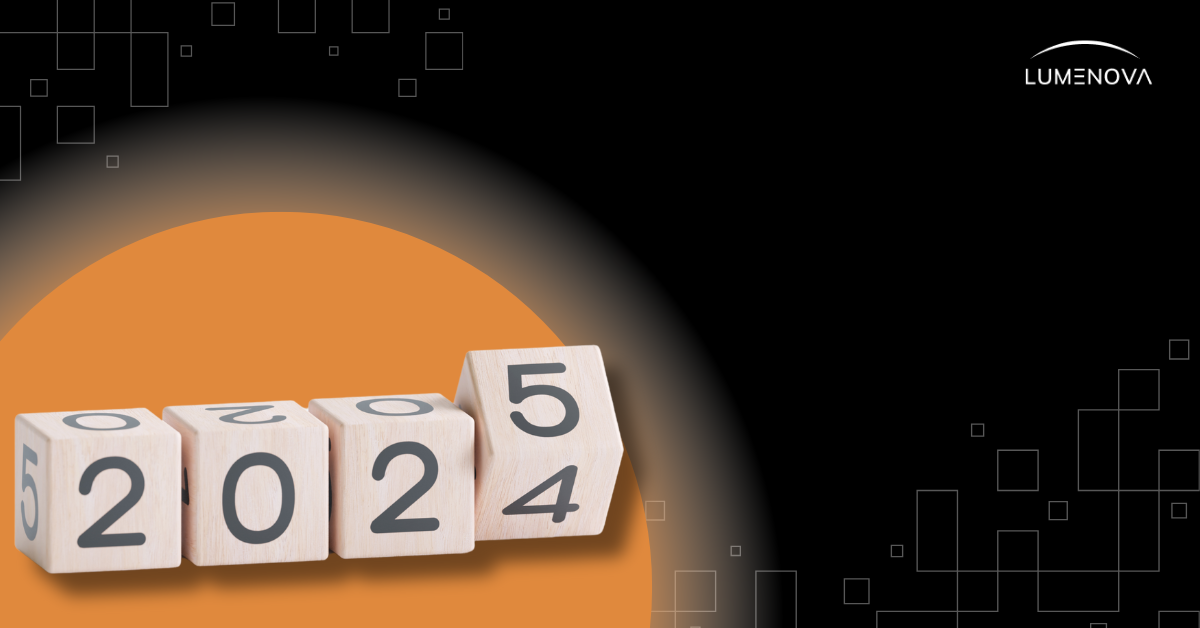
Contents
In February, we made several AI policy predictions for 2024—now that we’re nearing the end of this year, we’re revisiting this topic, shifting our focus to 2025. While the US and many other countries have plenty of work left to do with AI regulation, major regulatory milestones and controversies like the EU AI Act and California’s SB 1047 continue to push AI policy discussions further into the limelight.
Although the AI policy discourse is becoming more accessible, popularized, and informed by multiple factions of society, we still don’t have much to go on for what works and what doesn’t. For example, the real-world consequences of the General Data Protection Regulation (GDPR) Act, enacted in 2018, are still being felt today, not only among those who enforced and designed the Act or are subject to its requirements but also among nations seeking to define, implement, and improve their own legal data protection strategies. Even with regulation as influential and effective (within reason) as the GDPR, we’re still discovering ways to ameliorate and modify it, especially as data-driven technologies advance.
More subtly, this GDPR example also illustrates a foundational characteristic of policymaking—the process of developing regulation is ongoing, flawed, and frequently laden with uncertainty. In some cases, proactively addressing the imperfections and uncertainties of a regulatory strategy is easier insofar as what the regulation targets, whether it’s a good, service, or socio-economic phenomenon, isn’t likely to change dramatically within a short timeframe.
However, when we consider the nature of AI, we must recognize that we’re dealing with a technology that can and will advance at a rate that surpasses human comprehension, compromising our ability to manage it reliably, safely, and for the good of society.
Technology policy is almost always reactive—this explains why many AI policies that exist today, most notably the EU AI Act, are intentionally designed to be flexible and adaptable, accommodating and responding to AI advancements and impacts as they emerge. It’s also important to note that AI governance and safety aren’t exactly “novel” fields, and many of the research advancements that enabled the technology we see today can be traced back years if not decades—we knew how crucial it would be to regulate AI long before ChatGPT and yet we didn’t do it.
To this point, an overarching implicit objective of this piece—and many of the other regulation-centric pieces we’ve published—is to bolster and support proactive approaches to AI policymaking whereby we account not only for what is happening but also for what we have good reason to expect will happen. We’ll begin by examining the core issues AI policies have targeted over the last year, painting a macro-level picture of the AI policy landscape. Next, we’ll explore a series of issues we predict AI policies will target within the next year, following up with an inquiry into the challenges that may prevent such issues from being solved. We’ll conclude with several recommendations, offering readers pragmatic forward-looking guidance on AI policy.
