November 14, 2024
Driving Responsible AI Practices: AI Literacy at Work
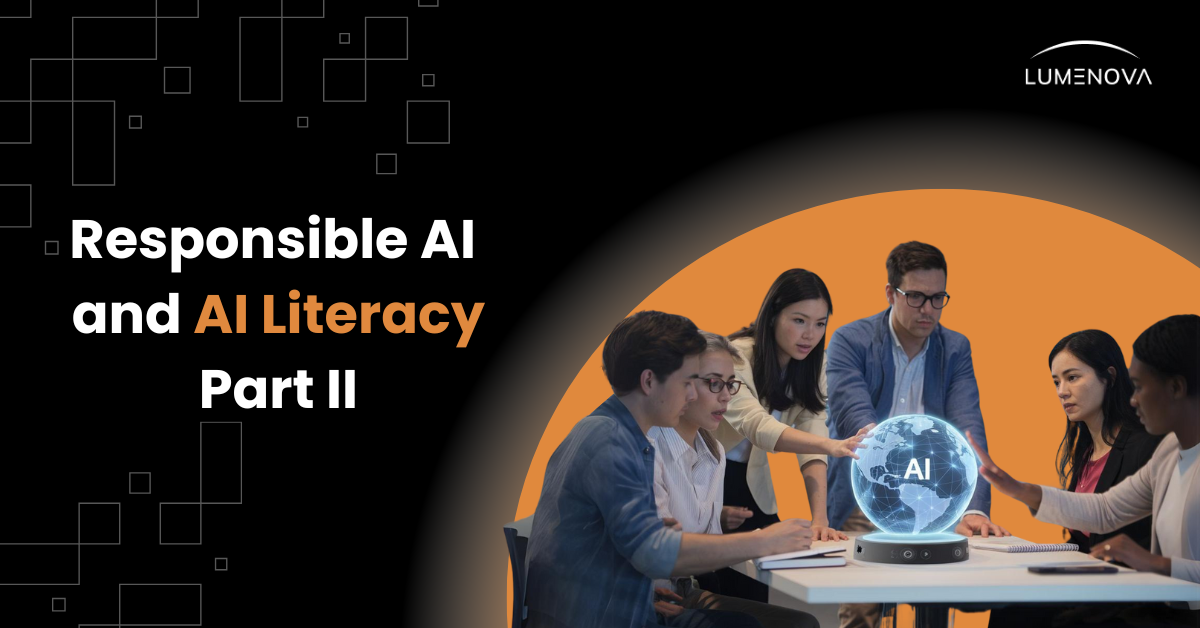
Contents
In part I of this series, we examined two core ideas in-depth: 1) why AI literacy can lay a strong and resilient foundation for responsible AI (RAI) best practices, and 2) how AI literacy can be leveraged as a mechanism that enables organizations to effectively fulfill many of their AI governance and risk management needs. We also took a stab at defining AI literacy in actionable yet generalizable terms, reiterating this definition below to refresh readers’ memory.
“Definition: The perpetual process of developing, maintaining, and improving AI-specific skills in a contextually appropriate manner, awareness of AI tools, capabilities, limitations, and use cases, and understanding of the evolution, context, and manifestation of pertinent AI risks, benefits, and real-world impacts.”
In contrast to our previous discussion, this piece will begin by illustrating three hypothetical case studies, each of which seeks to model how AI literacy can inspire positive downstream effects for AI governance and risk management within small, medium, and large organizations. We’ve adopted a scale-oriented perspective here because the ability to safely and effectively scale AI initiatives remains a significant socio-technical barrier to AI adoption while representing a key growth objective.
Ultimately, in presenting these case studies, we hope to demonstrate how AI literacy improves an organization’s agility, resilience, and transformative potential, enhancing how it accounts for the fluctuating dynamics of the AI innovation, safety, and regulatory ecosystems while carefully balancing the evolving needs of its workforce and stakeholders.
