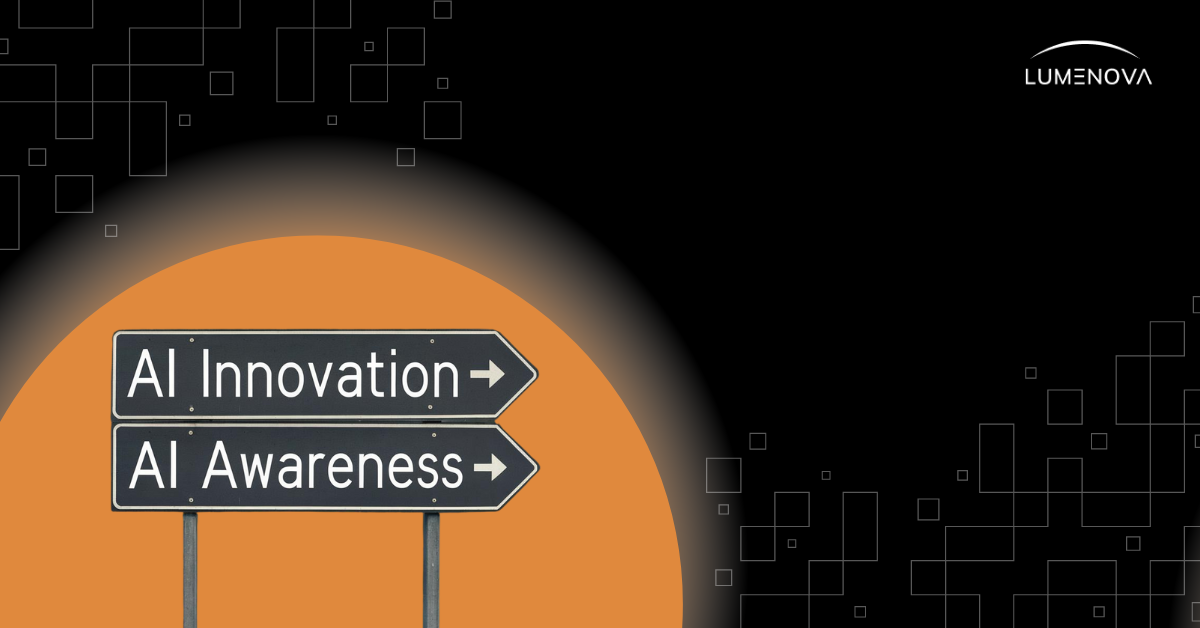
Ensuring a safe, trustworthy, and responsible AI-driven future depends on an array of complex and interconnected factors ranging from safety by design and the prevention of systemic and existential risks to the ability to balance AI’s transformative potential with its disruptiveness, and maintain the systems/infrastructures that uphold society, all in the face of exponential innovation. AI safety isn’t just a technical issue that AI developers, companies, and lawmakers must confront—it’s something that applies to every member of society, and the more stakeholders know about AI’s capabilities and limitations, risks and impacts, and possible use cases, the more meaningfully they can engage with the AI safety discourse.
Knowing what AI can and can’t do, understanding the magnitude and severity of its potential impacts, designing and implementing effective and resilient regulation, promoting and supporting scientific R&D, fostering inclusive economic growth and development—the ability to streamline and accomplish all of these objectives with the help of AI depends significantly on AI awareness at every level of society, from governments and companies to average citizens.
Regardless, the excitement around AI opportunities has inspired widespread global investment in AI design, development, and procurement, accelerating the pace of AI innovation and acquisition, which by itself isn’t a bad thing. However, when governments, businesses, and citizens inevitably begin to adopt this technology at progressively larger scales in the absence of corresponding scalable increases in AI awareness, the likelihood of AI-inspired adverse consequences increases dramatically.
Right now, we’re in a crucial period—even the most powerful AI systems are still relatively low risk when compared to their potential future counterparts, which are much closer than we think. To make this idea more concrete, consider the thought experiment below:
“Imagine supplying an entire remote village with simple bows and arrows, which none of its members have ever seen let alone used. You let a few days pass and return to find that several of the villagers have accidental self-inflicted injuries. Rather than sit down with them and explain how to use the bow and arrow safely and effectively, you decide to replace the bow and arrow with the “easier-to-use” crossbow. What do you think you’ll see the next time you visit the village?”
