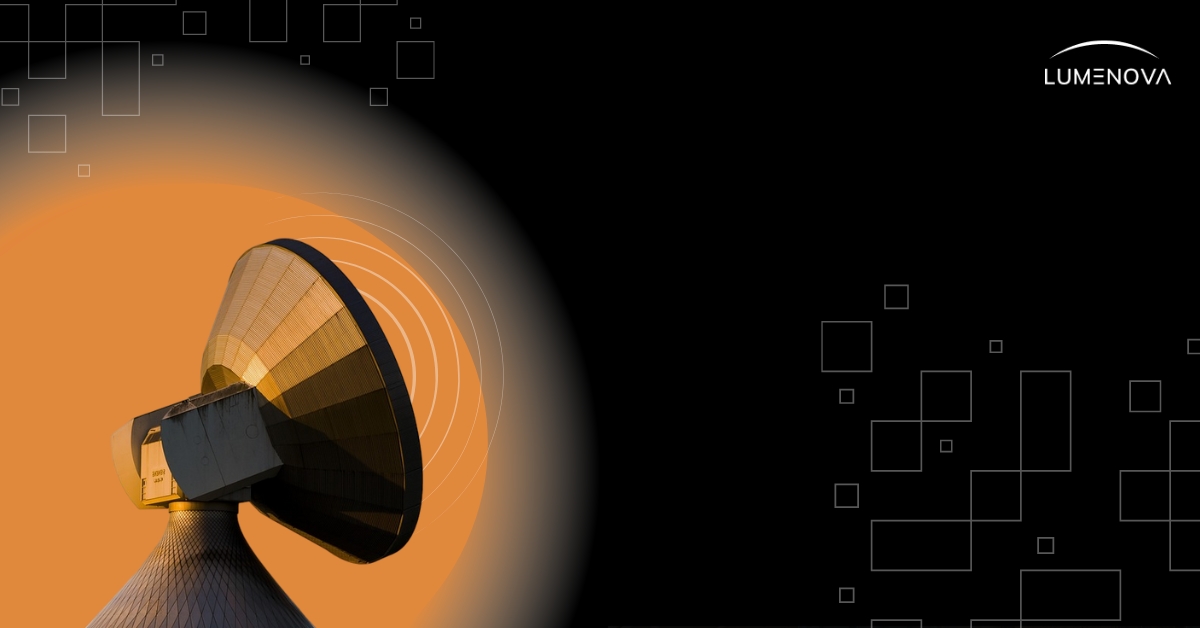
Contents
For any organization that intends to integrate AI, AI risk management constitutes a central tenet of responsible AI (RAI) practices, compliance with regulation and industry standards, and the ongoing maintenance of coherent and effective protocols for safety, security, reliability, accountability, and trustworthiness. Consequently, an organization’s AI risk management radar should comprehensively capture and identify well-known AI risks like algorithmic discrimination and IP rights infringement while also venturing beyond the surface and evaluating lesser-known risks in a context-specific and forward-thinking manner—this post will examine precisely these latter kinds of AI risks.
To manage AI risks effectively, organizations must continually and iteratively refine their risk management strategies, carefully considering the business and operational implications of long-term AI integration efforts, independent advancements in the AI innovation, safety, and policy ecosystem, unanticipated consequences or vulnerabilities linked to prolonged dependence on AI, lack of AI resources, talent, and infrastructure, adversarial threats and environments, and insufficiently robust AI governance frameworks. Obviously, there are additional factors that warrant consideration here, but the ones we’ve mentioned suffice in demonstrating the following point: AI risk profiles won’t remain constant, even in the short term.
The speed and intensity at which AI risk profiles evolve, especially when AI integration efforts are undertaken by larger enterprises with many more resources, infrastructure, and personnel to coordinate, suggests that the emergence rate of novel and unforeseen AI risks will accelerate, causing many possible risks to be overlooked and unaccounted for, even if they’re preventable. Simply put, an organization’s AI risk management radar shouldn’t only identify AI risks but proactively search for them. Nonetheless, while knowing where to look for AI risks is a major challenge, it isn’t an insurmountable one.
