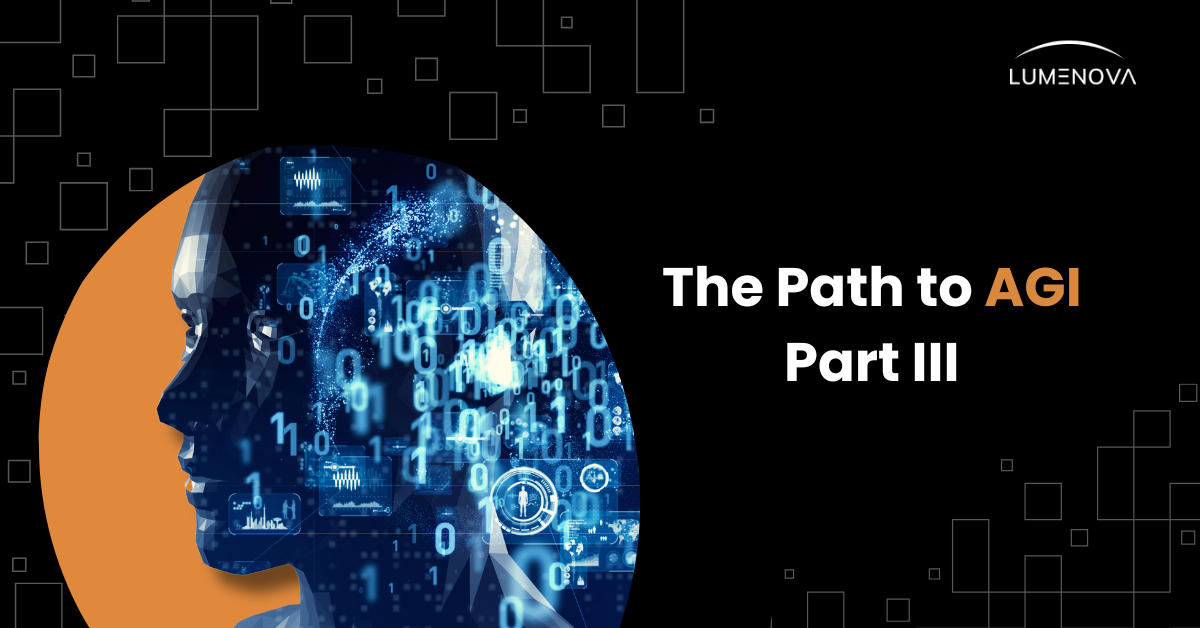
Contents
Note to Readers: In light of recent news, we have shifted the scope of our discussion and redirected our interest toward timeline considerations for AGI deployment (not arrival) and immediate AGI impacts on society. For readers who haven’t read the first two parts of this series, we strongly advise doing so since we’ll return to concepts discussed previously.
In part 1 of this series, we investigated current progress toward AGI followed by examples of non-human intelligence intended to dismantle our anthropomorphic biases. In part 2, we focused on the concept of AGI evaluation, breaking down four distinct evaluation frameworks/benchmarks and providing several bold suggestions for future AGI evaluation and design criteria. In this final segment, we’ll anticipate the forces that may impact AGI deployment timelines and conclude by envisioning the short-term and large-scale impacts of an early AGI arrival.
We’ll begin with a summary and discussion of the potentially revolutionary AGI advancements that have occurred over the last month, critically analyzing them to maintain a grounded and realistic perspective. Next, we’ll operate on the assumption that AGI has arrived, examining the core factors we think will affect AGI deployment. We’ll conclude by exploring a series of notable and immediate consequences we expect AGI will inspire should it become commercially accessible within the next year.
AGI: To Be or Not To Be?
Whether we like it or not, nascent AGI might already be here. On December 20th, the high-compute version of OpenAI’s newest and most advanced model, o3—currently undergoing rigorous pre-deployment safety testing—passed the ARC-AGI-1 benchmark with a score of 88% (the low-compute version scored 76%, four points below the average human score). By contrast, only a few months earlier, OpenAI’s high-compute chain-of-thought reasoning o1 model scored 32%. Frankly, this quantifiable and exponential leap in intelligence capabilities is beyond remarkable.
