November 7, 2024
Managing AI Risks Responsibly: Why the Key is AI Literacy
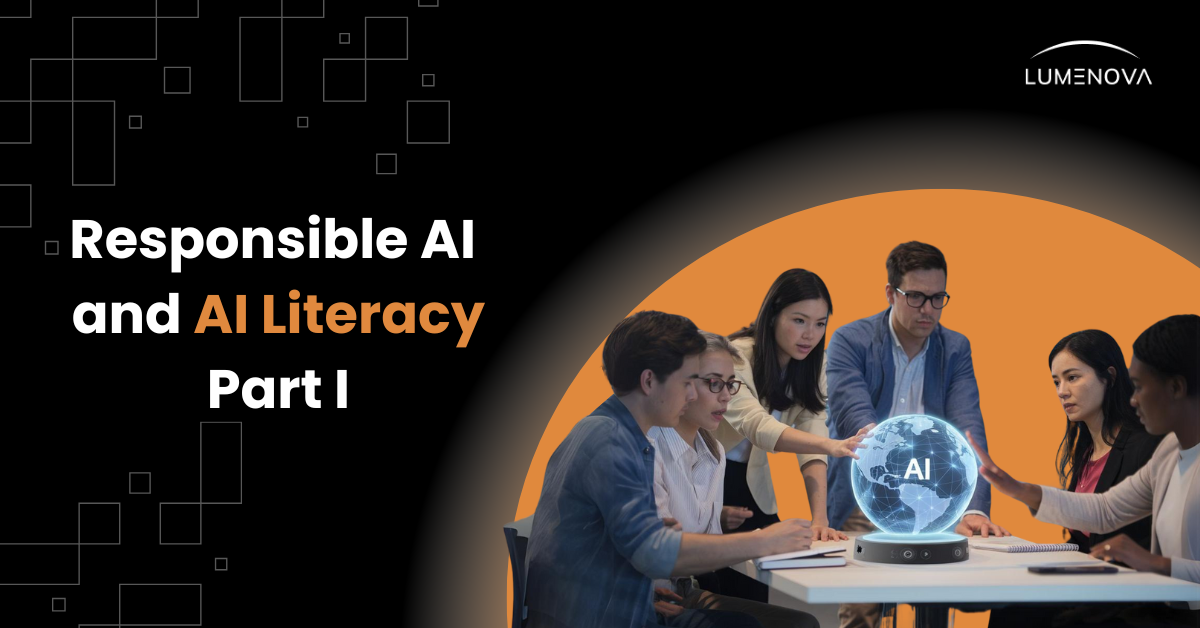
Contents
AI literacy is becoming a central—though still undervalued and underrepresented—tenet of responsible AI (RAI). It’s a practical mechanism through which AI users are empowered with more accountability, control, and understanding of AI products and services while continually increasing their awareness of how AI capabilities, risks, impacts, and skills may evolve over time.
Consequently, it’s unsurprising that the world’s first comprehensive AI legislation—the EU AI Act—directly endorses AI literacy as one of its core objectives. Notable organizations in the private and academic sectors are also following suit, with some tech companies, including IBM, Google, and Amazon, alongside leading universities like Yale, Stanford, and Berkeley, funneling significant resources into building and offering pragmatic and proactive AI skills procurement initiatives for professionals of all profiles.
Moreover, while the US federal government has made inconsequential progress on national AI literacy campaigns, hope should not be abandoned, in large part due to organizations like the National AI Advisory Committee (NAIAC), the Bipartisan Policy Center, and the National Institute for Science and Technology (NIST), all of which continue to contribute to and stress the growing importance of AI skills and knowledge procurement, particularly at a nation-wide scale. With hundreds of additional pieces of AI legislation under development, we further expect the emergence of early-stage regulations aimed at increasing the resources, channels, and knowledge necessary for administering large-scale AI awareness and education campaigns over the next year—these regulations will likely begin with states and then hopefully inform the US’ national AI literacy strategy, even if it isn’t federally enforced.
In the research community, AI literacy is gaining traction, though not as fast as we’d imagine, and we hypothesize this may be due to a lack of available evidence on which to base robust empirical studies. Although numerous papers on the topic have been published, the majority have approached it from a narrow (usually education-centric) or theoretical point of view, while those with the highest number of citations (a quick search on an academic database will confirm this) were published during or before 2022—this is a critical consideration seeing as November 2022 marks the public release of ChatGPT, which revolutionized how both the technical and non-technical individual leverages AI globally.
