sentiment & Market Analysis: By aggregating data from financial news, social media, and analyst reports, RAG helps assess market sentiment for trading and investment strategies. Hedge funds like JPMorgan use this capability to improve their risk management frameworks.
- Credit Risk Assessment: RAG retrieves and analyzes borrower profiles using data from credit reports, transactions, and social platforms. This generates detailed risk profiles, helping lenders refine credit scoring models and reduce default risks.
- Asset Liability Management (ALM): Treasury departments leverage RAG to monitor interest rate trends, economic forecasts, and policy shifts. This enables banks to optimize asset and liability positions dynamically.
Best Practice: Use verified economic data sources and ensure frequent performance reviews to maintain accuracy.
3. Research & Portfolio Management
- Financial Insights & Recommendations: By accessing live market data, financial news, and regulatory updates, RAG provides actionable insights for portfolio adjustments and strategic planning. BlackRock is known to use RAG for timely and accurate decision-making.
- Earnings Summaries and Trend Analysis: RAG summarizes data from earnings calls, reports, and market trends, offering concise overviews that save time for analysts.
- Client Reporting and Portfolio Summarization: Wealth management firms utilize RAG to generate quarterly reports, highlighting portfolio performance, benchmarks, and rebalancing opportunities.
Best Practice: Train RAG on portfolio management principles and validate all outputs to ensure accuracy before delivering them to clients.
4. Regulatory Compliance & Audit Support
- Document Retrieval and Summarization: Financial institutions use RAG to quickly retrieve and summarize regulations like Basel III or MiFID II, enabling faster compliance reporting.
- Anti-Money Laundering (AML): RAG flags suspicious transaction patterns by cross-referencing data with known fraud indicators and compliance rules. Banks such as HSBC use this capability to streamline AML detection and KYC verification processes.
- Regulatory Compliance Monitoring: Investment firms use RAG to track global financial regulation changes that impact trading and reporting. Alerts notify analysts when critical updates occur.
Best Practice: Source only from verified regulatory bodies, and maintain logs of consulted documents for audit purposes.
5. Personalized Financial Advisory
- Investment Advisory: By retrieving real-time data on performance metrics and industry trends, RAG generates tailored recommendations. Advisors use these insights to help clients achieve their investment goals, such as focusing on renewable energy stocks.
- Budgeting & Financial Planning: RAG analyzes customer spending patterns to develop personalized savings or budgeting plans, making it a valuable tool for robo-advisors.
Best Practice: Set prompts to prioritize reliable and data-driven insights, avoiding speculative information that could mislead clients.
6. Data Retrieval for Decision Making
- Due Diligence for Mergers & Acquisitions: During M&A, RAG retrieves and summarizes financial reports and regulatory filings, enabling analysts to make informed recommendations.
- Real-Time Market Analysis: Traders leverage RAG to assess market conditions and stock performance in real-time, facilitating swift and informed trading decisions.
Best Practice: Limit RAG to reputable data sources and implement trader feedback loops to refine outputs for better accuracy.
The capabilities of RAG are being adopted by various financial institutions and banks are using RAG to power chatbots that can answer customer queries with real-time data, improving customer experiences and reducing the workload on customer service teams. For instance, Bank of America’s AI-powered virtual assistant, Erica, has surpassed 1.5 billion interactions since its launch in 2018, providing 24/7 customer support and efficiently handling queries and transactions, which leads to reduced waiting times and improved customer satisfaction.
The Risks of Using RAG in Financial Services
While RAG offers significant benefits, its introduction to financial services also comes with risks. According to a study by the Center for Audit Quality (CAQ), companies deploying generative AI, including RAG, in financial reporting face a number of audit risks. These range from governance failures to fraud and cybersecurity vulnerabilities.
1. Governance and Oversight
Financial institutions must establish clear governance frameworks to manage AI applications effectively. Without proper oversight, organizations may not fully understand where and how RAG is being deployed, leading to compliance issues. The “black box” nature of AI, where outputs are generated without clear traceability, makes it difficult for auditors to interpret or explain how decisions are made.
2. Regulatory Compliance
AI models can inadvertently violate regulations or legal contracts if not carefully managed. This is especially true in finance, where strict regulatory oversight governs operations. Organizations must ensure that their use of RAG complies with industry laws and avoid using AI in ways that might conflict with their obligations.
3. Skill Gaps
Financial professionals may lack the expertise required to implement and oversee RAG systems effectively. As generative AI becomes more prevalent, it is necessary for employees to be trained to ask accurate questions and interpret AI-generated outputs. Without this knowledge, employees may misuse RAG, which could then lead to inaccurate or irrelevant data.
4. Cybersecurity and Data Privacy
As with any AI technology, RAG is vulnerable to cyberattacks and data privacy breaches. Confidential financial data could be exposed if it is improperly entered into a RAG model, and hackers could exploit weaknesses in the system to manipulate outputs. Ensuring robust cybersecurity measures and controlling access to sensitive data is vital to mitigate these risks.
