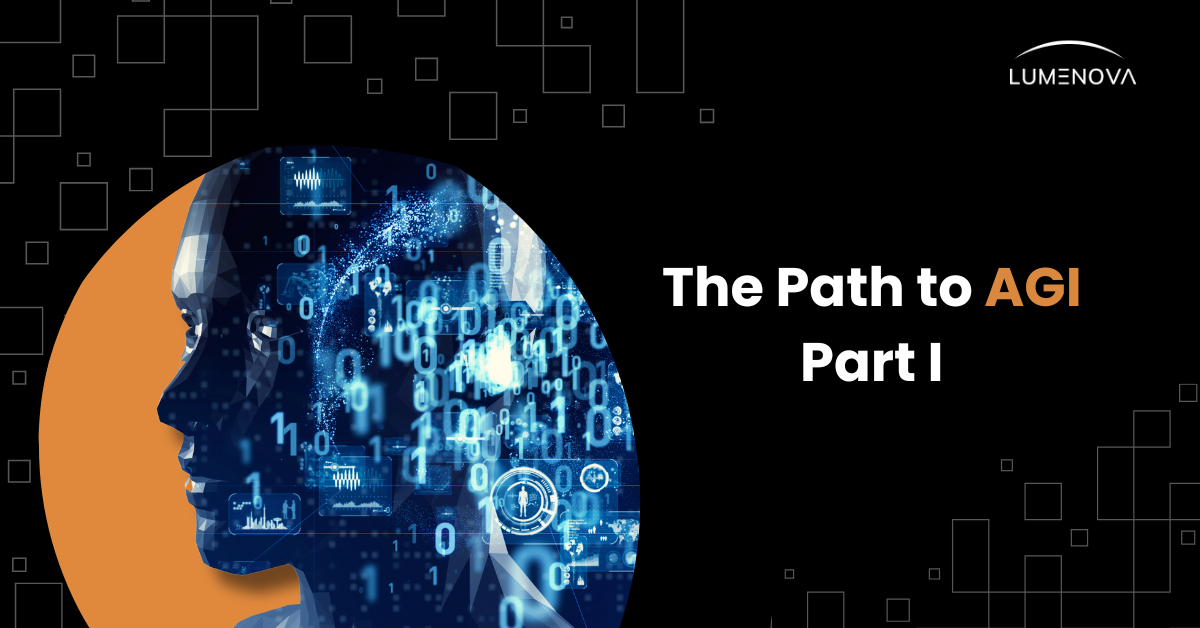
Contents
Current Progress Toward AGI
The path to Artificial General Intelligence (AGI) is rather nebulous. Even if this technology emerges within the next decade—some predict AGI within a few years, while others believe it remains decades away—there’s little guarantee that we’ll know what we’re dealing with. For one, it’s possible that scientific skepticism will preclude us from recognizing early AGI systems for what they are, whereas on the other hand, we might misclassify systems that appear to exhibit properties congruent with our conception of AGI, despite not being “true” AGI.
This brings us to a central problem within the AGI discourse: the lack of a standardized and universal definition of this technology. This problem is unlikely to be conclusively resolved until we interact with and learn from AGI in numerous real-world circumstances. Still, in the meantime, we’ll proceed with the following operating definition:
AGI: Autonomous AI systems with general reasoning, self-improvement, and continuous adaptive learning capabilities that can perform any human task at a level of proficiency that either matches or exceeds average human capabilities across one or several of the intended task domains.
Furthermore, it’s important to note that properties like consciousness, sentience, phenomenological experience, physical embodiment, and emotions might not be essential to the creation of AGI.
While these properties do affect human intelligence, particularly in areas like social understanding and connectedness, empathy and intuitive reasoning, bodily awareness, perception and memory formation, introspection and self-actualization, and self-determination, it’s difficult to say, with certainty, to what degree they affect the average human’s ability to solve a complex problem, plan for uncertainty, or make novel discoveries. Again, the keyword here is “average”—the kind of intelligence we would anticipate in AGI is general, meaning that we shouldn’t necessarily expect early AGI to be a genius at anything (or better than mediocre), especially since the vast majority of humans aren’t.