This final point brings us to the first part of our discussion, in which we’ll examine the macro and micro-level reasons for why AI literacy could form the foundation of RAI use—in other words, why individual and collective AI literacy matters. Next, we’ll explore, at a high level, how comprehensive AI literacy within an organization can reduce the AI risk management and governance burden it faces while simultaneously positioning the whole as more adaptive, flexible, and inherently transformative—the more you know about AI, the more effectively you can manage the dangers it poses. We’ll conclude with some brief recommendations, leaving readers with actionable guidance and setting the stage for part II of this series.
Understanding AI Literacy: A Foundation for Responsible AI Use
A standardized definition of AI literacy has yet to be constructed, and that’s okay. When considering the expansive variety of available AI tools and evolving/emerging use cases, even if we were to confine them to one area like generative AI (GenAI), it’s clear that a narrow and targeted definition isn’t very useful to the average AI user.
Interestingly, however, as AI users become more specialized and advanced, we expect they will seek to understand, from a granular and forward-looking perspective, what constitutes AI literacy within their domain of interest—the process of maintaining a sufficient degree of AI knowledge and awareness is ongoing and dynamic, reflecting the continually shifting forefront of AI innovation, deployment, and integration.
Therefore, the definition we illustrate below represents our attempt to find the balance between the demand for standardization and collective accessibility vs. the ability to adjust for domain, value, objective, or tool-centric preferences on behalf of individual users. The notion of AI literacy must be scalable and differentiable, and there’s no reason why this should come at the cost of individualized fine-tuning and targeted application.
“Definition: AI literacy is the perpetual process of developing, maintaining, and improving AI-specific skills in a contextually appropriate manner, awareness of AI tools, capabilities, limitations, and use cases, and understanding of the evolution, context, and manifestation of pertinent AI risks, benefits, and real-world impacts.”
Readers should consider a few key takeaways from this definition. First, AI literacy exists on a spectrum with no endpoint—you can be more or less literate, but your literacy journey will never be “complete.” Second, AI literacy requires you to approach AI with a multi-faceted perspective—someone who is an “expert” AI user but knows nothing about the dangers relevant to their AI use isn’t AI literate. Third, AI literacy doesn’t require in-depth technical AI knowledge—it’s a pragmatic mechanism to conceptualize and evaluate the skills and knowledge required to operate various kinds of AI effectively and responsibly. Fourth, AI literacy doesn’t need to look the same for all AI users—some users may choose to predominantly leverage text-to-image generators while others may prefer to spend more time working with and developing AI agents.
Finally, AI literacy won’t remain constant, even broadly speaking. For instance, if some fundamental change to the way most humans interact with AI were to occur—if we stopped using language and used only our thoughts—its ramifications for the larger AI space would force us to update the foundation on which we’ve built our AI skills and knowledge base.
Now that we’ve defined AI literacy, we can break down its constituent roles at the individual and collective scale, viewing them through an RAI lens. We’ll begin with the individual perspective first:
-
AI literacy cultivates a professional competitive advantage → AI literate professionals will outperform their less AI-skilled coworkers, and this could be the catalyst that drives others to begin their AI upskilling and awareness journey without waiting for a handout. Individual competitive incentives indirectly favor collective AI literacy.
-
AI literate users are more likely to leverage AI responsibly → If AI users possess even a moderately sophisticated understanding of an AI tool’s risk-benefit profile, they’ll be less likely to use it in ways that perpetuate harm due to their ability to identify potential biases, privacy concerns, security risks, and other AI risks. Admittedly, this is predicated on the assumption that the majority of AI users are reasonable people with decent intentions—a phenomenon that seems to ring true for most technologies humanity has invented.
-
AI literate users are more capable of spotting malicious AI use → Bad actors remain a significant concern within the AI safety discourse, mainly because of how difficult it is to identify them, anticipate their intentions, and counteract them before harm occurs. In organizational settings, AI literate users will play a valuable part in spotting and reporting malicious AI use. Where bad actors represent a source of external risk, these users will play an indirect but equally important part, helping their larger organizations address AI vulnerabilities before they can exploited.