Nonetheless, while the ARC-AGI-1 benchmark is generally seen as a “gold standard” for AGI evaluation, we should maintain a healthy skepticism regarding AGI’s arrival—o3 still fails at simple human tasks, and ARC claims that the ARC-AGI-2 benchmark could drastically reduce o3’s performance to rates as low as 30%.
Additionally, the cost-per-task discrepancies between the two versions of o3 tested are substantial—high-compute cost-per-task ranged in the thousands of dollars, whereas low-compute cost-per-task was approximately $20. This inspires obvious and pragmatic efficiency and scalable deployment concerns for both the low and high-compute versions of o3, particularly since humans are willing to solve the same tasks for a mere $5. Regardless, we shouldn’t expect these efficiency barriers to linger for long as companies like NVIDIA continue to make steady advancements in super and quantum computing while models like o3 and its predecessors are leveraged to further expedite and improve AI research and development.
More profoundly, while the ARC-AGI assessment purports a unique methodology for comparing human and AI intelligence, its validity as an evaluation benchmark can be reasonably contested according to the core assumptions it makes. First off, the benchmark broadly defines AGI as a system capable of “efficiently acquiring new skills outside of its training data.” Though this definition drives a purer assessment of a system’s general-purpose capabilities by moving away from task-specific performance evaluation, it fails to grasp the full scope of what human and general intelligence might be.
Human intelligence is more than the ability to formulate new skills by operationalizing, synthesizing, transferring, or recontextualizing prior knowledge and experiences—intuition, creativity, foresight, motivation, social learning, cooperation, reflective thinking, self-monitoring, distributed cognition, moral reasoning—many if not all of these properties of our intelligence can be acquired or developed as skills, at least to some degree, by a neurotypical individual.
However, do we honestly have good reason to believe it’s “that simple” when the majority of neurotypical humans, endowed with the same basic set of cognitive faculties from childhood (e.g., intuition of elementary physics and geometry, theory of mind, complex language use, etc.), can live most of if not all their lives without acquiring or meaningfully improving each of these skills, despite the effort they put in? If we agree that humans represent the pinnacle of natural general intelligence on earth, and we know from our individual experience how difficult it can be to acquire new skills efficiently, even when we have access to a wealth of prior knowledge and experiences, why should skill acquisition efficiency serve as the primary maxim of intelligence?
Furthermore, if we are to accurately compare AGI with humans, we can’t dismiss the role that emotions play in our intelligence. Emotions are properties that all neurotypical humans possess from birth, and while they can be honed, improved, redirected, managed, and manipulated, the capacity to exercise them is built into us. Emotion is an undisputable tenet of human intelligence and existence, structuring how we perceive and act on virtually all our experiences. Respectfully, can we be certain that for any of the most brilliant discoveries made throughout human history, from agriculture and democracy to nuclear power and space travel, emotions like curiosity, hope, empathy, envy, and ambition had no role to play? The strength and beauty of human intelligence stem from the complex interplay between our inborn, acquired, and improved qualities—rationality, emotion, morality, creativity, adaptability, lived experience, and relationships, to name several.
Okay, but what if we “lower” the bar and shift our perspective, applying ARC’s view of intelligence to non-human entities? Does it hold up? It doesn’t seem so. Slime molds display emergent optimization behaviors ingrained within their biological structure; ant colonies exhibit collective intelligence that doesn’t arise due to a single entity acquiring or generalizing skills; Caledonian crows manufacture and use tools instinctively; elephants grieve for and console others in their herd; dolphins and magpies can recognize themselves—there are many more such examples.
In nature, intelligence can emerge because of numerous factors including evolutionary instincts, traits, and behaviors, pre-programmed biological structures and/or neuronal architectures, random mutations, innate cognitive and emotional capacities, and swarm-based distributed cognition, among many others.
To be clear, ARC-AGI-1 is by no means a poorly designed or thought-out AGI benchmark evaluation, though it does implicitly assume the following:
-
Humans can efficiently learn new skills from prior knowledge and experiences. This doesn’t correspond with the reality of human existence and experience, particularly at the individual level.
-
The essence of a system’s general intelligence is reducible to efficiently learning new skills outside of its training data. This contradicts examples of non-human intelligence in nature while overlooking innate cognitive and emotional capacities integral to human intelligence.
-
Emotional intelligence isn’t a necessary characteristic of human general intelligence. The creation of value-driven systems like democracy coupled with historic scientific paradigm shifts where scientists pursued truth despite intense doubt and scrutiny (e.g., Copernicus’ heliocentric model of the solar system) seems to disprove this.