We’ll conclude with several bold predictions on the future of AI literacy, envisioning how this field may change over the next few years. However, we’ve split these predictions into two categories—optimistic and pessimistic—showcasing two potential future trajectories that, at this current juncture, appear equally plausible.
In other words, the sentiment and general interest surrounding AI literacy now inspires both significant hopes and considerable concerns, muddying the right path forward. Importantly, we expect this ambiguity will persist for some time until the first notable large-scale AI literacy case studies emerge—for readers interested in tracking these potential developments, we suggest keeping an eye on leading academic institutions, major tech companies, eduction-oriented non-profits, and online learning platforms.
Overall, this piece is less dense and more pragmatic than its predecessor, providing readers with insights into the potential benefits of AI literacy within real-world professional settings. What we want more than anything is to inspire our readers to realize how AI literacy can empower them, their coworkers, and their organizations with more adaptability, creativity, independent thought, and control over their future.
AI Literacy: Case Studies
The following case studies explore how AI literacy can improve AI governance and risk management practices, presenting a few real-world organizational narratives that operate at different scales. However, the “neatness” of these stories shouldn’t be misinterpreted as ease—you can’t simply snap your fingers and assume that AI literacy will solve all your problems. We chose to frame these narratives in this way to help readers grasp the powerful role that AI literacy could play in fostering RAI best practices.
Case Study 1: Local Marketing Agency
Neon Marketing, a 20-person boutique marketing agency based in New York City, has noted an uptick in its employees’ use of various popular generative AI (GenAI) models ranging from general-purpose models like ChatGPT and Claude to visual content generators like DALL-E and Midjourney. The agency primarily services early-stage tech startups and local businesses, many of which have begun submitting complaints regarding minor incidents involving notable content errors, damaging client relationships, and reducing overall trust and confidence in their reputations.
However, due to Neon’s exemplary history of owning up to its mistakes and promptly fixing them, most of its clients are determined to give the agency the benefit of the doubt before severing all ties. To quickly resolve its client-facing problems without abandoning AI use, Neon decides to implement an agency-wide AI literacy program.
During the initial assessment phase, all Neon’s members come together to comprehensively and transparently audit the agency’s AI usage, with each staff member reporting on how they leverage AI and for what purpose during day-to-day operations. After a series of in-depth weekly meetings conducted over a 6-week period, key knowledge gaps are identified and mapped onto relevant risk areas in employees' workflow—these meetings also reveal a small selection of employees that emerge as more advanced AI users. This is where phase two begins.
After knowledge gaps and risks are prioritized and aligned, Neon develops several AI workshops, each 1-hour in length and intended to be administered weekly over a 3-month timeframe by the advanced AI users previously identified. Importantly, workshops aren’t generically designed—each covers a specific AI use case, pertinent risks, and the skills required to enact it effectively, affording employees with targeted hands-on practice with AI tools directly relevant to their workflow. Within one month, Neon has documented numerous cases of AI failures and successes, and by the time phase two ends, the agency has successfully developed a robust set of internal best practices.
Roughly 4 months into its AI literacy program, Neon now recognizes the need for a detailed AI governance framework. By being aware of how their employees use AI, having access to numerous documented AI failures and successes, and operating on functional internal best practices, Neon’s governance objectives materialize with relative ease. The agency establishes an AI tools approval and review process, role-specific AI usage guidelines, human-in-the-loop systems that serve as a peer-review mechanism for AI outputs, and easily interpretable client disclosure protocols for AI-generated content. This whole process takes around two months to complete.
Within 8 months of launching its AI literacy program, Neon’s clients report back with deeply positive feedback, noting that no significant AI-related incidents have occurred while project delivery times and content revision requests have been fulfilled around 30% more efficiently. A few months later, via word-of-mouth, Neon lands 4 additional contracts with enterprise clients who are impressed by the agency’s ability to produce high-quality work at scale within constrained timeframes. Within one year, AI literacy has brought Neon from the brink of losing its business to expanding it beyond what it thought was possible.