We’ve just conceptualized, rather grimly, a critical notion: AI, like a bow and arrow, is inherently risky and beneficial, but its risk-benefit ratio is influenced by whether those using it are aware of what constitutes safe and effective use. The replacement of the simple bow and arrow with the crossbow serves as an analogy to AI innovation. Just because the technology gets “better” doesn’t mean that it will be any safer—if we can’t safely and effectively use the rudimentary versions, we’re even less likely to use advanced versions as intended. The difference with AI, however, is that once the technology reaches a certain level of sophistication, it will be indistinguishable from its predecessors, meaning that those who aren’t AI-aware now will profoundly struggle to catch up with those who are.
Consequently, we’ll begin our discussion by examining the relationship between AI awareness and innovation in the US, adopting this US-centric perspective because of the US’ undisputed position as the global leader in AI innovation. Next, we’ll consider two hypothetical case studies, each of which will describe an AI integration initiative that fails due to a lack of AI awareness. Finally, we’ll conclude by providing a set of actionable recommendations for closing the AI innovation-awareness gap.
AI Awareness and Innovation: The Relationship
The rate at which AI innovates and proliferates, both nationally and internationally, makes it extremely difficult for most to keep up with the latest AI trends and developments. While over 90% of companies (globally) indicate an awareness of AI-driven risks and impacts, only 9% believe they’re prepared to address them, whereas 17% have taken measures to raise AI risk and impact awareness among their teams and key personnel. More broadly, 6 out of 10 leaders express concerns about their organization’s ability to integrate AI effectively whereas 55% worry that they won’t be able to source enough AI talent.
Moreover, while AI adoption rates have significantly increased in early 2024, Mckinsey’s State of AI 2024 report highlights that only 26% of C-level executives consistently use AI at work and throughout their daily lives, compared to 28% of senior managers and 24% of midlevel managers—throughout each of these categories, over 40% have little to no exposure to AI.
Nonetheless, AI adoption rates, while not strictly indicative of AI awareness levels, do provide us with tangible insights into AI awareness throughout the business landscape. If companies were better equipped to understand and mitigate AI risks and impacts while also identifying high-value use cases and opportunities, we would expect to see much higher adoption rates, especially among company leadership. In fact, two-thirds of leaders claim they wouldn’t hire someone without AI skills, yet only 25% of companies indicate plans to administer internal AI training initiatives—the disconnect between business leaders and the general workforce is painfully obvious.
Emerging concerns around a generative AI bubble also drive this point home, highlighting that many organizations and leaders have fallen prey to AI hype cycles over the last two years. Identifying where and how AI tools can deliver value in real-world environments, implementing sufficient safeguards and compliance protocols, being able to differentiate between various AI offerings or solutions by reference to their real-world utility value, ensuring that AI initiatives are scalable and aligned with key organizational objectives, building, maintaining, and improving workforce AI skills repertoires—many if not most of these factors have been overshadowed by AI’s “cool factor,” the erroneous popular belief that AI will just solve your problems for you, and the lack of a general standardized understanding of what this technology is, what it can do, and what it might be capable of.
Furthermore, despite intense excitement surrounding AI-driven opportunities, the majority of Americans don’t actually know much about AI. In November of 2023, the Pew Research Center revealed that while 90% of Americans know a little about AI, only a third claim to know a lot, and only 18% have hands-on experience with ChatGPT, which is one of, if not the most globally popularized AI tool. Similarly, a more recent 2024 study by YouGov reported that while 10% of Americans claim to know a lot about AI, 50% know very little or nothing at all, which, although more optimistic, remains deeply concerning.
Still, a separate 2024 study by the Common Sense Media and HopeLab highlighted even more pessimistic trends among young Americans (ages 14 to 22), revealing that over 40% have never leveraged AI tools whereas a mere 4% use them daily—historically, young people have been disproportionately more likely to adopt emerging technologies, so in theory, these statistics should be reversed. Moreover, the number one reason young Americans cited for not using generative AI (GenAI) tools concerned the perception that they’re unhelpful to them, which is perplexing since the top three GenAI use cases identified were research, brainstorming, and helping with school work, all of which are objectively useful.