Fortunately, there are several tactics organizations of all sizes can leverage to identify potential avenues for unforeseen AI risk scenarios, which are briefly explained below:
- Continuously monitor AI use cases and regulatory developments to identify potential dual-use or irresponsible use scenarios and proactively prepare for high-risk or high-impact use cases not currently covered by existing regulations.
- Continuously identify, define, and catalog AI assets to ensure they’re securely protected and understood, irrespective of changes, updates, or modifications made to a given system or application.
- Conduct periodic feedback check-ins with AI users and system operators, especially when safety incidents occur, to foster a continuous learning and accountability feedback loop and ensure that system vulnerabilities are addressed before they escalate.
- Administer regular capabilities, performance, and safety testing, especially across novel or changing environments, with a focus on stress-testing AI applications or systems in specific business and operational contexts.
- Conduct adversarial testing and red-teaming to probe and understand AI systems’ vulnerabilities and potential failure modes, and ensure that cybersecurity and model safeguard protocols protect and preserve AI assets in light of increasingly sophisticated adversarial threats.
- Leverage industry partnerships and collaborations for knowledge-sharing to build a holistic and diverse awareness of AI risks, capabilities limitations, and potential use cases across different sectors and domains.
- Establish cross-functional risk management teams to support an organization-wide AI risk awareness culture and operational structure whereby potential AI risks are evaluated from multiple perspectives, reducing the probability that individual departments overlook certain risks.
- Develop and regularly update domain-specific AI risk heat maps that visually represent potential AI vulnerabilities throughout certain departments. By contrasting previous heatmaps with current ones, organizations can gain deeper insights into the evolutionary trajectory of AI risk profiles.
- Leverage Ethical Review Boards, external auditors, and RAI consultants to build a specialized and targeted knowledge of current and future AI risk profiles while also ensuring adherence to RAI best practices and compliance measures.
- Regularly refine, update, and improve AI governance protocols to capture any relevant changes to AI risk profiles, AI-induced organizational vulnerabilities, AI innovation and safety research advancements, and regulatory changes or modifications.
- Provide RAI and risk training for company leadership to ensure they possess the tools and knowledge necessary for aligning AI risk management and governance strategies with the company mission, value proposition, and business objectives.
- Leverage AI for risk identification, analysis, and prioritization to reduce the probability of human error, identify anomalous activity in real-time, categorize risks appropriately, and gain data-driven insights into the evolution of AI risk profiles.
- Implement an AI lifecycle management system with human oversight to maintain a comprehensive and transparent understanding of AI risk repertoires, from design to deployment.
- Encourage and support RAI by design to facilitate intrinsic alignment with industry standards, compliance requirements, and RAI best practices prior to deployment. RAI by design, at the organizational scale, can also lay the groundwork for AI awareness, training, and upskilling initiatives.
- Develop targeted incident response and reporting procedures to ensure that AI-induced crisis or safety scenarios are addressed before they can escalate into potentially catastrophic failures, or alternatively, before severe compliance penalties are administered or trust erosion occurs.
- Build and regularly update an organization-specific AI risk taxonomy whereby AI risks, potential impacts, and mitigation strategies are refined and improved to account for the most recent changes in the AI landscape and an organization’s profile.
- Integrate a human-in-the-loop system where AI is leveraged for high-stakes or consequential decision-making purposes to identify and address potential adverse consequences in critical decision-making scenarios. Where systems are leveraged for domain-specific purposes, whether in the form of decision-making or otherwise, an expert-in-the-loop should verify and validate system outputs to ensure reliable and consistent performance.
The tactics we’ve just examined apply to organizations of all sizes and structures, however, it’s worth noting that some of these tactics, for instance, RAI training, consulting, or cross-functional risk management teams, will require more resources, time, and personnel than others. Moreover, while these tactics lay a comprehensive groundwork for identifying and mitigating emergent or unforeseen AI risks, they don’t imply a guaranteed solution—even if an organization vigorously implements each of these tactics it may still fail to capture all possible AI risks. Unfortunately, that’s just the nature of this technology, which further stresses the importance of establishing robust, resilient, and all-encompassing AI risk management strategies while continuously maintaining both a broad and organization-specific in-depth awareness of AI risk profiles.