-
The best way to measure AGI is by reference to human intelligence. How can we be sure that AGI will think like humans, and if examples of super-human intelligence exist in nature, why would we not include them in our AGI evaluation criteria?
-
General intelligence can be simplified into four essential properties that constitute its foundation. This approach considers neither the functional nor architectural complexity and diversity of human intelligence, let alone non-human biological intelligence, holistically.
-
For highly intelligent entities, intentions should rationally correspond with goals and the processes invoked to achieve them. For agents that can act rationally and irrationally, like humans, this isn’t always true. Intentions can also shift unpredictably and remain outwardly or inwardly covert.
-
Full autonomy, agency, and self-improvement capabilities are not required for AGI. If an AI can’t reliably make decisions, act on them, and correspondingly improve itself—all without human input—assuming it’s generally intelligent, not to mention as intelligent as an octopus or crow, seems like a stretch.
Returning to our original question, should we assume that o3’s performance on ARC-AGI-1 confidently marks the birth of AGI? Notwithstanding our in-depth criticism of this benchmark—which doesn’t reflect its quality or intentions but instead, our attempt to remain grounded—we would argue that, yes, we cautiously should, yet only if the following conditions are met:
-
Assessment of o3 against other available, comprehensive, and high-quality benchmarks and frameworks, including some mentioned in part 2, OpenAI’s MLE-bench, and the Tong Test returns equally if not more impressive results.
-
o3’s score on ARC-AGI-2, to be launched this year, doesn’t exceed a margin 10% below that of the average human score—humans of below-average intelligence are still highly intelligent. A score at or just above 30%, ARC’s low-end estimate, should cause us to reconsider our assumption, though we shouldn’t abandon it.
-
Two non-mutually exclusive options: 1) the cost-per-task of low-compute o3 drops to $5 or less or 2) the cost-per-task of high-compute o3 drops to $70 or less, a rate roughly equivalent to the average hourly rate a PhD graduate makes in the US.
-
The cost-per-task of one or both versions of o3 drops to the above levels within the next 6 months or sooner. Alternatively, cost-per-task for low-compute o3 remains constant or decreases but performance on ARC-AGI-1 nears or exceeds 85%.
-
o3 is subjected to multiple psychological assessments, conducted by a diverse group of expert human psychologists, evaluating theory of mind and emotional intelligence. Performance should come close to or match that of average children between the ages of 3 and 5 on the same assessments.
-
Acceptance that if we are dealing with AGI, it’s the dumbest and most incomplete version of it imaginable. In other words, fetus-AGI, where properties like autonomy, agency, and recursive self-improvement are entirely undeveloped.
AGI Deployment Timelines
If we are to speculate about the factors affecting AGI deployment timelines, we must assume that AGI has arrived, regardless of how immature it might be. From a safety perspective, it may also be wiser to rely on false positives rather than false negatives in this context.
To maintain a non-prescriptive, open-minded, and curious approach, we’ve chosen to frame this discussion by posing questions that may reveal the larger dynamics that govern our current world. This approach should also motivate us to expand our perspective and view the arrival of AGI not only as a national event but as a global one. There are three key questions we ask.
Question 1: Will existing regulation impact AGI deployment?
Robust AI regulation remains largely absent throughout most regions of the world, and where some form of it exists, whether in the US, Canada, or EU, it has yet to be fully tried and tested. Still, if we are to give AI regulation the benefit of the doubt, it would be best to focus on the EU AI Act, since it represents the global regulatory gold standard.
With the EU AI Act, there are two major points of interest: 1) how the act defines and classifies general-purpose AI (GPAI) systems, which it considers to be the most advanced class of AI available, and 2) the role that built-in flexibility and adaptability play in the future of the act’s evolution.
With respect to the first point, the EU AI Act identifies several qualities emblematic of GPAI systems:
- Training on large datasets
- The use of self-supervision at scale
- Generalized learning and task performance
- Integration versatility with downstream applications and systems
Similarly, it describes the following parameters for determining whether such a system poses a systemic risk:
-
Display of high-impact capabilities, measured using available technical tools, standards, and benchmarks or by reference to overall compute, provided that it exceeds a value of 1025 floating point operations (FLOPs).