-
AI literate users can more easily build trust and confidence in AI → Knowing what AI can do is just as crucial as knowing what it can’t do. AI literate users are aware of the capabilities and limitations of the specific AI tools they leverage, and this will enable them to identify for which tasks or objectives reliance on AI is appropriate vs. inappropriate. More advanced and prolific users may also develop an intuition for discerning when a model displays emergent or hidden capabilities and limitations.
-
AI literate users are more adaptive to AI-induced transformations → An AI literate user has, by definition, embraced AI, but not blindly. Such users will resist AI-driven disruptions while guiding and supporting transformations, operationalizing the skills and knowledge required to enact positive AI impacts. AI literate users are also more capable of identifying potential skills and knowledge gaps within transformation strategies, improving their overall robustness and resilience, and cementing their position as high-value assets to an organization.
-
AI literate users will help their less literate team members → While this appears to contradict our first point, we’re actually looking at the other side of the coin here. Where professional teams are composed of AI literate and non-literate members, those who are literate are directly incentivized to help the rest of their team upskill, not for charitable reasons, but for self-interested ones—a team’s performance is evaluated as a whole, meaning that every one of its members has a stake in its overall success.
-
AI literacy empowers users with more control over their professional future → AI will create many new sources of value while modifying and eliminating others. AI literate professionals, due to their continual learning mindset, will be engaged in a constant upskilling and reskilling process whereby they ensure the continued relevance of their skills as workforce dynamics fluctuate in response to AI innovations, disruptions, and transformations.
From a collective perspective, AI literacy can:
-
Allow organizations to build a resilient AI-enabled workforce → By implementing organization-wide AI education and awareness programs, organizations can increase the flexibility and adaptability of their workforce when responding to or initiating AI-driven changes. By administering these programs, organizations will also demonstrate that they value their employees, reducing the potency of automation-induced fears while increasing the loyalty and alignment of their workforce with overarching organizational objectives and mission.
-
Encourage human-centric AI innovation → AI should augment and empower humans to become better versions of themselves through self-determination and access to a greater variety of resources and opportunities—regular citizens should have a voice in the dialogue on what AI is designed and built to accomplish. The higher collective AI literacy rates are, the more capable citizens will be of putting pressure on policymakers to support laws that reflect society’s larger interests and values while pushing AI companies to build products and services that directly meet user needs rather than challenge them. Collective AI literacy can drive the democratization of AI.
-
Encourage RAI experimentation → Experimentation forms a major dimension of RAI development—for AI products and services to align with safety, ethics, and governance best practices, vulnerabilities and potential avenues for malicious exploitation must be addressed. AI must be stress-tested in the real world, and AI literate populations could be instrumental in this case, experimenting with and applying AI in diverse and unexpected ways, showcasing vulnerabilities while reducing the likelihood of misuse. Technical safety experts are obviously still needed, but we must recognize that no matter how much technical AI safety expertise a small group has, it will never be able to account for all the possible ways AI might be used irresponsibly or dangerously.
-
Lay the groundwork for standardized concepts and definitions in AI safety and ethics → While there may be some high-level agreement on what constitutes “good” vs. “bad” AI use, detailed and universally accepted RAI principles and mechanisms have yet to emerge. With technology as powerful as AI, there must be at least some high-level alignment between the world’s most influential powers on what constitutes safe and ethical use and deployment. AI literate users will be a prevalent force in this process, particularly as they aggregate at larger scales, uncovering both positive and negative insights on AI use and adoption trends that transcend cultural and societal boundaries.
-
Foster AI knowledge sharing and collaboration → An AI literate user who is forced to compete individually with non-literate peers won’t relinquish their competitive advantage. However, if everyone within the group is literate to some degree, recognizing who has the competitive advantage will be more difficult. Consequently, the group’s time is better spent on sharing AI knowledge, precisely because the individual cost-benefit ratio of knowledge collaboration outweighs that of not participating at all—it’s also worth mentioning that no one in the group actually knows how others will choose to apply their AI knowledge, meaning that competitive advantages are still up for grabs (at least they are perceived as such, which is more than enough).
-
Reduce the probability of AI-orchestrated mass manipulation or coercion → AI literate individuals are critical pragmatists, grounding their understanding of AI in its real-world value and utility. At the group level, this enables communities to develop a collective understanding of how AI might be leveraged to influence opinions or behaviors en masse, improving the group’s larger ability to identify and guard against manipulation attempts, particularly those orchestrated via social media platforms or within political contexts.