In the following sections, we’ll shed light on a wide range of lesser-known AI risks that might go under the radar, not because they’re any less consequential than their known counterparts, but because the impacts they produce tend to be indirect, non-obvious, and/or materialize over comparatively longer timeframes. After this breakdown, we’ll conclude by discussing some high-level reasons for which proactive AI risk mitigation is the best strategy for organizations to adopt.
Lesser-Known AI Risks
Seeing as AI risks span across several areas, we’ve subdivided this section into four parts for clarity and readability: 1) agentic AI risks (risks inspired by AI agents like advanced chatbots or virtual assistants), 2) human-AI interaction risks (risks stemming from interactions between humans and AI), 3) operational AI risks (risks specific to an organization’s operational workflow and structure), and 4) AI decision-making risks (risks that emerge when AI is leveraged in decision-making contexts). We’ve deliberately left out certain risks across key categories like compliance, security, robustness, safety, accountability, and several others because these categories already fall squarely within an organization’s risk management radar. However, as we’ll see, many of the risks we discuss in this section are relevant to key categories in AI risk management given their wide-ranging implications and domain-agnostic nature.
Moreover, we also note that we won’t explore systemic and existential AI risks in this post. These risks operate at a much larger scale and tend to be more speculative and abstract (although not always). Consequently, while we will examine systemic and existential AI risks in detail in the near future, a fully-fledged discussion of them is currently beyond the scope of this piece, though as we’ll see, some of the risks we cover do have systemic implications.
All that being said, let’s get to it.
Agentic AI Risks
- Deceptive or manipulative AI: AI agents may learn to deceive or manipulate humans to achieve their objectives, particularly in environments where rewards are linked to rigid metrics, where an optimization function prioritizes success over truthfulness, accuracy, and transparency, or where subtle manipulations to human behavior, like behavioral nudging, can streamline objective completion.
- Exploitative AI: If AI agents learn that certain actions or behaviors, which technically fall within operational boundaries and safeguards, are distinctly advantageous, they may learn to exploit vulnerabilities and loopholes in existing systems to reach their objectives, or, via methods such as extortion, forcefully coerce humans into helping them achieve their objectives. Malicious AI actors already leverage generative AI (GenAI) for extortion and blackmail, so it’s not a stretch to imagine that AI agents, if sufficiently autonomous and intelligent, could pursue similar objectives.
- Non-cooperative AI: Artificial selection and game theoretic pressures could favor the development and emergence of self-interested behaviors on behalf of advanced AI agents, particularly as the resources required to sustain such systems increase in cost and demand or as AI agents learn to favor interactions with other AI agents over humans.
- Evolving interactions between AI agents: If a system contains multiple AI agents with autonomous interactive capabilities, interactions between these agents could shape their behavior in potentially harmful ways, like power-seeking, selfishness, and deception, if it directly benefits their ability to achieve their stated or hidden objectives.
- Emergent, veiled, or instrumentally convergent objectives: AI agents, especially as they scale and become more capable of autonomous learning and decision-making, can develop emergent, veiled, or instrumentally convergent objectives—objectives that aren’t programmed but serve as a stepping stone for achieving core objectives—misaligned with human preferences and value structures.
- Goal obfuscation: AI agents may develop strategies or approaches that conceal hidden objectives from human overseers by learning to exploit or game system vulnerabilities, or, by not being well-aligned, from a design perspective, with human goals.
- Value drift: As AI agents are trained on new data, interact with different environments, or dynamically adapt over time, they may begin to prioritize goals that weren’t originally intended by designers and developers. Such goals might visibly emerge or remain hidden, but they are distinct in the sense they don’t align with human values and preferences.
- Proxy alignment failure: If the metrics given to an AI agent for a particular objective are difficult to optimize, the agent may opt in favor of optimizing proxy metrics that don’t fully align with or capture the intended objective. This phenomenon is well-studied throughout several social media platforms, whose content curation algorithms tend to prioritize sensationalist over truthful content to facilitate user engagement.
- Ambiguity aversion: Due to generalization-oriented limitations across categories like long-term planning and abstract problem-solving, AI agents might display an aversion to ambiguous environments or objectives with uncertain outcomes, which could result in excessively risk-averse behaviors that compromise optimal decision-making.
