August 13, 2024
Existential and Systemic AI Risks: Systemic Risks
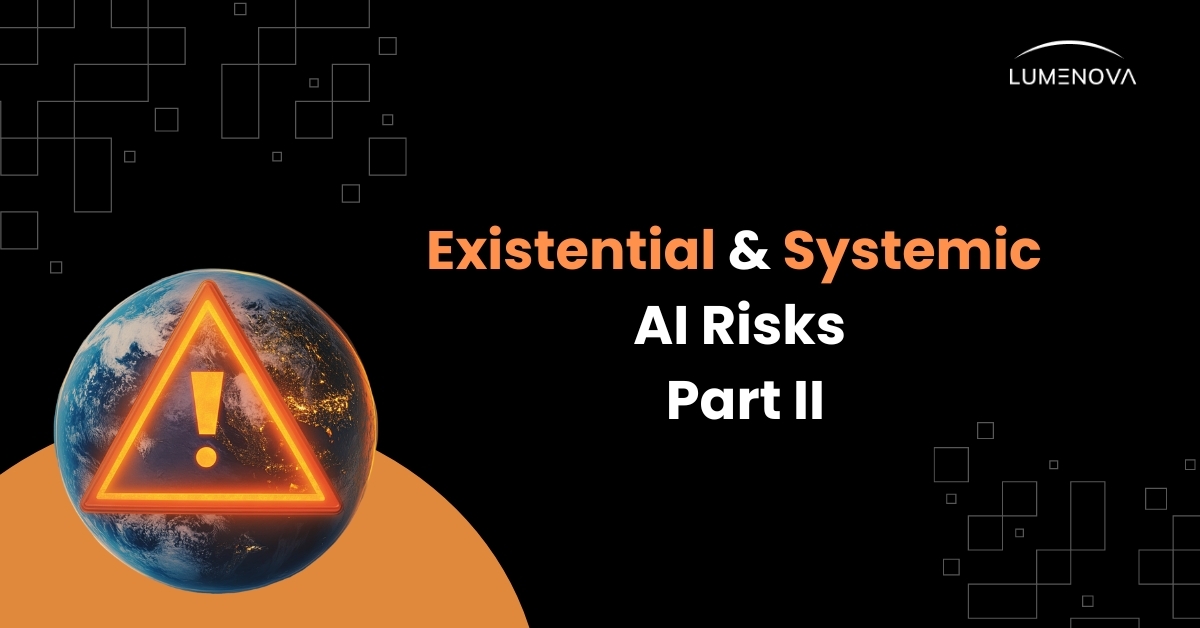
Contents
Systemic AI risks are risk scenarios that compromise the entire functionality of a system, not just its parts. This means that any large-scale sufficiently complex system, whether in the form of an organization or industry like banking, healthcare, education, government, or agriculture, is technically subject to systemic AI risk scenarios. For readers who have not yet been introduced to this topic, we invite you to read the first piece in this series, which offers a brief introduction to the concepts we cover.
Consequently, and for the purposes of clarity, we’ll categorize systemic AI risks broadly, in terms of the systems or industries to which they apply—we’ll begin with societal and democratic risks, followed by organizational and finally, environmental risks. As we reminded readers in our first post, our intention with these discussions is to raise awareness around AI risks, not to cultivate fear or anti-innovation rhetoric among our audience.
Societal and Democratic Risks
From a societal and democratic standpoint, AI systems leveraged in high-impact domains like healthcare, finance, housing, and education could dramatically improve access to and distribution of critical goods and services and/or additional resources that average citizens require to maintain a minimum acceptable standard of well-being and fundamental rights.
When AI systems are used in these kinds of contexts, they can significantly reduce the rate of human error, streamline the consequential decision-making process, optimize critical goods and services distribution in terms of institutional capacity and resource availability, and consider a wider range of socio-cultural and socio-economic variables that would otherwise not be captured in high-impact scenarios, fostering more equitable decision and real-world outcomes.
These kinds of societal AI applications are obviously appealing due to the scalable benefits they inspire, however, their high-impact focus also opens them up to a variety of systemic risks:
-
Inequitable benefits distribution: Even if AI systems successfully optimize resource allocation, the benefits such practices inspire might not be equally distributed among differing societal groups or demographics. AI systems that operate at a population scale may overgeneralize, selectively distributing benefits to majority groups. Alternatively, population subgroups with low AI awareness levels and/or limited access to high-utility AI systems, or conversely, those who have been historically underrepresented by social and legal systems, could be easily overlooked.