Overall, building AI knowledge, skills, and awareness should constitute a cornerstone of the RAI foundation, and while we could say much more about the individual and collective benefits this process inspires, the general overview we’ve provided suffices in demonstrating the immense positive impacts of AI literacy, particularly in terms of AI accessibility, safety, and innovation.
AI Literacy in Action: Reducing the AI Risk Management and Governance Burden
To create some context, we’ll begin by highlighting an array of key factors, framed as challenges, that fall at the core of the AI risk management and governance burden an organization faces. Next, we’ll explain how AI literacy can reduce the potency of these factors and subsequently lower this very burden.
In the interest of transparency, we’d also like to note that the factors discussed here don’t holistically represent all the key challenges organizations can expect to encounter within the aforementioned domains, only the ones that can be ameliorated through AI skills procurement and knowledge acquisition—for readers interested in taking a deep dive into these fields, we suggest checking out some of our in-depth AI governance and risk management content.
For clarity, we’ll subdivide the factors we examine into two groups, beginning with AI risk management and then governance:
-
Risk Identification and Classification: AI risks can manifest in numerous disparate forms and timescales at various stages of the AI lifecycle, from bias, data privacy, hallucination, cybersecurity, and adversarial risks to overreliance, decision-making opacity, goal drift, algorithmic entrenchment, and proxy alignment failure. Understanding which risks to prioritize and classify, particularly during and after deployment, can be extremely challenging, especially with frontier AI models, which may exhibit emergent properties as they scale.
-
Risk Evolution: Even when risks are identified and classified, they may not remain constant over time as AI tools and applications undergo modifications, updates, and/or improvements. Similarly, while AI systems may perform reliably within certain environments or settings, changes to the environment in which they operate could drastically alter their efficacy and safety—building robust and resilient AI systems continues to represent a major hurdle within the AI development landscape. For advanced models that leverage unsupervised and/or self-supervised learning paradigms, implementing risk controls that account for the dynamic evolution of their capabilities and limitations is also highly difficult, even when models are pre-released to beta-testers before official deployment.
-
Operational and Cybersecurity Risks: Certain risks may not surface until an AI system is deployed within a real-world environment. For instance, an organization might fail to account for the systemic effects of overreliance on AI, which accrue slowly yet steadily, until irreversible damage is caused. From the cybersecurity perspective, adversarial testing during pre-deployment stages can only go so far—even the most brilliant team of adversarial testers can’t be expected to account for all the possible cybersecurity vulnerabilities an AI system inspires when integrated.
-
Standardized Risk Assessment Metrics: While frameworks like the NIST AI RMF and ISO 42001 represent promising steps toward a standardized model of AI risk assessment, they are still in their infancy, and most organizations have yet to implement them let alone be aware of their existence. On the other hand, the diverse breadth of available AI tools and applications continues to expand exponentially, covering more and more use cases with each day that passes—this suggests that purpose-built standardized risk assessment metrics will need to be created for specific kinds of AI tools and use contexts, which is a monumental task on its own.
-
Oversight and Accountability: In an ideal world, the team or individual who oversees the use of an AI system should be held accountable when things go wrong. But, what happens when hundreds or thousands of users leverage that system during day-to-day operations for multiple tasks and objectives? Mass-work surveillance is clearly not a viable option, suggesting that complex frameworks for distributed accountability and oversight are the path forward. Moreover, further complexities will emerge with models that lack transparency and explainability, particularly in scenarios where external audits must be conducted or where such models play a primary role in driving consequential decision-making.
-
Balancing Risk: To innovate, you need to take risks. However, you should understand which risks are worth taking vs. which risks are so severe that they can never be entertained. For organizations to initiate and maintain successful AI integration and deployment practices, they need to adopt a risk-neutral perspective that considers all possible known risks from a measured and informed viewpoint that is constantly updated to reflect AI advancements and real-world impacts. As compliance pressures strengthen, organizations will also be forced to account for an increasing variety of socio-technical risks, which, though they may not be as “severe” as AI-induced operational or cybersecurity failures, will warrant an equivalent degree of consideration.