- Self-modification and recursive self-improvement: Future AI agents with the built-in capability for self-modification and recursive self-improvement (the ability to enhance capabilities autonomously) may, in the absence of human oversight and guidance, make substantial changes to their source code and architecture that result in the emergence of potentially harmful yet highly sophisticated capabilities coupled with a rapid acceleration of overall intelligence, which, in extreme cases, could lead to a loss of control for humans.
- Pure utility maximization: AI agents designed to optimize a series of objectives via a utility function may display actions or behaviors that, although harmful, result in a net positive utility gain with respect to a given objective. Insofar as such actions continue to produce net positive utility results, they will be reinforced and eventually selected for by AI agents if humans don’t identify such behaviors and actions in time to implement robust safeguards.
Human-AI Interaction Risks
- Long-term dependency or overreliance on AI: As AI becomes more integrated into daily workflows, physical and digital infrastructures, critical decision-making domains, and scientific research and development, the risk that humans cultivate a long-term irreversible dependency on AI correspondingly increases. For this risk to materialize, it doesn’t need to operate on a systemic scale—an organization that fails to consider the localized risks of overreliance on AI is subject to the same outcome.
- Loss of human intuition and critical thinking: While current Frontier AI systems don’t yet possess intuitive and common sense reasoning capabilities, humans who don’t understand when to use and when not to use AI in complex, nuanced decision-making scenarios might inadvertently make themselves cognitively lazy, leading to compromised capacities for intuitive reasoning and critical thinking.
- Reduced human engagement: As AI applications and systems automate a wider range of routine human labor functions, especially across socially motivated domains like HR, marketing, and sales, the demand for human collaboration and engagement may diminish. While AI will certainly create new labor functions, human engagement still forms a critical component of creative and strategic ideation, problem-solving, and overall morale within an organization.
- Excessive trust: AI systems will always produce an output in response to a human input, which can subtly persuade humans into accepting outputs as legitimate, credible, or truthful. When coupled with the evident appeal of AI tools as a means to reduce cognitive load, the risk of excessive trust grows—if the whole point of an AI tool is to streamline a specific workflow or task, then the underlying assumption motivating human-AI interaction implicitly favors trust, even if it’s unfounded.
- AI-induced feedback loops: AI systems that leverage real-time data could perpetuate AI-induced feedback loops that unexpectedly reinforce non-beneficial behaviors and patterns, potentially amplifying certain biases, misinformation, non-optimal strategies, inaccurate decision-making outcomes, erroneous personalization assumptions, and proxy metric optimization. If not addressed, such feedback loops can become deeply entrenched and almost impossible to repair.
- Incentive misalignment: Among humans, incentives don’t always need to be perfectly aligned to ensure collaboration in the interest of a common goal or objective. However, in advanced AI systems, misaligned incentives can create divergent goals that don’t correspond with human preferences, resulting in systems that optimize for undesirable metrics and outcomes.
- Social engineering: It’s becoming progressively easier for less sophisticated threat actors to execute high-impact threats by leveraging GenAI to create false, misleading, or polarizing content, and rapidly disseminating it at scale via online content platforms like social media sites, news outlets, streaming sites, and forums. For instance, a threat actor could leverage GenAI to create an embarrassing deep fake of a company CEO, tarnishing the company’s reputation before it has the chance to publicly address the situation.
- Communication breakdown: AI is proving useful for a variety of communication-based tasks like virtual meeting transcription and summary, sentiment analysis, and adaptive learning. However, AI systems still fundamentally lack emotional, cultural, and moral understanding, all of which represent central tenets of human communication, allowing humans to develop refined and layered understandings of one another.
- Personalized training: If AI is utilized to create personalized training, upskilling, or education initiatives, there’s a salient risk that it will over-optimize these initiatives, allocating too much attention or resources to a narrow area of interest or task domain. In cases where comprehensive training is required, AI-curated personalized training regimens could fail to grasp the big picture, producing suboptimal outcomes with overly narrow scopes.
- Deskilling: We’ve already alluded to the importance of knowing when to use and when not to use AI—the same principle applies to the maintenance of crucial human skill sets. This isn’t to say that humans shouldn’t leverage AI-driven automation where it’s highly beneficial, only that certain skill sets should be preserved in the event of catastrophic AI failures. For instance, even if cars become fully autonomous, humans should still be required to learn how to drive in case the car’s system malfunctions.