-
How many parameters the model uses, the size and quality of its dataset, and compute efficiency for training, training time, and energy consumption.
-
The variety of input-output modalities the model possesses and the benchmarks leveraged to evaluate high-impact capabilities across individual modalities.
-
The degrees of autonomy, scalability, adaptability, and access to various tools exhibited by the model.
-
Whether the model is available to >10,000 business users within the EU. This is separate from how many end users the model has.
Here, we already have a problem—if a benchmark like ARC’s becomes the standard for AGI evaluation, the EU AI Act’s definition of GPAI systems could fail to extend to AGI. While related to generalized learning and task performance, task acquisition efficiency is a distinct measure that explicitly aims to quantify general intelligence independent of generalized performance across specific human tasks. This raises issues regarding how European lawmakers refine and improve their GPAI definition to capture AGI. Even if classifying AGI according to performance across core knowledge priors remains the best benchmark, it’s not clear how evaluation criteria and results can be translated into pragmatic and actionable policy.
There is an additional practical issue to consider—every AGI benchmark differs in the assumptions it makes, methods and metrics it uses, and conclusions it draws about general intelligence. If we lack a universal definition of human intelligence and are far from certain as to what constitutes general intelligence—particularly for non-human entities—how could we reliably operationalize an AGI policy definition during the early stages of its evolution? Benchmark criteria must, at minimum, be standardized and agreed upon before policy can sufficiently cover AGI, however, this would also assume that we know what AGI is, which could lead us down yet another worrisome rabbit hole.
Fortunately, the EU AI Act’s GPAI systemic risk criteria, due to its thoughtful balance of broadness with specificity, should be able to account for AGI systems, particularly since they are virtually guaranteed to surpass the 1025 FLOPs compute threshold. Furthermore, the act’s inherent flexibility and adaptability, bolstered by the EU Commission’s AI Office and scientific panel, should enable fairly swift remediation of the act’s provisions if AGI enters the market.
At the global scale, there are no regulatory guardrails for AGI deployment, which is unsurprising in view of the default anarchic state of international politics. That being said, we see two possible governance options here: 1) the creation of a global alliance on AGI that can hold individual nations accountable for the impacts of AGI deployment (akin to an organization like NATO or the UN), or 2) the US president, via executive order, issues a temporary ban or moratorium on AGI deployment and/or development. This second point is qualified by the assumption that the US will maintain an unobstructed lead in AI innovation and that foreign powers will fail to replicate the technology, cementing the US as the global epicenter and ultimate controller of AGI.
In a nutshell, regulations’ influence on AGI deployment could range from marginal to extreme, and the degree of influence will be heavily mediated by the actions (or lack thereof) taken by powerful international bodies like the US, EU, and China. By contrast, a reinvigoration of the global effort to maintain and likely redefine the bedrock of human and civil rights will need to be taken, which alone, serves as a colossal task.
Question 2: How might socio-economic incentives and goals affect AGI deployment?
Within markets, there is a clear incentive to deploy AGI due to its historically unparalleled automation and augmentation potential. Even in its most immature form, AGI could revolutionize how we collaborate, innovate, adapt, grow, and lead within professional environments. Employee turnover, lack of managerial oversight, language, communication, and cultural barriers, poor employee training and upskilling, cybersecurity, and optimization of multiple business processes—many of the factors that frequently hinder professional productivity and efficiency would weaken significantly or disappear entirely once AGI is integrated.
However, this incentive is predicated upon two beliefs, the first of which is that scalable AGI deployment is both cost and energy-efficient—all for-profit companies, irrespective of their missions and values, must prioritize profit-making, otherwise they will fail sooner or later. This brings us to a faulty assumption businesses could make when considering AGI integration: automation and augmentation potential outweigh AGI’s efficiency costs. The fact is if companies like OpenAI can’t build AGI systems that are as cost-efficient per task as the average human worker across some domain, large-scale deployment will remain practically unfeasible in the short-term.
The second belief comes from base human psychology, namely our tendency to anthropomorphize. Businesses could implicitly assume that an AGI system will a) perform tasks and reach objectives by invoking understandable human-like thought processes, tools, and methods, b) freely and consistently cooperate with humans and other AIs, c) only pursue the goals and objectives prescribed to it by humans, d) make far fewer mistakes than humans do, and e) some combination of all these assumptions.