AI Policy in 2024: A Review
2024 can be characterized as the year in which AI policy “took off” at a global scale. While high-profile governance resources like the EU AI Act, NIST AI RMF, ISO 42001, and OECD AI Principles continue to serve as cornerstones for AI policymaking, the US, EU, ISO, and OECD aren’t the only international bodies racing toward building legal guidelines to ensure AI is safe and ethical.
Canada’s AI and Data Act, Australia’s AI Ethics Principles, the UK’s Framework for AI Regulation, the ASEAN Guide on AI Governance and Ethics, and the World Economic Forum’s AI Governance Alliance—these examples demonstrate the global engagement and interest that AI governance is garnering. Moreover, they also hint at the emergence of a common language through which to understand the nature of AI, the risks and benefits it presents, and the real-world impacts it drives.
In this respect, there are several core AI policy issues worth discussing—issues that now appear to transcend national boundaries:
- Transparency, Accountability, and Consumer Protection: The immense power and potential of AI requires the ability to ensure that the impacts it generates are predominantly positive for as many people as possible—transparency, accountability, and consumer protection are beginning to form the core of many emerging AI legislations. Accountability relies on a transparent understanding of the decision-making processes that AI is involved in and orchestrates, and consumer protection is predicated upon the capacity to hold individuals, companies, and organizations accountable for their AI-related actions and initiatives. These principles lay the groundwork for their superiors, including fairness, safety, non-maleficence, trust, and the equitable distribution of AI benefits over time.
- Bias and Discrimination: Bias in AI systems, whether it stems from algorithms, training data, operational environments, or some other source, has presented a major challenge to the AI innovation ecosystem throughout its existence. If we are to leverage this technology safely, ethically, and in alignment with fundamental human values, we must be able to trust it beyond a reasonable doubt—the first step in creating this trust is ensuring that the decisions these systems drive and support are fair, equitable, and consistent. However, this involves being collectively honest with ourselves and building a deep comprehension of our faults as societies and cultures, identifying the sources behind our behaviors, actions, and values that preclude us from creating a better world—AI tends to mirror our humanity, and our humanity is imperfect.
- Government AI Procurement: Even in democracies, the line between citizens being the subjects of their governments vs. governments existing solely to serve the interests of their citizens is easily blurred. AI can do a lot of good, especially in the hands of government, however, the blind belief that the institutions of government will always do the “right” thing is both naive and misplaced. Citizens must be able to exercise their agency in society and to do so, they must also be able to protect and preserve their rights just as governments do—from an ethical standpoint, the rights of citizens supersede the rights of government, despite the irony that governments exist to protect citizen’s rights.
- Automation Risks: While technology innovation has historically influenced the creation of new jobs and markets, the destruction or elimination of existing jobs and markets tends to be a prerequisite for automation-induced change and adaptation. Understanding the automation risks that AI presents, both in terms of scale and timeframe, is crucial to building a future in which AI enables transformation as opposed to disruption, and ultimately, scalable socio-economic prosperity. On a deeper level, entertaining and anticipating these risks allows us to intuit and predict where reliance on AI is justified, curbing the probability of future large-scale events like human enfeeblement and loss of control scenarios.
- Critical Infrastructure Risks: From supply chain and energy grid optimization to predictive maintenance and emergency response, AI can provide value and utility across numerous critical infrastructure domains. Nevertheless, as AI increasingly integrates into critical infrastructures, novel vulnerabilities and failure modes will emerge—even if AI-assisted critical infrastructures are designed with redundancy and robustness in mind, a partial failure could still affect millions of citizens (in other cases, partial failures could trigger failure cascades that reverberate through multiple infrastructures). In a similar vein, we also run the risk of adversarial attacks from foreign powers or actors with malicious intent, particularly in areas like national security and the provision of critical goods and services.
- Deepfakes and Elections: Advanced generative AI (GenAI) systems can create multi-modal content that is virtually indistinguishable from human-made content and incredibly difficult to detect and authenticate without clear disclaimers. Deepfakes are an obvious concern here, especially when we consider the impacts of their proliferation at the population scale—AI-driven mass manipulation, coercion, indoctrination, and the overall distortion of collective truth and reality present a serious threat to democracy, most notably during election cycles when political tensions are high and digital information ecosystems are saturated with partisan rhetoric.
- Frontier AI Development: While there are thousands of AI companies, only a handful have the resources, expertise, and infrastructure to pursue frontier AI development, regularly releasing more capable and sophisticated AI models that non-linearly increase the innovation gap between them and their smaller competitors. Such companies are already valued anywhere from tens to hundreds of billions—even trillions in some cases (e.g., Google or Meta)—which suggests that we are predisposed to a path where power will become concentrated among a few. To be clear, this is not a guaranteed outcome, though it is one that we need to pay close attention to. Though California’s SB 1047 was vetoed, the fact that it made it as far as it did is promising.