In a nutshell, AI awareness levels are nowhere near where they should be in the US, which is concerning not only for the US, but also for many other countries that are taking AI innovation seriously—the US AI ecosystem houses some of the most prominent Tech Giants, investment, and venture capital firms in the world, which imbue it with a disproportionate amount of power, capital, and subsequently, international influence on AI. In other words, many current and emerging global AI trends can trace their roots back to the US AI ecosystem, or alternatively, to the attempt to compete with US-based AI initiatives.
To put this into perspective, let’s consider several factoids and statistics, the first bunch of which we’ll categorize as investment trends, and the second of which we’ll classify as innovation trends. To be clear, we’re not covering all AI investment and innovation trends, only those that are relevant to our discussion.
Investment Trends
- Of the AI startups included in Forbes 2024 AI top 50, 39 are US-based companies, 21 of which have obtained over $100 million in funding, and 4 of which have reached funding levels in the several billion-dollar range.
- According to the World Economic Forum, the US holds a strong global lead in private AI investments, with VC investments in AI eclipsing $290 billion over the last 5 years, which more than doubles Chinese (the runner-up) VC investments. In terms of total AI investment over the last 5 years, the US has spent over $328 billion, once more, followed by China at $132 billion, respectively.
- In 2023 alone, US-based AI investment surpassed $67 billion, which quadruples Chinese-based AI investments, and is approximately 20 times greater than AI investments made by runner-ups UK and India.
- The US holds such a commanding lead in AI investment that it would take China around 14 years to catch up, the UK around 79 years, and India over a century, provided they continue to invest in AI at the same rate they are now. These statistics should also be taken with a grain of salt in consideration of future AI breakthroughs made outside the US.
- According to the Forbes 2024 Midas list, 9 out of the 10 most prominent VCs in the world are US-based, with Sequoia (invested in OpenAI) and Ribbit Capital (invested in Coinbase) ranking 1 and 2, respectively.
- Mistral AI, one of Europe’s most promising and high-valuation AI startups, has been funded primarily by American VCs. Similarly, Aleph Alpha, Europe’s most prominent AI startup, has also received substantial US-based funding. Both of these company’s founders also solidified their careers at US tech companies, namely Meta, Google, and Apple, before leaving to create their own organizations.
- Multiple US Tech Giants, including Meta, Amazon, Microsoft, Apple, Netflix, Nvidia, and Tesla have numerous offices in the EU and UK, with some even extending their reach as far as South America, Asia, and Oceania. Simply put, there are few corners of the world that US tech companies have not touched.
- In 2023, US tech companies accounted for 36% of the global information technology and communication market share, more than tripling Europe and China’s individual shares, both of whom have the second largest shares, respectively.
Innovation Trends
- In June/July of this year, McKinsey (and Forbes) predicted that AI agents will emerge as the next frontier of generative AI (GenAI) technology. Even if this prediction doesn’t hold true, it suggests a potent demand for pragmatic AI tools that can handle complex open-ended tasks throughout dynamic real-world environments without severely compromising transparency and explainability standards.
- The pressure to democratize AI is growing as resource and development costs for Frontier AI models continue to increase in conjunction with smaller, more efficient, open-source AI offerings like Meta’s Llama 3.1, Mistral AI NeMo-Minitron, and the UAE Technology Innovation Institute’s Falcon Mamba 7B. Relatedly, OpenAI also allows paying users to utilize “mini” versions of their most powerful models (GPT 4o and o1-preview). Taken together, these trends indicate a focus on the ability to effectively run advanced AI models on edge devices with limited compute capacity (e.g., smartphones).
- Scaling AI initiatives has proved much more difficult than initially expected. Organizations are struggling to overcome problems like outdated legacy digital infrastructures, limited high-quality data, and GPU availability constraints. Practical concerns like employee skills gaps and a lack of general AI understanding at the leadership level only exacerbate these problems. These issues also emphasize the importance of developing smaller, more purpose-built, efficient, and easy-to-use/integrate AI tools.
- Europe’s leading AI startups—Mistral AI and Aleph Alpha—are developing state-of-the-art generative pre-trained transformer (GPT) models, attempting to directly compete with US-based AI companies like OpenAI, Anthropic, and Google, hinting that while AI innovation is concentrated in the US, influential breakthroughs are likely to occur on international scales. It’s also worth noting that Mistral AI and Aleph Alpha have yet to penetrate US markets significantly.