To adopt a hopeful perspective, let’s presuppose most businesses can understand the nature of the assumptions driving the incentive behind their AGI adoption efforts, and let’s say AGI does reach an acceptable level of cost-efficiency. Could we expect businesses to readily and quickly deploy it? To put it bluntly, no. The full array of reasons for this answer is nuanced and complex—we hope to tackle this discussion in the future—however, four key points stand out:
-
As a worker, regardless of skillset and qualifications, AGI represents a profound threat to professional livelihood. Even if companies assure their employees that AGI will be restricted to an augmentative force, it’s difficult to envision how most workers, when faced with a technology that can do their job just as well if not better than they can, will respond positively to it. We expect that AGI’s arrival in the workforce will be anything but welcome.
-
AGI integration would necessitate a radical restructuring of any organization, where leadership, mission, values, business objectives and processes, and workflow operations are redefined from the ground up. Whether AGI is deployed in the form of AI agents, a singular operating system, or a combination of the two, a paradigm shift in how humans work is inevitable. Moreover, anticipating the after-effects of this paradigm shift would prove extremely challenging since history doesn’t offer any examples of automation that parallel machines that can think and perform as humans do.
-
Industry and sector-specific business and regulatory requirements will muddy the scope of AGI integration. This isn’t to say that such requirements will target AGI specifically—chances are they won’t—but they will have a bearing on the impacts that AGI can inspire for how an organization runs. For example, imagine how hard it would be to grapple with the problem of what AGI could mean for HR operations, especially in terms of employee motivation and satisfaction.
-
To quell automation fears among their workforce and showcase commitment to a human-centric future, more companies than expected will resist AGI integration. We shouldn’t blindly assume that all companies, or even a majority, will accept AGI, despite how naive or counterintuitive this seems. The business landscape is often condemned as ruthless, yet there are numerous companies that have maintained their integrity and adhered to core values, like employee-centric operations, since their inception.
Question 3: What role could international pressures play in AGI deployment?
While we won’t spend much time on it, we have asked this question last for a reason: international pressures, characterized as AI race dynamics, are situated as the most powerful group of incentives driving AGI deployment on a global playing field. Put simply, the first nation that acquires and implements AGI will be in a position of power comparable to that of the first nation that obtained nuclear weapons. We don’t believe this is an exaggeration, and if anything, it might be a modest comparison, particularly as AGI evolves.
The benefits AGI cultivates for national security and defense, surveillance of foreign adversaries, novel weapons manufacturing, critical infrastructure management and optimization, resource utilization and sustainability, governance, bureaucracy, and policy-making, market growth and expansion, and the rate of scientific discovery and innovation barely scratches the surface of what this technology could do for nations as a whole. These potential benefits wouldn’t only allow a nation to guarantee an arguably insurmountable adversarial and defensive advantage over all other nations but also begin rebuilding the fabric of modern human civilization in their image, authoring the future of humanity.
This process of rebuilding, whether it goes well or poorly—relative to the nation and its people, values, and culture—will be the “AGI experiment” whose results the rest of the world eagerly anticipates. However, to assume that other nations will passively wait until the first stage of the experiment is completed would be foolish. As soon as one nation has AGI, all other nations who perceive they have the resources and power to try and steal and replicate the technology before its potential is realized will engage in a full-fledged and highly aggressive effort to do so, whether it’s overt or covert.
Perhaps the more insightful and daunting question to ask here is this: will the first nation that has AGI be able to deploy and operationalize it before powerful adversaries steal and replicate it and/or instigate a war?
Final Timeline Considerations and Impact Predictions
Taking into account the three questions we’ve illustrated and the incentive factors they’ve revealed, it’s evident that even humble predictions regarding immediate AGI deployment timelines and impacts will be remotely accurate at best. Still, in our spirit of boldness, we leave readers with the following predictions, divided into two categories: deployment and impacts.
Deployment Predictions
-
Large-scale AGI deployment throughout the business sector and workforce will follow the validated emergence of true AGI by a minimum of 6 months or more in whatever nation has AGI first.
-
The first AGIs deployed within the workforce will take the form of collaborative AI agents. These agents will be fine-tuned for specific human task domains though they will easily and quickly adapt to any other tasks they are asked to perform.