Moreover, let’s say we did have an AGI that appeared to possess some or all of the properties we’ve just discussed. First, how would we know that the AGI isn’t simply mimicking these properties, feigning an understanding of them that is nonetheless convincing to any human that interacts with it (for reference, see this thought experiment)? Second, and perhaps more interestingly, could the mimicry of these properties be sufficient for boosting the AGI’s intelligence, and could this phenomenon eventually lead to true understanding instead of simple memorization? Finally, can complex reasoning, planning, and problem-solving occur in the absence of these properties?
While we don’t have answers to the first two questions we posed, it could be argued that we’re starting to obtain answers to our final question. In this respect, it’s worth disseminating the key findings of two influential papers published over the last two years, one of which centers on OpenAI’s generalist GPT-4 while the other examines OpenAI’s o1 chain-of-thought advanced reasoning model, both of which hint at early precedents to AGI.
GPT-4 Paper: Key Findings
Capabilities
- Multi-Disciplinary Expertise: GPT-4 is remarkably proficient across multiple disciplines including mathematics, law, coding, medicine, literature, and psychology. Its complex task performance is impressive, exemplified by its abilities to generate code-based visual graphics, build 2D HTML games, create mathematical proofs written in poetic forms, imagine detailed philosophical dialogues, and deconstruct music composition.
- Human-Level Proficiency Across Multiple Domains: While GPT-4 does not achieve human-level performance across all task domains, it matches and even exceeds human capabilities in areas like software engineering, where it made light work of mock exams on LeetCode in roughly 4 minutes, and law, where it passed the multistate Bar exam with an accuracy rate surpassing 70%.
- Linguistic Capabilities and Generalization: Not only can GPT-4 generate sophisticated, coherent, and believable text but it can also modify and internalize certain textual components and ideas, exhibited by its abilities to generate comprehensive summaries, adopt certain communication tones and styles, manipulate and explain complex concepts, and extract various high-level themes from text. In terms of generalization, GPT-4 does show signs of common sense and creative reasoning—it can write and understand jokes and riddles, derive creative solutions to math problems with non-obvious answers, and produce convincing extensions of philosophical arguments.
- Theory of Mind: GPT-4 can pass a modified false-belief task (a psychological test that requires one to adopt the perspective of another), navigate the subtleties of nuanced social and family scenarios it’s presented with, make accurate inferences on the emotional and mental states of others, identify areas of misunderstanding, and comprehend human beliefs and intentions. As a word of caution, these capabilities should not be conflated with the notion of emotional intelligence, of which theory of mind is but a part.
- Discriminative Capabilities: GPT-4 can successfully (although not perfectly) differentiate between disparate ideas, scenarios, and stimuli. Instances of this include the ability to identify personally identifiable information (PII) without any guiding examples and judge the similarity between different statements or solutions while providing potential alternatives.
Limitations
- Architectural Constraints: GPT-4 was trained on a static dataset meaning that its ability to adapt to real-time user feedback and learn dynamically during post-deployment stages is predicated upon exposure to new data and retraining. This should not be confused with GPT’s ability to adapt to individual users during a singular interaction—what is being referenced here is the model’s core knowledge base. GPT-4’s multimodality limitations, while important, are becoming less relevant today as the latest versions of the model gain new multi-modal capabilities like data visualization, image generation, voice, and web search.
- Long-Term Planning & Engagement: Due to constraints arising from its autoregressive architecture—a type of ML model that predicts the next step/datapoint in the sequence based on the steps/datapoints that preceded it—GPT-4 struggles when attempting to plan for multi-turn interactions or maintain sustained coherence and relevance within a long conversation or document. In simple terms, GPT-4 has bad foresight, though the current iterations of the model have demonstrated substantial improvements on this front (note: we are still far away from human-level long-term planning capabilities).