Case Study 2: Regional Hospital Network
Duluth Health, a 3-hospital network with 600 beds and 2,500 staff members headquartered in Duluth, Minnesota, caters to urban and rural populations within local districts. However, Duluth Health is struggling to meet patient needs, as thousands from neighboring communities flock into the city and its outer rural areas, overwhelming the network’s hospitals beyond capacity. To account for the growing influx of patients and continue providing high-quality care, Duluth Health tentatively adopts AI diagnostic tools and administrative automation systems, possessing a low-level awareness of potential AI-induced patient privacy, safety, and liability concerns.
To adequately address these concerns while maintaining day-to-day operations, Duluth Health’s upper management decides to pilot an AI literacy program that centers on four domains of interest: 1) clinical staff (focused on diagnostic AI), 2) administrators (focused on automation systems), 3) managers (responsible for oversight and risk management), and 4) IT specialists (tasked with integration and security). Clinical and administrative staff are expressly prohibited from using AI until management has established a comprehensive distributed accountability system, IT has successfully and securely completed its integration objectives, and AI training has been conducted.
Once management and IT have resolved their early initiatives, Duluth Health, realizing that it lacks internal AI talent, decides to outsource, hiring a few consultants with vetted expertise in medical diagnostic and administrative automation tools. Over 6 months, these consultants conduct numerous one-on-one interviews with Duluth Health’s leading clinicians and administrators, gathering in-depth information on the daily tasks they must perform, and anticipating how they might change due to AI integration. The consultants then reconnect with management and IT, operationalizing these anticipated changes into monthly department-specific workshops, quarterly cross-functional training sessions, and specific policies that clearly delineate relevant AI risks.
To maintain agility and safety, management further requests that the consultants build online learning modules with easily interpretable and accessible competency tests that are administered at regular intervals—these tests soon emerge as a reliable mechanism for identifying clinicians and administrators who are skilled AI users. Viewing this as an opportunity, management then selects these high performers as the founding members of Duluth Health’s AI ethics board, who are now tasked with an entirely new repertoire of responsibilities.
Moving forward, Duluth Health’s AI ethics board develops feedback and reporting channels through which the network’s members can report on novel AI developments—this information is then leveraged to create a centralized and secure database in which AI incidents are comprehensively documented and reviewed, fostering audit preparedness and the further creation of a detailed and malleable AI tool evaluation framework. Overall, these feedback and reporting channels enable Duluth Health to maintain an agile yet responsible approach to AI-driven innovations, capitalizing on emergent opportunities for improving healthcare in line with increasing demands while avoiding risks linked to rushed or outdated AI integration practices.
Within approximately two and a half years of launching its AI literacy program, Duluth Health’s AI-assisted diagnostic accuracy rates reach 95%, patients indicate improved trust and satisfaction, liability for insurance premiums decreases, and the network is praised as a local leader in RAI integration. In fact, Duluth Health is now being approached by several innovative healthcare AI startups, each of which wishes to beta-test their new and powerful AI products and services within one of the network’s hospitals at low cost.
Case Study 3: Multinational Manufacturing Corporation
TechCore Manufacturing Co., a multinational manufacturing corporation with over 60,000 employees across 12 countries, has already integrated AI at scale to manage and optimize complex supply chain and production processes. Thus far, AI integration initiatives have proved moderately successful, however, a variety of recent AI incidents and regional compliance concerns have hindered TechCore’s attempts to further expand and operationalize new and exciting AI innovations.
Since TechCore has been leveraging AI and ML systems for well over a decade, their technical understanding of these tools and systems is solid—each branch of the corporation has a skilled IT team that’s dedicated to resolving the technical risks these systems inspire, dealing primarily with security, automation, and infrastructure-related issues. Where TechCore falls short is in its approach to AI governance—it lacks standardized risk assessment metrics, a reliable AI incident tracking and reporting system, regionally-specific AI governance teams, and robust AI training and upskilling programs and resources for non-technical employees.
To address these needs, TechCore implements a governance-centric AI literacy program. This program first establishes a 6-month executive leadership immersion course, requiring all members of upper management to deeply familiarize themselves with emerging risk management, governance, and RAI standards like the NIST AI RMF, ISO 42001, the EU AI Act, Executive Order on Safe, Secure, and Trustworthy AI, and OECD AI Principles.