-
The first AGIs will be deployed within controlled operational settings that operate as sandbox environments within a finite selection of government-picked organizations, private or public. These deployment initiatives will remain classified until national security concerns are addressed.
-
Societal-scale protests will erupt on national scales upon the first public AGI deployment attempts. The issues that these protests signify will play a notable role in the design and implementation of future AI policies concerning the nature of human work and civil rights.
-
In Western nations, AI regulation will affect AGI deployment insofar as current AI policies are amended before AGI models finalize pre-deployment safety testing procedures or executive orders placing a temporary ban/moratorium on AGI deployment are issued.
-
Global governance will not affect early AGI deployment efforts. If a global AGI council is created for international AGI oversight, accountability, and development, this will happen posthumously, within one year or more following the first national-scale AGI deployments.
-
The nation that leads AI research and development will not be the first nation to deploy AGI. Foreign adversaries will discover how to steal and replicate this technology before it’s widely available in its birth country, and they will make all efforts to deploy it as quickly as they can at home.
-
If both authoritarian and democratic regimes possess what they believe is true AGI, authoritarian regimes will be the first to deploy it. Authoritarian AGIs will have two initial objectives: surveillance (international and domestic) and military operations.
-
International conflicts will disrupt early AGI deployment efforts and increase AI race dynamics, setting the stage for a global array of rushed AGI deployments that result in a slew of negative externalities at the national level.
-
Within the first 6 months of AGI deployment, we’ll witness the emergence of relationship AIs—AGI systems that are explicitly designed to assume the role of “friends” or “romantic partners. Immature versions of this phenomenon have already been documented.
Impacts
-
Many of the first AGI deployments in the workforce will fail, not as a consequence of the technology itself, but because of the failure to anticipate that AGI integration will not transform but reinvent and resculpt business processes.
-
Even if businesses adequately prepare for the potential structural and operational impacts of AGI integration, employees will struggle to work in tandem with these systems. It will take time for people to learn how to engage with AGI as a genuine collaborator rather than an advanced tool.
-
The entire notion of technology will be brought into question. Historically, technologies have served as tools—a means to an end—that enabled humans to solve bigger and bigger problems. AGI won’t just be something we use, but something we work with and alongside.
-
The average citizen will not care about or comprehend the significance of AGI’s arrival. For the majority of people, AGI simply isn’t something they spend time thinking about, and most humans haven’t witnessed a scientific discovery of this magnitude in their lifetimes, lacking any frame of reference with which to make sense of it.
-
AGI benchmarks will prove almost comically uninformative in evaluating AGI capabilities over time, especially during the early stages of its evolution. Models will improve so fast that our benchmarks will quickly become obsolete—we also continue to insist that the best way to measure AGI is against human intelligence, which a) we don’t universally understand, and b) implies that AGI will invoke human-like thought processes.
-
Early AGI will become the most important tool for designing and orchestrating AGI benchmark evaluations. Just as humans have developed tests to evaluate human intelligence, AGI will do the same.
-
The first commercial users of AGI will be scientists and researchers who are granted early access to these systems within closed operational environments. In these contexts, AGI will be used to facilitate research, experimental design and hypothesis generation, and testing and analysis.
-
Some commercial AGI systems, particularly once they are replicated, will be hijacked and weaponized by bad actors and/or foreign powers. They will be used to manipulate, coerce, and extort both individuals and groups en masse.
Conclusion
Throughout this piece, we’ve discussed 1) the nature of ARC’s AGI benchmark, the assumptions it makes, and their bearing on our assumption regarding the arrival of AGI, 2) the large-scale factors influencing AGI deployment timelines, conceptualized through three key questions on governance and regulation, socio-economic incentives, and international pressures, and 3) two sets of predictions on the dynamics of AGI deployment timelines and immediate AGI impacts. With this, we wrap our AGI series, though we do expect to return to this topic soon.
For those interested in exploring related topics including AI agents, multi-agent systems, and existential and systemic risk, we suggest following Lumenova’s blog. Here, you’ll also be able to access a wide range of resources covering broader topics within the responsible AI (RAI) space including governance, risk management, ethics and safety, AI literacy, and generative AI.
On the other hand, if you crave tangible guidance and tools for AI governance and risk management, we encourage you to consider booking a demo of Lumenova’s RAI platform and/or exploring our AI risk advisor and policy analyzer.