-
Cascading failures and systemic feedback loops: Sometimes, a critical system component failure is enough to cause the collapse of an entire system, provided that the failure of the component inspires a failure cascade—as an example, consider the 2008 US housing market crisis, which plunged the global economy into its most severe recession since the Great Depression. In high-stakes systems with many component interdependencies, like finance or healthcare, AI systems leveraged for resource management, access, or acquisition purposes such as algorithmic trading, portfolio management, insurance premium adjustment, and access to medical care could systemically misclassify certain data points or actively discriminate against individuals, groups, or companies due to hidden algorithmic or data biases. The effects of this kind of discrimination or misclassification, if not immediately addressed, could promote systemic feedback loops whereby AI systems reinforce their existing biases with every consequential decision they drive, assist with, or execute, and depending on the frequency, scale, and depth of their operations, system collapse may become imminent.
-
Critical supply chains: AI’s increasing presence and application in supply chains across functions including predictive maintenance and asset management, demand forecasting, inventory and logistics optimization, quality control and risk management, and sustainability, is promising. However, due to the interconnected nature of supply chains and the opaque properties of existing advanced AI systems, several avenues for systemic risk emerge: overreliance on AI-driven predictions and recommendations for resource management, risk forecasting, contingency planning, and crisis management could lead to critical skills degradation, inflexibility in crisis situations, and significant legal accountability concerns. Data integrity, quality, and security issues could propagate rapidly, causing error cascades or feedback loops that result in faulty decision-making, discrimination, and cybersecurity vulnerabilities. Moreover, the integration of advanced AI systems into highly interdependent supply chains could also create single points of failure that are easily exploited by adversarial actors.
-
Unanticipated Interoperability failures and vendor dependency: AI systems can be fine-tuned or modified via post-training enhancements for a narrow task domain or objective. For instance, an agricultural AI that optimizes water irrigation to maximize crop yield could be modified for sustainable building purposes, optimizing energy-efficient urban design by maximizing sustainable resource utilization and simulating energy usage. Seeing as both these models, although they serve vastly different purposes, are built on the same foundational architecture and provided by a sole vendor, if a core component of this architecture were to fail or become compromised via some adversarial attack or data center breach, the models could begin generating highly inaccurate and potentially harmful outputs. At the scale of one farm or city, this might be a manageable problem, but at the level of multiple cities and entire agricultural territories, the consequences would be much more severe.
-
Concentration of power: As we alluded to in the previous section, the most advanced AI models require enormous amounts of data and compute for training and development, which suggests that only a select few companies and organizations will have the financial resources required to continue developing Frontier AI applications. These types of institutions are also disproportionately more likely to subsidize or buy out high-impact or high-value AI start-ups, further concentrating their power and share within the national and/or global AI landscape. If left unchecked, we may find that a handful of companies (and their affiliates) control the course of AI innovation and development, prioritizing their interests over those of society and humanity.
-
Mass manipulation, indoctrination, and coercion: Fallacious AI-generated content like Deepfakes, deliberately misleading news articles, or advertisements have garnered serious attention from lawmakers, ethicists, and AI safety researchers. This kind of content is extremely difficult to identify and authenticate while also proliferating rapidly, permeating virtually every corner of the digital information ecosystem. Moreover, for malicious actors, it’s not particularly difficult to create persuasive or coercive AI-generated disinformation resources and quickly disseminate them at scale via globally interconnected platforms like social media sites (e.g., X), online forums (e.g., Reddit), and community servers (e.g., Discord). This becomes even more problematic when considering how much AI training data is obtained through web scraping methodologies—if dataset validators and curators don’t carefully scrutinize training datasets for accuracy, truthfulness, and representativeness, future iterations of current AI systems may inadvertently perpetuate disinformation feedback loops.
-
Mass surveillance: The opportunities AI affords for surveillance, namely in terms of biometric categorization and identification, inspire a variety of complex threats to democracy and fundamental human rights. Whether in the hands of government actors or large corporations, surveillance tech could be leveraged to systematically undermine democratic structures, fostering invasive practices such as workplace monitoring, social credit scoring, and unchecked surveillance in public places like schools, parks, public squares, and hospitals.
-
Distributed or decentralized AI: Decentralizing AI via methods like open-sourcing, federated learning, and smart contracts could significantly reduce the probability that a concentration of power among a handful of AI actors occurs. However, decentralization also enables malicious actors to exploit advanced AI capabilities to orchestrate scalable, sophisticated, and almost undetectable threats or adversarial attacks that target critical physical and digital infrastructures like the energy grid and stock market, or other high-stakes systems. In this respect, a balance that carefully weighs the benefits and pitfalls of centralized and decentralized approaches is necessary.