-
Sensitive Data Handling: Once an organization integrates AI, ensuring that various users don’t intentionally or accidentally input sensitive data—for example, proprietary product descriptions or confidential customer information—is much harder than it would seem. Data governance and management policies are moderately effective mechanisms for reducing the possibility of poor sensitive data handling, but with larger organizations, we run into the same problem we encounter with oversight and accountability—how can an organization generate unobstructed yet ethical visibility into how its workforce leverages AI?
AI Governance challenges include:
-
Adaptive, Continuous Monitoring: Establishing reliable and adaptable mechanisms for continuous AI performance, risk, and impact monitoring is a challenging process, especially for organizations that leverage different kinds of AI across departments or domains. Building and implementing these mechanisms also requires that organizations draw from an array of internal and/or external expertise, ensuring that their mechanisms align with technical gold standards while respecting compliance and business requirements, adapting to changes in workflow dynamics and operations, and capturing all relevant KPIs, risks, and impacts AI produces. From end to end, this process can become extremely costly, resource-intensive, and complicated.
-
Transparency and Explainability Limitations: While the field of explainable AI (XAI) is committed to pioneering effective solutions—for instance, LIME and SHAP—for overcoming advanced AI’s transparency and explainability limitations, much more work is needed before we can say that we’ve solved this fundamental problem. Though not all AI systems possess these limitations, most GenAI tools and applications do, and seeing as GenAI still largely represents the AI development, deployment, and adoption frontier, XAI solutions must be discovered and implemented before the technology scales to a point where its impacts become almost impossible to reverse. XAI solutions should also entertain the increasingly wide variety of AI technologies, operationalized throughout diverse use contexts and potentially inconsistent environments.
-
Understanding Accountability: We already suggested that distributed accountability frameworks could be the path toward understanding and maintaining AI accountability within organizational settings. These frameworks will be complex, dynamic, and context-specific, built to meet an organization’s AI needs and objectives as they evolve. Moreover, the current absence of standardized distributed accountability frameworks indicates that organizations will have to pursue and implement these strategies from the ground up, having to make assumptions about what they think might work, without having any valid excuses to fall back on when things don’t go as planned.
-
Regulatory Uncertainty: Within a regulatory landscape as fragmented as that of the US, ensuring that AI governance approaches tightly reflect the latest regulatory developments, particularly on a state-by-state basis and with no distinct plans for federal regulation in sight, is a daunting task. This means that most organizations will have to self-regulate for now making educated guesses as to what kinds of compliance and business requirements they’ll face within their respective industries and jurisdictions. To circumvent this problem, some organizations may choose to “wait and see”, which is an equally if not more dangerous strategy that could leave an organization entirely unprepared for when AI regulations enter into full force.
-
Cross-Functional Collaboration and Coordination: For AI governance to be effective, it must operate at every level of an organization, fostering cross-functional collaboration and coordination between diverse sets of stakeholders. This holistic approach should drive organization-wide awareness of AI risks, impacts, and benefits, meaning that organizations will need to equip all their members with the knowledge, tools, and skills necessary to transparently understand and enact AI governance provisions. To make things even more complicated, AI governance strategies must be inherently flexible and adaptable, internalizing advancements within the AI innovation and regulation ecosystems while remaining organizationally relevant.
-
Resource and Expertise Constraints: Both AI governance and risk management are resource and expertise-intensive tasks, which, if they are to be pursued successfully, can’t be confined to one domain, whether legal or technical. Comprehending the evolving conglomeration of governance and risk management requirements necessitates multi-disciplinary engagement with internal and/or external actors including business teams and end users, skills procurement specialists, managers and executives, legal, HR, cybersecurity, and AI experts, and risk and impact forecasters. There are several other actors to include, but the main point is this: if organizations don’t carefully examine the full scope of their AI governance and risk management strategies, they risk potentially depleting available resources and expertise before a viable strategy is developed.
Now, we’ll look at all these AI risk management and governance challenges together, explaining how in each case we’ve identified, AI literacy can lower the weight of the burden they inspire.
-
Risk Identification and Classification: As stakeholders become more AI literate, their corresponding awareness and knowledge of AI risks increases, enabling them to identify, classify, and contextualize a wider range of AI risks more effectively at multiple levels of an organization. This could dramatically streamline the risk identification and classification process, and significantly reduce the amount of resources and expertise organizations must expend during pre-deployment, early integration, or post-modification stages of risk management.