- Reliability & Memory: Beyond its hallucination troubles, GPT-4 lacks an understanding of real-world context and overrelies on training data (once again, this is not a problem unique to GPT-4). These factors can hinder GPT-4’s ability to make sense of dynamic or contextually-motivated real-world interactions whereas in other cases, lead to harmful biases and/or low-quality reasoning in novel, nuanced, or low-resource scenarios and tasks.
o1 Paper: Key Findings
Capabilities
- Logical & Analytical Reasoning: Across domains such as advanced mathematics, medicine and public health, quantitative investment, policy, education, and coding, o1 successfully engages chain-of-thought reasoning processes to solve complex multi-step and multi-layered problems. It can solve college-level math and statistics problems (with moderate consistency), reason by analogy, perform multiple reasoning tasks in tandem, reliably assist with human decision-making, internalize logic-based knowledge from different fields, and most impressively, respond and adapt to novel problem-solving scenarios.
- Cross-Domain Competency & Dynamic Problem-Solving: Throughout a diverse array of highly specialized fields ranging from genetics to anthropology, o1 exhibited knowledge rates that either matched or exceeded those of young professionals and PhD students. Moreover, the model can generate accurate radiology reports, perform medical diagnostic tasks, conduct advanced sentiment and social media analyses, comprehend and plan robot command structures, infer the logic between different elements of natural language, enhance various parts of the writing process, from preparation to editing, create 3D representations of environments, and break down, in a clear step-by-step fashion, stochastic processes in statistics.
- Creativity: While o1’s creative capabilities clearly do not meet human-level creativity, the model did display notable signs of creativity in the context of art education. In one case, o1 was prompted to break down the theoretical concept of currere, providing an explanation that was not only thoughtful and accurate but aligned with the original work on the concept. In a separate case, the model was tasked with developing an art lesson plan for children, which proved to be well-structured, detailed, and coherent. However, the expert human evaluator involved in this case did find that the plan was slightly too structured, failing to provide students with enough creative liberty.
Limitations
- Abstract Reasoning & Long-Term Dependencies: When presented with abstract logical puzzles and non-linear reasoning tasks, o1’s performance dropped significantly, which is unsurprising when considering that all state-of-the-art models still struggle with this. Similarly, tasks that necessitated a prolonged contextual understanding proved difficult for o1, with the model occasionally displaying a tendency to contradict itself as more steps were introduced into the reasoning process. At a higher level, o1 also lacks meta-cognition—the ability to reflect on and assess its own capabilities, limitations, and uncertainties. For the record, GPT-4 shares many if not all of these limitations with o1, although to what appears to be a greater degree in some cases.
- Real-Time Adaptability: Within a singular session, o1 was unable to integrate user feedback and adequately account for the dynamics of fluctuating scenarios. The chain-of-thought reasoning that o1 uses also limits its ability to think creatively or unconventionally—the model tends to perform well when reasoning about a domain-specific problem as a domain expert would, however, where the model is asked to approach a problem from a wholly different angle, performance becomes compromised.
- Specialized Knowledge Gaps & Generalization: Like GPT-4 and many other language models, o1 also produces non-factual outputs and hallucinations, most frequently when it’s tasked with combining information from different fields. This also feeds into o1’s multi-disciplinary weakness, whereby the model doesn’t reliably integrate multi-disciplinary information into a holistic solution. Where certain knowledge niches exist, typically defined by a requirement for deep expertise or access to up-to-date information, o1 can perform inconsistently, though it depends on the niche itself.
- Efficiency & Cost: Like virtually all large language models—including ChatGPT—o1 consumes enormous amounts of energy and compute during training and deployment stages. This inspires relatively obvious concerns for the ability to scale such models and operate them sustainably.
These two papers further highlight an additional set of limitations possessed by both GPT-4 and o1. While both models do represent promising progress toward AGI, they remain deficient in vital properties like self-awareness, intrinsic motivation, and continuous learning, which even superficially, appear to connect with higher-level properties like consciousness, phenomenological experience, intuition, introspection, and perception—we must consider the possibility that these latter properties are essential to AGI, despite the fact that they might not be. We should also point out that the GPT-4 paper was published in April of 2023, and since then, the model has improved significantly, particularly in terms of reliability and memory.