- AI Literacy and The Digital Divide: If AI is not democratized somehow and soon, those who have adopted this technology early will have a vastly disproportionate learning advantage over those who are behind the curve—an advantage that could build by orders of magnitude as time passes. The elevated emphasis that is being placed on AI literacy is one of the first tangible steps in reducing the risk of a national and/or global digital divide—the more people understand how to use the technology, identify and anticipate its risks, benefits, and limitations, and prepare for its impacts, the more likely we are to support a world in which AI is leveraged safely, effectively, and beneficially.
To reiterate, the picture we’ve painted here does not illustrate the AI policy landscape at a granular level. However, if we were to start getting into the micro-level details of what most AI policies support, they would fall under the umbrella of the issues we’ve just examined.
AI Policy Predictions for 2025
Certain predictions we make here, for instance, a targeted and in-depth regulatory focus on catastrophic AI risks, have already been alluded to in existing policies like the Biden Administration’s Executive Order on Safe, Secure, and Trustworthy AI and the EU AI Act. However, it is one thing to consider the potential for catastrophic AI risks and another thing entirely to develop and implement concrete strategies through which to address them. Risk acknowledgment is the first step in ensuring a safe and beneficial AI-driven future, but it is by no means that catalyst that will bring this future to fruition.
With that being said, let’s explore several predictions for the future of AI policy in 2025, as well as the challenges that may stand in the way of these predictions moving from theory to practice.
Predictions
- Misinformation Feedback Loops: As AI systems learn from their environments, users, and new training data while integrating with an expanding variety of dynamic digital platforms, the risk of high-frequency and large-scale misinformation feedback loops can’t be ignored. Mitigating the evolving array of factors that affect the emergence of these feedback loops will become a central concern for policymakers moving forward.
- AI-Washing: Exaggerating AI’s true involvement and role in a product will continue to damage the technology’s overall trustworthiness. If this damage escalates to a point beyond repair, humanity could be left with no choice but to abandon AI entirely, precluding itself from reaping the future benefits it could inspire. Regulations will need to crack down on AI-washing, primarily in the interest of preserving trust in AI and ensuring that AI-assisted products are transparently communicated to consumers and end-users. Another dimension here involves safety—if companies lie about how much they use AI in a product, even if it’s overstated, certain AI risks could be overlooked, resulting in a product that’s deployed irresponsibly and causes harm.
- AI Monopolies: Factors such as network effects, data advantages, infrastructure and computing requirements, technical talent concentration, market integration and platform power, and IP rights and patents could drive AI market concentration and potential monopolies. In this respect, regulators will be required to consider the risk of innovation stagnation, privacy and data control by those “at the top”, economic inequality and disproportionate market influence, and overall access to and pricing of AI services. However, as a word of caution, initial attempts to regulate AI monopolies might not be as fruitful as we’d hope.
- AI Agent Risks: AI agents inspire substantial risks across public safety and security, privacy, data, and consumer protection, market dynamics, democratic processes, and even the sociocultural fabric that defines human relationships and societies. Seeing as agentic AI systems are still in the early stages of their evolution, policymakers are under serious pressure to ensure their responsible development and deployment before things spiral out of control. To gain detailed insights into the AI agent risk repertoire alongside a realistic conception of this technology, we advise readers to check out our four-part series on this topic.
- AI Deception and Coercion: AI deception and coercion can take several different forms, from AI systems outputting false information with conviction and manipulating human emotions through fabricated relationships to exploiting cognitive or behavioral biases and hiding certain capabilities or limitations. These risks are separate but not incommensurable from those inspired by bad actors, who will encounter fewer barriers to human-motivated AI-driven deception and coercion attempts as AI advances. Policymakers will need to build legal frameworks for authenticating AI identities, educating citizens about AI manipulation tactics, and the creation of AI deception detection tools and safeguards.
- Catastrophic AI Risks: From proxy gaming and power-seeking behavior to critical infrastructure attacks, bioterrorism, and cyberwarfare, catastrophic AI risks are no longer merely theoretical, increasing in relative probability with each day that AI becomes more advanced. We think this coming year will mark the transition from simply assessing the probability of these risks with loosely defined predictive metrics to developing the first sufficiently targeted, detailed, and standardized technical and regulatory methodologies for understanding how, why, and when these risks might manifest themselves.
- Transhumanism: Whether or not one views the philosophy that humans are destined to augment themselves by synthesizing with technology as troublesome or acceptable, the issue of transhumanism is one that will need to be addressed, particularly as AI-powered biotechnologies like Neuralink start to redefine the boundaries of what’s possible with human-machine interfaces. The value-based questions these technologies raise, from both legal and ethical standpoints, reveal answers that border on utopian and dystopian rhetoric, so we should scrutinize this issue with the utmost care.