- As of 2023, China leads global GenAI patent acquisitions, outpacing the US, which holds second place, by a factor of six. In doing so, China has effectively cemented and reinforced its position in the global AI innovation landscape and demonstrated its status as a key player, specifically as the US’ number one contender.
- The University of Buffalo has just received $10 million in federal funding to create and establish a center for Early Literacy and RAI. Similarly, Yale University has pledged to privately fund an internal $150 million AI leadership initiative aimed at faculty, staff, and students. As for non-academic organizations, the National Science Foundation, Salesforce, and Google are investing millions in AI literacy and opportunity campaigns, with $8 million, $23 million, and $75 million, respectively—AI literacy is no longer just a concept, it’s becoming an imperative.
- According to a recent estimate by AI4SP, a whopping 92% of AI startups fail, primarily due to two reasons: 1. an inability to adequately and precisely capture what the market needs, and/or 2. operational challenges linked to factors like poorly designed KPIs, monetization strategies, and a lack of intellectual and skills diversity within key teams. This phenomenon stresses that even among AI founders, bridging the AI innovation and awareness gap—particularly in terms of what product end-users desire and seek to accomplish with AI in real-world settings—remains a major hurdle.
Key Takeaways
Based on our previous discussion and the investment and innovation trends we’ve illustrated, readers should consider the following key takeaways:
- While AI innovation and investment are concentrated in the US, AI breakthroughs will not be confined to the US AI Ecosystem. China and Europe are major players in the global AI landscape, and close attention should be paid to future international AI innovations. A US-centric understanding of AI innovation could be misleading.
- Near-term AI investments are likely to favor AI offerings with clearer value and utility propositions that are aligned with market, integration, and user needs, and scalable growth strategies. This suggests that startups will have to venture beyond the proof-of-concept stage to demonstrate real-world applicability and business value.
- As the GenAI hype continues to subside, early-stage AI companies and product developers won’t be able to simply label a product or service as GenAI-powered to garner investor interest. They’ll have to show why GenAI forms a crucial feature of the product, why it’s a better alternative to other AI and machine learning approaches, and how it will deliver positive tangible results in real-world settings.
- Purpose-built AI solutions, whether in the form of AI agents, custom models trained or fine-tuned on proprietary data, or smaller, more efficient, and easier-to-integrate systems, will likely stand out in the AI ecosystem. Leading AI companies and developers are great at building advanced AI, but they’re not as good at understanding where it could be useful.
- There is still a major disconnect between leaders and their workforce regarding what’s required to pursue AI integration initiatives in responsible, effective, and scalable ways. In the coming years, we can expect a much stronger emphasis on AI literacy development throughout all factions of society, from governments and corporations to higher education and even local communities.
- AI companies will be forced to confront the fundamentally multi-disciplinary nature of AI, especially in light of mounting regulatory pressures, ethical and risk-related concerns, and the ability to discover niches in an increasingly saturated market. The future of AI doesn’t just include computer and data scientists—we need people who understand economics, psychology, philosophy, education, politics, and culture.
Case Studies
To move from the abstract to the concrete, this section illustrates two hypothetical case studies, each of which outlines a failed AI integration initiative that occurs due to an AI awareness-innovation gap. The first of these case studies examines how misaligned communication on AI initiatives between the leader of a mortgage company and their key personnel leads to an AI integration failure. By contrast, the second case demonstrates why it is crucial to continually maintain AI literacy in an academic institution where both educators and students regularly use AI.
Case Study 1: Mortgage Company AI Failure
SmartLenders, a medium-sized mortgage firm, has begun losing several of its long-time customers to other local firms that offer a more friendly, innovative, and pragmatic customer experience. In an attempt to quickly pivot and remain competitive, SmartLenders’ CEO has decided to integrate an AI-powered underwriting system to improve and streamline loan processing claims and augment risk assessment practices. The new system is designed to automate roughly 70% of the decision-making process to ultimately reduce loan approval times by almost 50% while allowing the company to ingest and process higher volumes of applications without hiring new personnel.