Once executives reach a uniform understanding and consolidate what they’ve learned into a comprehensive AI governance, risk assessment, and reporting framework, they initiate a mandatory certification program for all of TechCore’s department heads—this program is rolled out in stages, targeting four departments simultaneously in 3-month intervals. Within one year, TechCore’s executives and department heads are aligned on the corporation’s AI governance and risk management strategy.
Fortunately, TechCore’s executives also realize that continuous AI oversight is crucial for further AI success, especially in terms of accounting for regional disparities. Consequently, four department heads who excelled in the certification program are handpicked as Chief AI Governance Officers, forming the organization’s first AI governance board and directly reporting to executive leadership on all things AI. Looking ahead, TechCore’s AI governance board will be responsible for running quarterly governance reviews with all department heads across each faction of the organization.
Next, TechCore’s department heads hone in on their regional workforce AI needs, holding three “town hall” meetings with each leadership team across key domains within their department. Employees are rewarded for participating in these meetings and actively encouraged to voice their AI-related concerns and hopes, ultimately creating the foundation for role-based AI training modules and impact assessments. Once created, these modules and assessments are vetted by regional IT teams for technical feasibility, evaluated by end-users in localized pilot programs, and then escalated to the AI governance board for in-depth review before full-scale implementation. As soon as the green light is given, modules and assessments are deployed in department pairs over 6 months.
When corporation-wide AI training is complete, executive leadership reconvenes, calling all department heads to report on the success of AI training initiatives. After a series of productive meetings that reveal multiple avenues for improvement, executives start to realize what they need to prioritize looking ahead—an AI procurement evaluation process, vendor and contractor AI guidelines, regular system audits and assessments, emergency response procedures, and cross-border data governance protocols. Had TechCore not implemented a governance-centric AI literacy program, this structure wouldn’t have existed, preventing the corporation from anticipating how it may need to evolve to continue balancing its needs for innovation with those of its workforce.
AI Literacy: Predictions
Here, we lay out two sets of predictions on the near-future (5-10 years from now) of AI literacy, the first of which is optimistic and the second of which is pessimistic. We’ve chosen this approach not because we don’t believe in the immense value and utility of AI literacy, but because it’s an experimental, nascent field that exists in the prominent shadow of far more well-understood fields like AI governance and safety, despite its potential foundational contribution to RAI.
Optimistic
-
AI literacy will emerge as a core subject in roughly half of public k-12 schools—by contrast, we predict that almost all private schools will teach it—building future generations of AI-savvy citizens, who possess a real-world understanding of AI’s potential while being acutely aware of its risks and limitations.
-
To vet professionals for desirable AI talent, third-party service providers will build standardized AI literacy certification programs that are role, tool, and industry-specific. These programs will be dynamic and relatively low cost while providing independent companies with the freedom to customize them and provide feedback.
-
Enterprises that have pioneered successful large-scale AI literacy campaigns will partner with state agencies, non-profits, academic institutions, and/or online learning platforms to offer free entry-level AI literacy courses and training, attempting to bolster their reputations through a visible commitment to democratizing AI.
-
AI literacy will become a prerequisite for policymakers, underlying the development of AI regulation that more closely reflects and balances the nuanced characteristics of safety and ethical considerations with real-world demands.
-
Small businesses, due to their limited scale, will be among the first organizations to achieve comprehensive AI literacy at every level of the business. This will empower them to more effectively compete with larger organizations while increasing their ability to source top-tier AI talent.
-
Digital media organizations will create and subsidize specialized AI reporting units, dedicated to public knowledge dissemination and engagement. AI users of all profiles and backgrounds will be able to submit detailed reports to these units while also gaining complete access to what others have submitted.
-
Corporate boards will make AI literacy a mandatory requirement for all their members, irrespective of whether they already serve on an AI governance or ethics board, fostering more robust human oversight and monitoring procedures that ultimately set new milestones for trust and confidence in AI.