Challenges
- Misinformation Feedback Loops: There is a growing concern that we’re running out of high-quality data on which to train future AI systems, a problem compounded by the fact that even state-of-the-art models remain prone to hallucinations. Synthetic data generation has been proposed as a solution to bypassing data availability limitations, though it’s unclear whether this will result in AI systems that are consistently more accurate and truthful—developing standardized legal and technical parameters for auditing systems trained on synthetic data will be tricky, especially as the digital information ecosystem becomes more saturated with AI-generated content.
- AI-Washing: Understanding precisely how much AI contributes to a product before and after market deployment will become more difficult as AI is integrated into a wider variety of goods and services, particularly those operating as edge devices. Due to the AI hype cycle, companies have a potent incentive to overstate AI’s involvement in the products they release—reliable methods for measuring the degree to which AI drives a given product offering and holding such companies accountable have yet to be constructed.
- AI Monopolies: As frontier AI companies expand their global reach, mitigating their financial power and influence could prove deeply challenging. Regulatory penalties in the hundreds of millions to the billion-dollar range may appear to be a viable solution, but what happens when these companies can afford to pay penalties while their smaller competitors are crippled by them? On the one hand, compliance-driven competitor buy-outs are a real risk, and on the other, some companies may simply choose to pull their products from a market to avoid penalties. Policymakers must find a steady balance between these factors where market competition remains intact without losing access to potential AI benefits.
- AI Agent Risks: AI agents could spread like wildfire, whether they are released by official AI companies, open-sourced, or proliferate as knock-offs of existing models—gaining visibility into who uses these agents and for what purpose, degree, and at what scale, presents a substantial regulatory hurdle, particularly as agentic AI capabilities become more sophisticated. To further complicate things, the possibility that AI agents themselves will control and operate other AI agents, creating profoundly complex and difficult-to-interpret multi-agent systems and networks, doesn’t appear unlikely. The ability to track and monitor AI agents will prove to be a monumental task.
- AI Deception and Coercion: We know that social media can be leveraged to manipulate human behavior en masse. With AI, we must entertain the two-fold idea that it’s becoming far easier for bad actors to exploit this technology to cause widespread psychological harm while there’s also evidence to suggest that the technology itself is already capable of deceiving and coercing humans. Policymakers are thus forced to confront the problem of anticipating the intentions of independent bad actors and AI systems, considering questions for which we don’t yet have a clear answer (e.g., why would AI want to cooperate with humans or other AIs?).
- Transhumanism: Transhumanism is a precarious issue to regulate. For instance, many transhumanist enhancements could be voluntary, blurring the line between therapy and treatment—implanting Neuralink to restore communication vs. enhancing information processing capabilities. This line becomes even more obscured when therapy itself risks turning into enhancement (e.g., restoring communication and enhancing information processing). The nature of AI-powered transhumanism could also make it extremely difficult to detect enhancements and gain visibility into cross-border data flows that contain novel types of data we have yet to understand fully. The implications of this technology, even if it isn’t fully realized yet, are monumental.
Recommendations and Conclusion
Below, are highlighted a series of actionable recommendations for navigating and preparing for the future of the AI policy landscape.
- Establish and implement protocols for halting AI deployment practices when specific capabilities thresholds are reached or certain risks are identified, even in a diluted form.
- Build internal teams that are dedicated to stress-testing and probing model capabilities and limitations, with a specific focus on identifying vulnerabilities and emergent properties.
- For AI systems that have the potential to influence human behavior, mandate human-in-the-loop oversight and regular psychological impact feedback sessions with end-users.
- Where agentic AI systems perform coordinated functions or tasks autonomously, ensure the presence of emergency shutdown mechanisms and measures that enable maximum transparency into their decision-making processes.
- Design specific policies for AI agents that consider the unique risks they pose and the functions they play within an organization, especially where multi-agent systems exist.
- Regularly assess advanced AI models for deceptive, manipulative, or coercive tendencies and educate users on the factors that could contribute to this kind of AI behavior.
- Define and implement metrics for measuring to what extent and degree AI contributes to a specific product or service to provide transparent visibility into AI-assisted offerings.
- At the national and international level, fund large-scale collaborative research targeting the safety and ethics of AI-powered transhumanism, the dynamics of multi-agent systems, AI deception and coercion, and the democratization of AI.
For readers interested in expanding their AI policy knowledge base and exploring additional topics across AI governance, risk management, ethics and safety, and GenAI, we suggest following Lumenova’s blog.
For those in the immature to mature stages of building AI governance and risk management standards, policies, and frameworks, we invite you to experiment with Lumenova’s comprehensive responsible AI platform and AI policy analyzer.