-
AI race dynamics: As Frontier AI companies compete with one another to develop and deploy the most advanced AI systems, competitive pressures could favor a “race to the bottom” whereby leading companies consistently cut corners on safety in the interest of maximizing profit and shareholder value. As advanced AI is progressively integrated into a wider variety of high-impact systems like healthcare, agriculture, urban planning, education, law enforcement, and housing, the corresponding breadth of AI vulnerabilities increases substantially, whether in the form of growing attack surface areas that create preventable cybersecurity and data privacy vulnerabilities or alternatively, the active perpetuation of systemic biases in critical decision-making domains. Several other safety issues including the unfettered development of non-cooperative, deceptive, or malicious AI agents could also proceed, introducing yet another array of complex risk dynamics to potential future multi-agent AI systems.
-
Ascended economy: As AI systems become more prevalent in financial markets, serving purposes like algorithmic trading, portfolio management, loan provision and denial, and investment allocation, humans may divulge too much control to them in light of improving autonomous capabilities. This opens up the possibility that financial markets, specifically the flow of financial capital, will be primarily controlled, or at least influenced by autonomous AI systems making high-impact financial decisions on behalf of human overseers. Even if financial AIs are closely monitored by human specialists, this doesn’t eliminate the possibility that humans will be systemically less capable of influencing financial decision outcomes due to an inability to transparently and coherently understand and keep up with high-frequency AI-driven financial decision-making practices.
Organizational AI Risks
Organizations are also systems, and although they tend to operate at a smaller scale than the kinds of systems we’ve discussed thus far, they are still subject to several AI-driven systemic risks, some of which parallel the larger-scale risks we’ve already examined:
-
Catastrophic accidents: Despite their immediate utility in domains like risk management and forecasting, asset management and protection, privacy, and security, widespread AI integration doesn’t make an organization immune to potentially catastrophic incidents like cybersecurity breaches, adversarial attacks, IP theft, supply chain malfunctions, manufacturing failures, or dramatic environmental, organizational, or workforce changes, to name a few possible scenarios. Moreover, seeing as most AI applications leveraged within an organizational context would require fine-tuning for a specific task domain or function, it’s unlikely that such systems would be able to respond to novel or unforeseen risk scenarios—given the resource constraints that most organizations deal with, failure-proofing systems via methods like built-in redundancy, supplementary privacy and security measures, adversarial testing, red-teaming, and failure-mode prediction would be limited if not entirely absent.
-
High-stakes decision-making: High-stakes decisions throughout high-impact industries like finance, education, healthcare, and law enforcement must be accurate, transparent, and truthful, especially when they produce adverse consequences for affected parties. For instance, if a healthcare provider leverages decision-making AI to make real-time adjustments to insurance premiums based on differential data characteristics like race or gender, it may systemically discriminate against certain customers while explicitly favoring others. The same goes for a bank that evaluates creditworthiness for a loan, a university that screens potential applicants for admission, or a law enforcement agency that utilizes predictive policing methods to preemptively mitigate criminal actions.
-
Irreversible organizational interdependencies: Organizations need to be careful about how and where they integrate AI, ensuring that AI integration efforts don’t create organizational interdependencies that can’t be managed by humans in the event of a catastrophic AI failure. For example, if an organization were to fully automate its cybersecurity protocols, a catastrophic AI failure would put its assets, personnel, IP, and reputation at risk, even if a human-in-the-loop is present—concrete protocols for human intervention and incident response must be established and implemented at every level of an organization’s digital infrastructure.
-
Rapid organizational changes or deep transformations: Even the most advanced generalist AI systems aren’t great at responding to novel occurrences or changing environments. When organizations undergo major transformations targeting infrastructure, identity, mission, value proposition, customer base, and/or management, they must ensure that AI integration efforts are aligned with or supported by these kinds of changes—a redefined digital infrastructure might not be interoperable with existing AI applications just as new upper management hires may not care or know about AI risks.
-
Increasing attack surface areas: As organizations integrate disparate AI systems into various departments, the corresponding attack surface area increases—with each AI system that’s integrated, novel failure modes and vulnerabilities emerge, usually in the form of cybersecurity, compliance, adversarial resilience and robustness, data privacy, asset protection, and IP rights.
-
Lack of AI literacy and awareness: Organizations that implement AI without educating their workforce and management on the risks, benefits, impacts, intended use, purpose, and limitations of AI applications put their reputation, security, and continued growth in jeopardy. In some cases, a single misuse of an AI system may be enough to tarnish an organization’s reputation, incur crippling legal fines or sanctions, or create irreversible cybersecurity or asset protection vulnerabilities, whereas, in other situations, misuse could lead to deeply misinformed decision-making based in faulty interpretations of AI outputs, which, if it occurs at the level of upper management, could compromise an entire department or organizational function.