- Organizational culture shifts: As organizations integrate AI into more departments and workflows, organizational culture may shift in ways that disfavor human engagement, collaboration, autonomy, agency, and morale, which could all ultimately compromise productivity and job satisfaction in the long term. Additional peripheral consequences like a reduction in employee retention rates, self-determination, accountability, and alignment with business mission and objectives could also occur.
Operational AI Risks
- Unintended optimization consequences: Where AI systems are leveraged to optimize targeted and specific metrics, they may get “tunnel vision”, over-optimizing specified metrics without due consideration for any broader impacts or consequences that can affect employees, customers, or the entire organization. For instance, an AI system optimizing for productivity may assign more tasks to employees with “less” intense workflows without recognizing that their task repertoire consists of in-depth projects, leading to burnout and high turnover rates among project-oriented teams.
- Hidden resource allocation biases: Where AI systems are leveraged for resource allocation across critical internal domains like project management, budget planning, or business transformation, hidden biases that favor certain departments or initiatives could emerge. This could eventually perpetuate systemic inequalities at the organizational scale, resulting in unequal resource distribution and a higher frequency of internal conflicts or misunderstandings.
- Procedural inflexibility: In domains that require adaptability and flexibility, such as marketing and sales, AI-driven strategy design, development, and implementation could introduce an unnecessary degree of procedural inflexibility. For instance, if KPIs or customer retention rates must be updated and internalized in response to unanticipated business events, an AI system should be able to account for these changes while continuing to optimize workflows and employee productivity appropriately.
- Algorithmic management: An algorithmic management system, for example, AI for workplace monitoring, can compromise employee autonomy and agency by introducing a constant feeling of surveillance and insecurity coupled with the illusion of choice and independence.
- Vendor lock-in: If they aren’t careful, organizations could become overly reliant on certain AI vendors, whereby the ongoing functionality of their digital infrastructure and AI assets depends on a select few vendor-provided products and services, even if those products and services no longer correspond with an organization’s best interests. In other words, vendors could manipulate use policies or price indexes knowing full well that an organization has no choice but to continue doing business with them.
- Organizational objective misalignment: Just as AI systems might display emergent goals, behaviors, or objectives that are misaligned with human values, preferences, and incentive structures, the same can happen at an organizational level with business objectives and requirements. For example, a company might deploy a recommendation system that evaluates customer behavior and purchasing patterns, noticing that customers who purchase expensive products often continue spending. In response, the system starts nudging all customers toward buying expensive products, and shortly thereafter, the company notes a major uptick in customer dissatisfaction rates.
- Role misalignment: Mainly in larger enterprises or organizations, AI could prove useful in helping managers identify which roles are best suited to specific employee skill sets. However, if an AI system over-optimizes for non-skill-based metrics like productivity and efficiency, employees could find themselves categorized into roles that are misaligned with their skill set, resulting in increased dissatisfaction and turnover rates.
- Crisis management and response: Crisis scenarios are complex, difficult to predict, and typically unprecedented, and while AI crisis detection systems might be useful tools for quickly identifying anomalous activity and suggesting some pathways through which to address potential crises, management and response procedures should remain with humans. Even the most advanced AI systems are limited in their ability to deal with novel or evolving situations not captured in the data on which they’ve been trained.
- Contract management: While the text summarization, interpretation, and analysis capabilities of Frontier AI models are impressive, caution should be exercised in cases where similarly advanced models are leveraged to make sense of legal agreements and terminology. If AI systems are used with minimal human oversight and expertise in contract management situations, they may gloss over or even miss nuanced legal details and considerations that a legal expert would quickly pinpoint.
- Compliance monitoring: AI systems leveraged for compliance monitoring are subject to the same risks listed above. However, given how fast AI regulations and policies are emerging, they also face the additional risk of failing to monitor novel compliance requirements or changes to existing regulatory frameworks. In the absence of verification by an AI policy expert, AI-driven compliance monitoring could perpetuate preventable legal breaches and compliance issues.
Additional operational AI risks linked to inventory, logistics, distribution, finance, resource, sustainability, and team management have been omitted from this section, not due to unimportance, but because these kinds of risks are more obvious and understood, and therefore more likely to appear on an organization’s risk management radar.