GPT-4’s improvements over the last year further showcase how quickly this technology is moving, especially with the more recent releases of o1, and now, Sora. We have reminded readers of this in many previous pieces—AI is an exponential technology that innovates at a rate that could easily overtake human understanding and control. We aren’t here to “hype” AGI, though we do offer this as a piece of advice to the AGI skeptics who dismiss earlier timeline considerations (our third and final piece in this series will be dedicated to this topic).
Natural Examples of Intelligence
Current general-purpose AI models (e.g., GPT-4o or Claude 3.5) are built using architectures and methods that resemble many of the core neural processes and structures within the human brain. This strongly suggests that humans are predisposed to view the emergence of general intelligence in AI as human-like, which is inherently risky with respect to the assumptions we make about what motivates this intelligence. For example, we might project human intentionality on AGI decisions, attribute emotional motives to the actions an AGI takes, or assume that the emergent objectives an AGI pursues are implicitly aligned with our own.
Consequently, this anthropomorphic bias is one that must be navigated with the utmost care, since at the end of the day, AGI would be a form of non-human intelligence, and during its later stages, likely evolve into super-human intelligence. By contrast, it’s worth entertaining the possibility that AGI will be built by AIs and humans working in tandem, meaning that the final result could be a vision that synthesizes AI and human intelligence, blurring the line between what constitutes intelligence between these fundamentally separate entities.
Therefore, as we think about intelligence in AGI, it may be wise to consider what other non-human forms of intelligence we can classify within the natural world. Viewing intelligence through a non-human lens could help us anticipate how AGI might evolve while also giving us some unconventional tools through which to dismantle our biases and evaluate the capabilities of future AGI systems. Below, we’ve developed a comprehensive list including numerous examples of non-human intelligence ranging across several kinds of species.
Non-Human Intelligences
Primates & Other Mammals
- Empathy in Orangutans: Orangutans have been observed to mimic the facial expressions of others within their group, offer help to group members in harm’s way, resolve conflicts and experience emotions like grief, and display a sophisticated understanding of social roles.
- Tool Use in Capuchin Monkeys: Capuchins can adjust how they use tools based on what they’re being used for, select certain tools by reference to the properties they exhibit, and create tools to solve specific problems.
- Theory of Mind and Self-Control in Chimpanzees: Evidence indicates that chimpanzees could possess theory of mind, highlighted by their ability to infer others’ intentions, knowledge, and goals. They have also demonstrated self-control in reward-based gratification tasks, suggesting the presence of meta-cognitive traits, among others including foresight and episodic memory.
- Emotional Intelligence in Elephants: Elephants console others within their group after experiencing hardship, showcase an understanding of death and mourning, and display some of the most robust social memories of any species within the animal kingdom.
- Cognitive Mapping in Rats: Rats are capable of encoding spatial and object-specific knowledge, navigating complex mazes, retaining and categorizing spatial memories over time, and adapting to fluctuating environments.
- Collaboration in Orcas: Orcas engage in remarkably sophisticated and adaptable cooperative hunting strategies that leverage role specialization, specific communicative vocalizations and visual cues, and hunting tactics that are culturally transmitted across generations.
Reptiles & Marine Life
- Imitation and Differentiation Bearded Dragons: Bearded Dragons have demonstrated the ability to learn via social imitation, an ability previously thought to be exclusive to birds and mammals. These reptiles can also differentiate between novel and familiar environments.
- Tool Use and Problem-Solving in Octopuses: Octopuses are uniquely intelligent creatures capable of performing various complex cognitive tasks like puzzle solving, experimenting with novel objects, adjusting their home environment, and utilizing defensive tools.
- Cognitive Skills in Cleaner Fish: Cleaner fish can prioritize actions that yield delayed rewards, adapt their behaviors in response to changing environments, generalize learned behaviors in novel circumstances, and have even shown hints of self-awareness.
Birds & Insects
- Tool Creation, Use, and Complex Problem-Solving in Crows: Crows can comprehend the functional properties of complex multi-step tasks, utilize tools in sequence and plan several moves ahead, manipulate tools to solve novel problems, and build compound tools using multiple non-functional components.