5. Fraud and Misuse
Generative AI could be misused to conceal fraudulent activities or generate misleading financial reports. Companies must be vigilant in monitoring RAG’s outputs and cross-checking them with independent data sources. Strong internal controls are necessary to prevent bad actors from exploiting AI systems.
Best Practices for Safely Using RAG in Finance
To safely use RAG in financial services, companies must develop a strategy that balances innovation with caution. Here are some key best practices:
1. Establish a Governance Model
Companies must have a governance model that provides visibility into how RAG is being used across the organization. Financial executives, including CFOs, should take an active role in linking AI governance to the broader AI strategy of the company. Regular audits and monitoring of AI outputs will help mitigate risks.
2. Focus on Employee Training
Building AI literacy among employees is crucial to ensure they understand the technology’s capabilities and limitations. Training should focus on how to craft effective prompts, interpret RAG outputs, and recognize potential errors. In finance, even small mistakes in data interpretation can have significant consequences, so proper training is essential.
3. Prioritize Cybersecurity
Implementing strict cybersecurity protocols is vital when using RAG in finance. This includes encrypting sensitive data, regularly testing AI models for vulnerabilities, and restricting access to the most sensitive information. Additionally, companies must monitor AI outputs for unusual patterns that could indicate a breach or manipulation.
4. Customize RAG for Specific Tasks
One of RAG’s key strengths is its ability to be fine-tuned for specific domains. Financial institutions should customize RAG models to their needs, whether analyzing ESG risks, pre-screening investments, or generating customer insights. Tailoring AI to fit the unique demands of finance ensures more accurate and contextually relevant outputs.
5. Regular Audits and Monitoring
Financial institutions must conduct regular audits of their RAG systems to ensure they are functioning as intended. Continuous monitoring of AI outputs will help identify any discrepancies or potential errors before they cause larger issues. Auditors should ensure that RAG’s outputs can be independently replicated to maintain trust in financial reporting.
What the Future Holds for RAG in Financial Services
The potential for RAG to revolutionize financial services goes beyond its current applications. As the industry continues to embrace this advanced technology, the future promises even greater integration, customization, and efficiency. RAG combines the capabilities of retrieval models that access real-time, industry-specific data with the language generation abilities of AI, creating solutions that cater to the rapidly evolving needs of finance.
One of the most significant future implications is the increased ability of RAG models to handle vast amounts of real-time data, providing decision-makers with highly accurate and timely insights. Financial markets, regulatory requirements, and global events change continuously, and RAG is uniquely positioned to stay up-to-date with these shifts, offering companies unparalleled flexibility. As data sources grow and improve, the accuracy of the predictions and insights generated by RAG will only get better. Financial institutions will be able to respond to market changes faster and more effectively, resulting in more proactive decision-making.
Another key area where RAG will have a significant impact is in regulatory compliance and risk management. The future of financial services will require companies to navigate increasingly complex regulations. RAG’s ability to retrieve and analyze up-to-the-minute regulatory documents will make it an indispensable tool for staying compliant, reducing the chances of oversight or human error. RAG can also help detect patterns in data to identify potential risks or fraudulent activities more accurately, making it an essential component of future risk management strategies.
Customization is another exciting prospect for RAG’s future. As AI technology becomes more advanced, RAG models will be fine-tuned for specific financial sectors or even individual firms, providing hyper-personalized solutions.
What Happens Next
Financial institutions that embrace RAG can gain a competitive edge by improving their decision-making processes and streamlining operations. From pre-screening investments to enhancing customer service, RAG has the potential to revolutionize finance. However, organizations must recognize the risks involved and take steps to ensure safe and effective use. By developing a solid governance framework, investing in employee training, and prioritizing cybersecurity, financial institutions can harness the full potential of RAG while minimizing its dangers.
In conclusion, while RAG offers exciting possibilities, its use in finance must be accompanied by comprehensive risk management strategies. As we consider the path forward, it’s clear that CFOs, auditors, and financial professionals will play a critical role in navigating this evolving AI landscape. Their guidance will be essential to ensuring that RAG’s transformative benefits are realized without compromising security, compliance, or accuracy.
Looking ahead, the true impact of AI in finance becomes even more compelling as we explore the critical issue of exaggerated AI claims. In our next installment, we’ll delve into the concept of AI washing, its effects on trust, regulation, and innovation, and provide practical strategies for identifying and addressing misleading practices. Stay tuned for a focused discussion on fostering genuine AI innovation in the financial sector.
If you plan on applying AI governance and risk management protocols, you can check out Lumenova’s RAI platform and book a product demo today. For organizations seeking an advisory starting point, our AI Risk Advisor provides tailored insights to help guide your AI policy decisions effectively.