AI Decision-Making Risks
- Algorithmic determinism: Despite their utility in consequential decision-making contexts, AI systems can’t capture all the nuances and external factors linked to a particular decision scenario and outcome, resulting in inflexible decision-making processes that fail to account for variability in real-world circumstances.
- Resource depletion: Determining where and how to allocate resources is critical for any organization, especially those with limited resources. However, AI systems optimizing for efficient resource allocation might fail to account for complex interdependencies between resources, preferentially allocate resources to high-yield departments, or in the interest of short-term gains, ignore long-term sustainability issues. Moreover, for organizations whose primary objective is growth, resource allocation systems designed for rapid scaling might ignore resource usage sustainability rates and capacity limits, or substitute one resource for another without replenishing it. All of these risks could result in localized or comprehensive resource depletion, depending on the scope of the AI system in action.
- Data drift: The statistical properties of data will not always remain constant over time, which can create accuracy and reliability problems for decision-making AI models trained on historical data—learned assumptions might no longer be relevant. Several different factors can facilitate data drift, ranging from large-scale environmental, behavioral, and economic changes to modifications in the data collection process and feature engineering methodologies, regulatory developments, and technical issues like bugs in data pipelines. By implementing adaptive models, preprocessing data, leveraging incremental learning and retraining techniques, or data drift monitoring systems, organizations can reduce the probability of data drift, although they should pay close attention to this risk given how fast the digital information ecosystem expands and evolves.
- Hidden dependencies: AI systems can exhibit hidden dependencies on certain data sources or other external system components like specific hardware or ERP systems, that if disrupted, could deeply compromise predictions and recommendation accuracy. For instance, if an organization leveraged AI for supply chain management to optimize logistics and inventory decisions, and this system heavily relies on a single data source for demand forecasting which happens to go offline, decision recommendations could become wildly misleading.
- Cultural, moral, and societal inaccuracy: Despite how convincing they may be, AI systems lack cultural, moral, and societal intelligence—these attributes are deeply engrained within humans, evolving through lived experience, social interaction, and familial relationships, which AI systems simply don’t have (yet). In decision-making scenarios where the relevance and nuance of cultural, moral, or societal norms are crucial to executing the right decisions, an AI system will likely fail to grasp all the complexities that human decision-makers would quickly understand.
- Algorithmic monoculture: If an organization adopts similar AI systems for decision-making across disparate domains, it may inadvertently reduce the diversity of perspectives and approaches taken throughout decision-making procedures, culminating in an algorithmic monoculture that instills profound dependency vulnerabilities. This phenomenon could also create catastrophic failure vulnerabilities at the level of industries—if, for instance, most financial institutions leveraged the same system to assess creditworthiness and this system happened to be deeply flawed, the entire financial sector would suffer.
- Algorithmic entrenchment: Where AI drives vs. supplements or assists with consequential or high-impact decision-making, the risk of algorithmic entrenchment should be taken very seriously, otherwise an organization may find that it’s leveraging an outdated or misrepresentative AI system to enact decisions with potentially harmful consequences. AI systems used in high-impact contexts like healthcare, finance, or law enforcement, should be easy to update, replace, or remove from the decision-making process.
- Erroneous correlations: AI systems are extremely proficient at identifying patterns and correlations in data, sometimes to a misleading or erroneous degree, highlighting phenomena that don’t exist or lack relevance in the real world. For instance, a system leveraged to dynamically adjust health insurance premiums may determine that customers with dogs are more likely to experience heart problems than customers with cats, leading to baseless premium increases for customers who purchase or already have dogs.
- Fallacious interpretation of AI outputs: Even if an AI system perfectly drives, supplements, or assists with decision-making processes, untrained human decision-makers who don’t fully understand AI outputs could misinterpret output data, leading to faulty and potentially harmful decision outcomes.
- Impact on human decision-makers: The prolonged use of AI for decision-making could perpetuate long-term and potentially irreversible skill detriments for human decision-makers. In this respect, recall the earlier points on deskilling, loss of intuition, and critical thinking in the human-AI interaction section.
- Focus on short-term gains: For decisions across domains such as resource management, task allocation, marketing strategy, and product management, decision-making AI could over-optimize in the interest of maximizing short-term gains, inspiring negative externalities like sustainability issues, burnout, reputational damages, and limited product availability.