Environmental Risks
Environmental AI applications could play an integral role in facilitating a long-term sustainable future for humanity, with some notable estimates projecting a reduction between 1.5% and 4% in global greenhouse gas emissions by 2030. These kinds of applications can help humans derive novel data-driven or technological solutions to existing climate problems, enhance accountability, transparency, and precision in environmental impact measurement and management, highlight potential sustainable investment areas while streamlining environmental R&D, and ensure equitable and efficient resource distribution and consumption across key industries such as agriculture, manufacturing, and city planning, among several other benefits.
Nonetheless, just as with the other systems we’ve discussed, the integration of AI applications into environmental contexts introduces several noteworthy systemic risk scenarios:
-
Resource allocation bias: AI systems may preferentially redistribute, harvest, or replace certain critical replenishable and non-replenishable resources without due consideration for negative externalities like resource pool depletion. Resource allocation biases can arise due to a variety of factors, including a utility function that over-optimizes for short-term gains, biased or unrepresentative training data, non-transferable use contexts for narrow purpose-built systems, differential error rates across disparate groups, and insufficient human understanding of AI outputs.
-
Energy consumption: Advanced AI systems, in particular Frontier AI models, require enormous amounts of data and compute during training phases. Currently, data centers account for roughly 3% of global energy consumption, whereas annual training costs for the most advanced AI models are projected to increase to approximately one billion dollars by 2027. As the necessity for data and compute continues to increase, despite advances in compute hardware efficiency alongside widespread AI integration in urban areas, scientific research and development, and manufacturing, the possibility that sustained AI innovations will deplete the global energy supply at a non-renewable rate increases substantially.
-
Regime shift vulnerabilities: Natural ecosystems can change dramatically, abruptly, and unpredictably. AI systems trained on historical climate data, even if they’re capable of adaptive learning, may not always be able to account for or predict novel changes or shifts in the environment. For instance, a system used to monitor rising sea levels in coastal urban areas may output predictions that underestimate or overestimate sea levels for the following year—the system may fail to factor in large-scale collective actions aimed at reducing sea level rise, or alternatively, might be trained on data obtained only throughout the last two decades, which points to sea levels rising faster than they ever have before.
-
Preferential environmental investments: AI systems leveraged to understand which areas of environmental concern warrant the highest degree of attention and resources may drive environmental investment decisions that overlook high-risk and potentially catastrophic scenarios. For example, an AI system may determine that due to the frequency and magnitude of earthquakes in California, earthquake preparedness should be the primary environmental concern for Californian lawmakers and city planners. In recent years, however, California has experienced widely varied precipitation levels, which have caused both droughts and severe flooding throughout the state—such trends, due to their statistical inconsistencies, are more difficult to identify and accurately predict, especially in light of climate change, and may therefore be overlooked in favor of managing risky environmental scenarios that are more concrete and consistent.
-
Overcompensation for environmental catastrophes: AI systems utilized for ecological disaster planning and response may overcompensate for certain environmental catastrophes, depleting emergency resources via overallocation. Returning to the previous example, an AI system may, in response to an earthquake in a highly populated urban area, allocate the majority of a state’s emergency food and water supply to affected residents without due consideration for potential droughts or other large-scale environmental events that may occur in the future.
Conclusion
The systemic AI risks we’ve covered in this piece represent several of the most notable risks that readers should be aware of. Still, with each day that passes, advanced AI becomes progressively more embedded into the large-scale complex systems that sustain human society and existence, suggesting that the path toward understanding AI-driven systemic risk scenarios will require continuous learning, interest, curiosity, and investment.
Fortunately, systemic risks tend to be foreseeable, reversible, and most importantly, manageable in light of current state-of-the-art tools, protocols, and methods in addition to ongoing research and regulatory developments aimed at preventing such risks from materializing. In simple terms, even if a systemic risk materializes, its consequences are unlikely to be catastrophic, which can’t be said for existential AI risks—the subject of our next post.
For readers craving more information on the AI risk landscape or other related topics in AI risk management, governance, policy, generative, and responsible AI (RAI), we suggest that you follow Lumenova AI’s blog, where you can track the latest developments across all of these spaces.
Alternatively, for those who’ve already begun exploring or building AI risk management and/or governance frameworks, protocols, or initiatives, we invite you to check out Lumenova’s RAI platform and book a product demo today.
Existential and Systemic AI Risks Series
Existential and Systemic AI Risks: A Brief Introduction
Existential and Systemic AI Risks: Systemic Risks
Existential and Systemic AI Risks: Existential Risks
Existential and Systemic AI Risks: Prevention and Evaluation