-
As AI literacy scales, citizens will begin leading discussions on AI governance, ensuring that diverse societal values and preferences are internalized by policymakers so that future AI laws align with society’s best interests.
-
Leading universities and AI research hubs will begin crowdsourcing AI talent through AI literacy outreach initiatives that identify high-performers and provide them with an expedited application opportunity. This will create a new wave of grassroots innovation that pushes both the academia and research sectors to adopt a more holistic and representative view of AI development.
Pessimistic
-
Rapid AI advancements will dramatically outpace AI literacy efforts, leaving most people in a position where they have neither an understanding of AI nor the opportunity to influence the course of its further innovation. This gap will grow exponentially as AI continues to advance.
-
Only elite educational institutions and private-schools will offer AI literacy programs due to the resources and expertise required to develop and maintain them. This will cause a stark AI literacy divide that becomes progressively more polarized along socioeconomic lines.
-
To maintain agility and transformation initiatives in a volatile work landscape, organizations will prioritize hiring new AI talent over upskilling their existing workforce. This will fuel significant disruptions in the labor market, which will skew towards older employees and those without college degrees, exacerbating the AI literacy divide.
-
The federal government will make no attempt to impose mandatory AI literacy requirements on schools, policymakers, and companies, resulting in a total failure to democratize AI and ensure that further AI advancements never threaten fundamental human rights like autonomy and the right to education.
-
As AI innovations persist, major tech companies will derive new ways to exploit the general public’s lack of AI understanding, marketing novel AI products as inherently beneficial despite the slew of risks they inspire. This dynamic will be present in the regulatory ecosystem too, where tech companies will aggressively take advantage of non-AI literate policymakers to pursue their economic incentives.
-
Misinformation about AI capabilities and limitations will spread at a rate that outpaces accurate information, corrupting AI literacy and positioning as an unreliable mechanism through which to gain valid insights into the nature and evolution of AI tools and applications.
-
Small organizations will be left behind by larger organizations that possess the capital required to hire external AI expertise for building and administering robust internal AI literacy programs. This will make it extremely difficult for small businesses to innovate, encouraging the formation of markets that are even more susceptible to monopolies than they are today.
-
As AI systems become more complex, sophisticated, and autonomous, concretely identifying the skills required to operate or oversee them safely and effectively will become more difficult. This could create a false sense of confidence in AI deployments, leading to high-profile AI failures with serious consequences.
-
AI literacy initiatives will predominantly focus on technical aspects, neglecting social, ethical, economic, and governmental implications. This will not only miss the entire point of AI literacy, but also make it inaccessible to most people, emerging as yet another factor that contributes to the AI literacy divide.
-
The true future of AI literacy likely falls somewhere in between these two sets of predictions. However, our message remains the same: don’t wait for a handout and begin building AI literacy now. Even if AI advancements challenge the ability to maintain timely AI knowledge and skills, it’s still better to know something as opposed to nothing at all. AI literacy provides you with an opportunity to take control of your future and play a part in driving the development of technologies that clearly serve humanity’s best interests.
Conclusion
To recap, in part II of this series, we illustrated three hypothetical and organization-specific AI literacy case studies, highlighting how AI literacy can enhance AI governance and risk management practices at vastly different scales.
Following this, we made multiple predictions on the future of AI literacy from both optimistic and pessimistic viewpoints, revealing a potential middle ground that hints at the future trajectory of this field.
While this is how we’ve chosen to conclude this series, we expect that AI literacy will warrant further in-depth exploration and research, so we urge readers to stay tuned for our next pieces on this topic.
On the other hand, for readers interested in diving into more well-known areas like AI governance and policy, risk management, safety and ethics, and GenAI, we suggest following Lumenova’s blog, where you can track regular updates and insights across these fields.
For those who are inspired or actively engage in developing AI governance and risk management frameworks and procedures, we invite you to check out Lumenova’s RAI platform and AI policy analyzer, both of which are built to comprehensively address your governance and risk management needs.