- Conflicts of interest: AI systems designed by actors with vested interests may orchestrate decision outcomes that overtly favor some stakeholders over others. In this respect, organizations should vet any AI system they plan to leverage for decision-making procedures to ensure fairness, non-discrimination, and output transparency, especially in consideration of emerging compliance requirements.
- Novel or changing environments: As we’ve mentioned before, even the most advanced AI systems struggle with navigating novel or changing environments, which can introduce major challenges for decision-making AI across dynamically oriented complex tasks like crisis management, emergency response, financial planning, investment, and medical diagnosis. These kinds of challenges tend to stem from generalizability limitations rooted in training data, also increasing the risk that decision-making AI fails to account for high-impact edge cases or outliers, such as critical manufacturing defects, when providing recommendations.
- Opaque decision-making criteria: Even if the decision outcome produced by an AI system is correct, the reasons for which the decision is made and the criteria against which such reasons are evaluated may be difficult to interpret and explain, especially for models that leverage deep learning architectures. In high-impact domains like healthcare, finance, hiring and promotion, and law enforcement, those subject to consequential decisions typically have a legal right to understand the nature of the decision made on their behalf, including the motives substantiating it.
Conclusion
Throughout this post, we exhaustively examined numerous lesser-known AI risks, and given the rate at which this technology advances and proliferates, such risks are poised to become more plentiful, widespread, complex, non-obvious, and ultimately, challenging to mitigate and identify. However, fortunately for most organizations, everyone is “in the same boat” so to speak—the AI risk landscape is laden with uncertainty and ambiguity, and those organizations who embrace the challenge of navigating this landscape with diligence, proactivity, resourcefulness, and integrity will be the ones that come out on top.
Nonetheless, to make things more concrete, there are several reasons why proactive risk mitigation—using the AI risk radar to identify and search for risks—is the best approach for any organization with AI interests. While this approach is admittedly more resource-intensive, difficult to implement, and fails to guarantee success, it will, without a doubt, be more effective and resilient than a reactive AI risk management strategy (for the record, there is no way to ensure that all possible AI risks will always be managed appropriately). But, why?
- AI regulations can’t keep up with AI innovations, and they never will because innovation will always move much faster than bureaucracy and safety research. This doesn’t mean that AI regulations won’t emerge or change at an increasingly rapid pace—organizations that proactively identify and manage AI risks will have a comparative advantage in terms of compliance preparedness.
- Innovation creates uncertainty, and in uncertainty there is opportunity. Organizations that possess a deep and contextually specific understanding of the potential evolutionary trajectories of the AI risk landscape will be more equipped to identify and safely capitalize on high-value niches before they become mainstream.
- Organizations with proactive AI risk management strategies will garner respect in the eyes of their customers, employees, and regulators, enhancing their overall trustworthiness, brand visibility, and commitment to responsible AI best practices. In a world where truth and objectivity are easily lost in dense information ecosystems saturated with mis and disinformation, the potential value of such an outcome shouldn’t be underestimated.
- AI integration efforts are permeating virtually all sectors, domains, and industries, which suggests that on a fundamental level, the structure and function of all businesses will undergo a major transformation. Anticipating the direction and scope of this transformation will be far easier for organizations with proactive risk management mindsets since they will have a more informed view of the fine line between AI-driven transformation and disruption.
- Organizations that adopt proactive AI risk management strategies, having a higher level of compliance and risk preparedness than their counterparts, would, in the medium and long term, have more time and resources to devote to enhancing internal AI initiatives, upskilling their workforce, and leveraging AI to streamline internal research and development. Together, these factors could lay the groundwork required for an organization to secure a strong competitive advantage within its given industry.
All in all, proactive AI risk management is the way to go, and even though it may be more difficult, the long-term benefits dramatically outweigh the short-term costs. At the very least, organizations with AI interests should devote considerable time and resources to AI risk management, perhaps even prioritizing this component over others associated with AI integration and deployment practices.
For readers interested in examining further insights into the AI risk management and governance landscape, we invite you to check out Lumenova AI’s blog, where you can also explore content on GenAI and RAI developments.
Alternatively, for readers who wish to design and implement AI governance and risk management protocols, policies, or frameworks, we suggest that you consider Lumenova AI’s RAI platform, and book a product demo today.