Despite the CEO’s excitement around this opportunity, the system’s potential, purpose, and the necessary skills required to operate effectively aren’t comprehensively communicated to key teams. Loan officers and underwriters, who form the foundation of the company’s value and services, receive only a vague memo loosely outlining the project and emphasizing the cost-reduction and efficiency benefits it will inspire—this memo also fails to mention that loan officers and underwriters are critical to the system’s performance in terms of providing feedback that enables it to improve its predictive accuracy over time. Consequently, employees perceive the system as a threat to their livelihood, fearing job loss and irrelevance that results in widespread apprehension. Had key personnel been thoroughly involved in the early stages of this AI integration initiative, receiving detailed information on the collaborative role and purpose the system will play and the skills required to enact its benefits, adoption would have proceeded more swiftly.
Nonetheless, the vast majority of the company’s loan officers and underwriters continue to rely on manual methods, doubting the system’s ability to perform reliably, especially in complex cases where human judgment is necessary. Still, a few loan officers and underwriters do hesitantly embrace the system, only to find that its performance is inconsistent, frequently inaccurate, and in some complex cases, entirely incomprehensible—recall that the system’s ability to improve performance over time depends on feedback from core personnel.
On the customer-facing side, sales teams are profoundly struggling to articulate to customers the benefits of the company’s AI integration initiative. Not only is the sales team unable to regularly showcase real-world positive results generated by the system—due to loan officers' and underwriters’ reluctance to use it—they’re also forced to address customer concerns regarding the role the system plays in decision-making and the handling of sensitive personal financial information, particularly for customers with irregular financial profiles. This was an obvious risk that the sales team should have been made aware of from the very beginning, and now, it’s also inspiring concerns among the company’s in-house legal and compliance team.
About 6 months in, SmartLenders’ CEO can no longer justify the million-dollar investment in the system—loan approval times are deeply inconsistent, employees are frustrated with unclear performance metrics and work-related duties, and customer satisfaction has fallen dramatically. To save face and minimize further reputational damages and potential compliance costs, SmartLends’ CEO issues a press release, detailing that the AI integration failure was a result of “technology limitations” when in reality, it occurred because of the CEO’s failure to holistically communicate all relevant information on the initiative.
Case Study 2: AI Literacy in an Academic Institution
Steeple University, an academic institution well-known for its innovative and forward-thinking approach to new technologies, implements a university-wide AI initiative for students and faculty who are granted access to a variety of state-of-the-art AI tools. The university actively encourages faculty to leverage AI for a range of tasks including administrative functions, automated grading, plagiarism detection, and curriculum development. Students, on the other hand, are allowed to use AI-powered platforms for several academic tasks like writing and editing assistance, research and data analysis, creative ideation and project development/planning, and exam preparation.
During the first year of the initiative, the university heavily invests in AI training for students and faculty, ensuring that they all possess the skills required to leverage AI tools effectively. Early benefits are widespread, with students reporting major improvements in personalized feedback and data-driven performance insights alongside increases in personal productivity and creativity with professors maintaining far quicker turnaround times for grading, administrative, and curriculum development tasks.
However, approximately one year later, everything changes. Major advancements in the AI landscape have redefined the utility, value, and complexity of many of the tools professors and students use. These newer tools are far more sophisticated than their predecessors, enabling advanced functions like real-time content and experimental results generation, reasoning about complex/open-ended tasks and problems, automated research paper drafting, and even the ability to manipulate plagiarism detection systems. Being digital natives, students are quick to adopt these newer tools and capitalize on their capabilities repertoires, but professors, being far less accustomed to these technologies, are overwhelmed by rapid innovation and struggle to provide meaningful guidance on ethical AI usage and critical thinking regarding AI outputs.
Operating under the belief that initial AI training was sufficient, especially due to the notable successes that emerged during the first year of the initiative, Steeple University failed to invest in further AI training for its faculty—AI workshops, skills, and awareness assessments are no longer regularly administered, and faculty development budgets are diverted to other priorities like research grants.
As the AI literacy gap between professors and students grows, students begin relying more heavily on AI to complete their assignments, elevating concerns about academic integrity, independent thinking, authenticity, and intellectual development. Meanwhile, professors realize they can no longer reliably distinguish between original and AI-generated work, leading to inconsistent grading practices, false plagiarism claims, and serious ambiguities around how much AI-powered assistance is appropriate. Moreover, academic integrity policies, particularly the university’s honor code, have utterly failed to capture the nuances around AI-assisted learning and academic work, opening up a grey area that many students exploit without having to face any consequences.