-
Risk Evolution: AI literacy encourages and supports a foundational understanding of the dynamic nature of AI systems and the environments in which they operate, serving as a mechanism to equip teams with the ability to anticipate and counteract potential shifts in model behaviors with adaptive risk controls. Organizations can also broaden their safety net by educating end-users and beta-testers, promoting early-stage risk detection when models are updated or modified.
-
Operational and Cybersecurity Risks: Due to their understanding of where human and AI capabilities intersect and a heightened ability to spot potential vulnerabilities in AI models (e.g., jailbreak susceptibility), AI literate teams will be better able to grasp and envision AI-induced workflow changes that could introduce operational and cybersecurity risks. Such teams will also be more likely to communicate their concerns to upper management and the executive suite, realizing that failure to do so could result in irreparable organizational damages that inspire dire negative externalities for them and the larger organization.
-
Standardized Risk Assessment Metrics: AI literate users will be aware of risk management standards and frameworks like ISO 42001 and the NIST AI RMF while recognizing the ongoing importance of robust risk assessment tools. Within an organization, such users are incentivized to help inform the development of standardized risk assessment metrics, realizing that if AI risks aren’t comprehensively and consistently addressed within their respective industries or sectors, systemic risk scenarios could unfold, jeopardizing their organization’s ability to innovate, and consequently, their continued value and utility as workers within a rapidly changing work landscape.
-
Oversight and Accountability: When those in leadership positions fully comprehend the limitations of the AI tools and applications used within their teams, assigning responsibility to relevant factions of their team in contextually appropriate ways will come more easily and transparently. This approach is also less likely to overburden individual employees with an overwhelming array of responsibilities, implicitly encouraging a culture of shared oversight where employees look out for each other in line with their unified responsibility for maintaining safe and fair AI use.
-
Balancing Risk: If full-scale AI literacy is achieved, organizations can leverage insights and recommendations readily provided by their workforce to drive their innovation and transformation strategies. By paying close attention to how employees use AI, identifying the challenges and solutions they encounter, and maintaining steady communication, reporting, and feedback channels, organizations will empower their workforce to play a primary role in balancing AI risks and benefits. Additionally, when employees feel that their organizations care about their professional well-being, as evidenced by AI education campaigns, they will develop a vested interest in the organization’s continued success, hence their willingness to drive and support innovation rather than resist it.
-
Sensitive Data Handling: While data governance is a separate field that organizations should prioritize to enact AI benefits and prevent risks, AI literate stakeholders will nonetheless be generally aware of the consequences of poor data handling and the importance of adhering to data management policies when interacting with AI systems. Ethical and cautious data practices are at the forefront of the RAI discourse, and AI literacy, when positioned as an RAI mechanism, will help organizations counteract potential data misuse cases and breaches cross-functionally and proactively, especially in cases where third-party providers supply AI services.
-
Adaptive, Continuous Monitoring: For continuous monitoring to work as intended, stakeholders should immediately report risks when they emerge, even if they’re unsure whether something qualifies as a risk. In this respect, AI literacy instills a healthy skepticism of AI, rooted in a pragmatic understanding of the technology that pushes users to make sense of it at face value, in terms of what it can and can’t do—it also enables stakeholders to accurately interpret, track, and achieve continuous monitoring objectives. More broadly, AI literacy supports sustained and adaptive AI risk tracking, allowing an organization to rely on an engaged workforce to help monitor and adjust AI practices as necessary.
-
Transparency and Explainability Limitations: Transparency and explainability limitations will be among the first notions an AI literate workforce deeply understands. While users can’t be expected to deploy and implement XAI methods to resolve these limitations—this should be reserved for technical XAI specialists—they will serve a vital function in critically assessing models’ transparency and explainability within real-world professional environments. XAI solutions must be continually informed by end-users, otherwise, organizations risk expending significant costs on solutions developed in a vacuum that fail to capture how AI is leveraged in the real world.
-
Understanding Accountability: Distributed accountability frameworks can get convoluted quickly, introducing avoidable complexities into an already complicated RAI domain. AI literate teams, however, will be more adept at accurately and precisely partitioning AI governance roles throughout their member base, increasing the visibility that their leaders have into their AI-enhanced workflow dynamics while ensuring that each team member is only tasked with handling what they’re capable of. This approach will further bolster organizations’ ability to construct distributed accountability frameworks that account for diverse accountability needs across AI usage scenarios.