- Mutualism in Honeyguide Birds: Honeyguide birds have developed mutualistic relationships with human honey harvesters, modifying their guiding behaviors in response to human vocalizations that indicate cooperation, and guiding humans by altering the dynamics of their flight patterns, vocal calls, and perch heights.
- Metacognition and Navigation in Honey Bees: Honey bees can assess uncertainty in decision-making processes, store, categorize, and remember visual cues related to food routes, generalize navigational memories across novel habitats and leverage social signaling (e.g., the “waggle dance”) to communicate food distance, direction, and quality to other bees.
Fungi & Bacteria
- Memory and Problem-Solving in Slime Molds: Slime molds can self-organize and dynamically adapt to solve mazes, modify their strategies according to risk thresholds, optimize resource use by changing their growth patterns, and perform tasks that require spatial, long-term, and short-term memory.
- Distributed Intelligence in Mycelium Networks: Fungi utilize mycelium networks for a variety of purposes including the transmission of information via electrical impulses and the optimization of resource distribution. Mycelium networks can also restructure their architecture to accommodate environmental variations.
- Quorum Sensing and Social Coordination: Quorum sensing is a mechanism that allows bacteria to regulate social interactions and coordinate cooperative acts via molecular signals. This mechanism also allows bacterial colonies to protect themselves against non-cooperators.
These examples, of which there exist a plethora not mentioned here, should allow us to begin dismantling our human-centric concept of intelligence, and look beyond what we think makes an entity “smart.” More subtly, these examples further illustrate the profound diversity of intelligence within the natural world, its incredible malleability, and the vast spectrum on which it can operate. When we think of AGI, especially during its early evolution, we might find it most useful to examine not where its cognitive faculties are aligned with humans’, but precisely where it is that they differ. In this context, we could ask ourselves the following questions:
-
Is building AGI that mimics human reasoning, cognition, and neural architecture the best way to create general intelligence?
-
When environmental stressors are present, does AGI react in ways that are aligned with human behavioral patterns, or do its reactions more closely resemble non-human species’ behaviors?
-
If intelligence exists in all biological systems to some degree, and AGI is aware of the many non-human forms intelligence can take, why would it choose to think like a human?
-
When AGI faces a complex problem or nuanced yet consequential decision, to what degree do the methods and strategies it recruits to solve the problem or justify the decision correspond with processes utilized by different species in the animal kingdom?
-
When an AGI initiates self-improvements that modify its optimization function, does the function more closely favor the AGI’s continued survival, or does it increase the AGI’s alignment with human objectives?
-
Could an AGI, even if it’s designed to serve humans benevolently, eventually learn to prioritize the existence and well-being of non-human species? What factors might motivate this?
-
Would an AGI respond and adapt to environmental changes in the same way that humans do? What if humans were the ones responsible for these changes?
-
Based on examples of super-human intelligence in the natural world, which cognitive capacities might AGI self-improve first? Would humans be capable of understanding this kind of self-improvement?
-
We leave readers with these philosophical questions to ponder, in addition to another big one: if you had complete control over how AGI was designed and for what purpose, what kind of AGI would you create, what behavioral qualities, cognitive and emotional properties, and real-world objectives and tasks would you give it, and what would you prevent it from doing?
Conclusion
In this post, we began with a general discussion and concrete definition of AGI after which we summarized the experimental results of two notable papers evaluating OpenAI’s GPT-4 and o1 models. Next, we described a selection of non-human examples of intelligence, attempting to minimize readers’ anthropomorphic biases while concluding with questions intended to challenge their conception of general intelligence. In our next post on this topic, we will explore the idea of AGI evaluation.
For those wishing to engage with related topics like AI agents and multi-agent systems or existential and systemic risk, we suggest following our blog, where you can find further in-depth resources on well-known domains throughout the AI landscape including risk management and safety, governance, policy, and ethics, generative AI, and AI literacy.
By contrast, for those who are already capitalizing on the responsible AI (RAI) wave and beginning to implement AI governance and risk management procedures, policies, and protocols, we invite you to check out Lumenova’s RAI platform as well as our AI policy analyzer and risk advisor.