A few more years down the line, Steeple University’s upstanding reputation is challenged when employers begin expressing concerns that graduates, although they appear qualified “on paper,” are actually sub-par critical thinkers and problem solvers. By contrast, two-thirds of the university’s professors draft an open letter to the Steeple’s president, outlining the issue of faculty burnout—a direct consequence of having to maintain high AI literacy and teaching standards simultaneously. Being too heavily invested in this initiative, Steeple University has no choice but to invest even more in retraining its faculty and updating its academic integrity policy, realizing that had they implemented regular upskilling practices and maintained a closer eye on what students were using AI for and how, this problem could have been easily avoided.
Recommendations and Conclusion
While there is hope that the AI innovation-awareness gap will be closed, we leave readers with the following recommendations, intended primarily for organizational leaders who must frequently maintain a big-picture perspective. These recommendations are industry-agnostic to allow for flexibility, adaptability, and alignment with specific preferences and objectives that leaders throughout different sectors might have.
- Design, implement, and maintain AI literacy initiatives. These initiatives should not be generic and target specific AI tools, work-related task domains, key teams and personnel, and the skills required to operate AI models effectively and responsibly. In certain cases, organization-wide AI literacy initiatives that highlight the latest advancements/trends in the AI ecosystem alongside relevant safety, ethics, and compliance concerns should also be administered.
- Build an in-house AI team whose roles include AI integration, training and upskilling, governance and risk management, market research and analysis, and outreach with industry AI providers. Your in-house AI team should be multi-disciplinary, drawing experts from a variety of fields from economics to philosophy.
- Look beyond the US AI ecosystem to understand what’s happening on the global stage. The US might be dominating the AI race, but this doesn’t mean that other countries won’t make highly consequential discoveries and innovations that will eventually find their way into US markets.
- Scrutinize different AI offerings to understand which one is best for you. If an AI solution is only moderately well-aligned with your key values and objectives, chances are something better exists. Resist the urge to settle for the first solution that comes your way, and don’t hesitate to seek external expertise if needed.
- Be prepared to fail and pivot if necessary. With any exponential technology, particularly AI, the ability to quickly respond to and overcome potential failures is paramount—reputational and compliance damages can cripple businesses, especially during their earlier development and growth stages. Having an actionable plan that enables your organization to maintain operations in the event of catastrophic AI failures will set you apart from your competitors.
- AI-induced organizational transformation will not be a smooth, fast, or secure process. Consider what you want to achieve with AI integration, how you will measure the success of your AI initiatives, how you will address relevant risks and hurdles, and how you might have to unroot and redefine many of the fundamental assumptions you have about your organization’s structure, function, and value.
- Create internal environments in which teams can safely experiment with different AI tools to find the solutions that are best suited to their preferences and tasks while seamlessly integrating with existing workflows. These experimental hubs will also allow teams to pinpoint risks and limitations in the tools they use, and then transmit this information to those responsible for governance and risk management practices to ensure comprehensive and robust coverage.
- Periodically evaluate AI awareness levels throughout relevant factions of your organization to determine where AI integration initiatives are successful/unsuccessful, refine and update KPIs, address emerging risks and opportunities, and facilitate continual upskilling. These evaluations should not only consider AI literacy—the skills required to leverage AI effectively and responsibly—but also whether AI integration remains aligned with business objectives and values, knowledge of industry/domain-specific risks and opportunities, and awareness of the latest state-of-the-art tools available.
These recommendations don’t represent a surefire solution to closing the AI innovation-awareness gap—each organization that confronts this problem will have to create and implement its own remedy, and this will require some ingenuity. That being said, our recommendations do provide readers with a solid starting point, notably one that they can expand upon, refine, and modify according to their overall needs.
For readers interested in exploring other AI-related topics across fields like GenAI, risk management, and governance, we suggest following Lumenova AI’s blog, where you can easily track progress through these domains and maintain an up-to-date perspective on the AI innovation ecosystem.
For those who have already begun designing, developing, or integrating AI risk management and/or governance frameworks, protocols, or standards, we invite you to check out Lumenova’s RAI platform and book a product demo today.