-
Regulatory Uncertainty: Regulations are often designed reactively, in response to how a product or service is used and the impacts it generates. However, AI moves so fast that in many cases, policymakers likely won’t have the opportunity to think proactively, though this doesn’t mean that consequential regulations aren’t on the horizon. AI literate Organizations could partially sidestep this dilemma, and while predicting emerging regulations with complete certainty remains an impossible task, anticipating the major issues regulations might combat is much easier when you have an inherently communicative and adaptive workforce that’s regularly tinkering with, exploring, and learning from AI tools at your disposal.
-
Cross-Functional Collaboration and Coordination: Recall that as AI literacy scales, knowledge sharing and collaboration rates between individuals and groups within an organization increase as competitive advantages become harder to identify. This phenomenon could also foster better alignment between the various functions of an organization and their respective RAI objectives, enabling disparate teams to coordinate their actions in unison to achieve common goals—a workforce in which all individuals are aligned with the overall goal of AI skills and knowledge acquisition is less likely to perpetuate collective action problems that drain valuable time and resources to solve.
-
Resource and Expertise Constraints: Full-scale AI literacy reduces an organization’s dependence on external experts by distributing foundational AI knowledge both horizontally and vertically, equipping teams with ownership of governance tasks while allowing them to optimize resource use and build organizational resilience. Where some external expertise or resources are nonetheless required to achieve a key governance objective, organizations can look to their various AI literate teams and departments to quickly and efficiently identify the gaps that must be filled, lowering the risk of paying high costs for “expertise” that doesn’t solve the problem.
Recommendations and Conclusion
This has been a meaty discussion to say the least, though we’re optimistic that we’ve done our job in making readers aware of how AI literacy doesn’t only inspire RAI, but also drives it.
Our next piece in this series will be less dense and more practical, exploring a selection of hypothetical case studies that demonstrate the importance of AI skills, knowledge, and awareness for RAI, along with several predictions on the future of AI literacy. In the meantime, we leave readers with the following recommendations:
-
Look beyond mainstream AI tools to see what else is out there. You never know what you may find, especially as AI advances and proliferates. However, conduct initial experimentation with new AI tools on your own time to avoid introducing preventable risks (e.g., sensitive data handling) in professional settings.
-
If you’re a leader, invest in AI literacy now. Though it may be costly to train your workforce, doing so will make your organization more trustworthy, resilient, innovative, and prepared for incoming regulations and technological changes, saving you a lot of time and resources in the long run.
-
Realize that AI skills must not look identical for all AI users—it’s acceptable for users to become experts on different AI tools. On the other hand, users can also specialize with AI in specific domains like content creation vs. code refinement, even if they’re using the same tool.
-
Attack AI literacy from multiple angles and perspectives. AI literacy is about more than building AI skills, and the sooner you understand that, the more equipped you will be to create and source opportunities with AI while maintaining an upstanding reputation and capitalizing on innovation.
-
Understand that AI literacy supports change acceptance. Change resistance remains a significant barrier to AI adoption, and if organizations wish to innovate with AI, building an AI literate workforce will lay the groundwork for AI-driven transformations as opposed to disruptions.
-
Look at what other organizations and sectors are doing to address their AI skills needs. By comprehending which AI skills market pressures favor and disfavor, organizations can create targeted AI literacy campaigns that maximize ROI.
-
Ask your workforce what they seek to gain from AI literacy before developing and implementing an organization-wide campaign. AI literacy campaigns should closely align with employee needs, otherwise, organizations risk pursuing irrelevant skills procurement initiatives while potentially facilitating change resistance.
-
Evaluate your organization’s AI literacy strategy biannually, if not more frequently (e.g., quarterly). AI literacy is an ongoing process, and failure to critically examine it regularly and at scale could result in a workforce that’s no longer equipped to enact AI opportunities while combatting relevant challenges.
For readers interested in exploring other topics in the RAI, risk management, safety governance, ethics, and GenAI space, we suggest following our blog, where you can find plenty of detailed content that helps you maintain an up-to-date perspective on these topics.
Alternatively, if you’re eager to begin your AI governance and risk management journey, we invite you to check out our RAI platform in addition to our newly released AI Policy Analyzer.