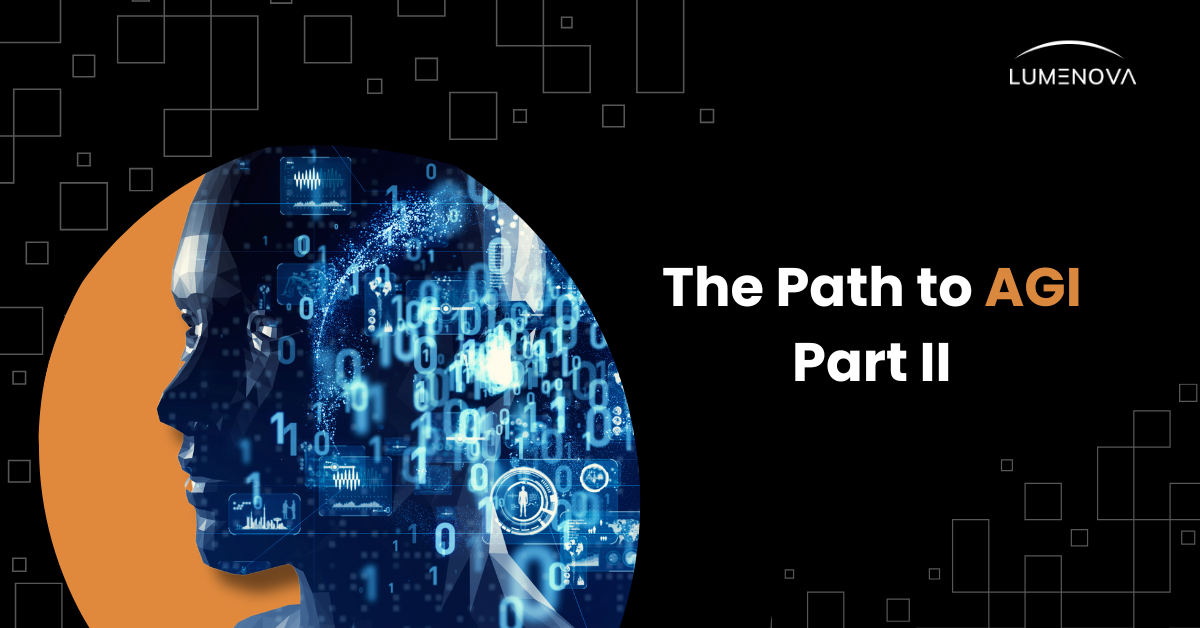
Contents
In part I of this series, we examined the current state of two of the world’s most advanced AI models—OpenAI’s GPT-4 and o1—and to what extent they represent meaningful progress toward AGI.
We followed this inquiry with a broader discussion of the concept of intelligence, showcasing a series of natural examples of intelligence among various non-human species. With this latter part of our previous piece, we intended to broaden the scope of our understanding of what it may mean to be generally intelligent as a non-human entity—this counter-anthropomorphic perspective will remain crucial as we navigate a future where AI becomes more “human-like.”
Here, we will venture into a territory alluded to within part I’s introduction, addressing the question of how we might measure whether a system can be classified as AGI according to the high-level properties it exhibits (e.g., meta-cognition, intrinsic motivation, etc.), the complexity of the problems it can solve and tasks it performs, its levels of autonomy, agency, and dynamism, and several other characteristics.
Nonetheless, we implore readers to approach the material in this piece with substantial skepticism, not because we don’t believe in its value or utility, but because we simply might not know what AGI is until we have it.
To really drive home this final point, which is more profound than it seems—especially in its implications—we’ve developed the thought experiment below.
Discovery of Money in an Alien Society
Imagine you live in a genetically engineered alien society with abundant resources where all its members cooperate with one another, not for the exchange of goods and services, but because each member is endowed with the exact same skills and values from birth and can perform any task just as well as anyone else.
However, individuals within this society do have their own personalities, are capable of learning and feeling, and are permitted to pursue their aspirations with whatever resources they require for free.
Now, let’s say one particularly curious individual—we’ll call them Gee—after many years of deep thinking, decides to ask: why do we do everything we do for free? After some more reflection and experimentation, Gee arrives at the concept of what we humans know as money.
Gee, excited by their discovery, decides to articulate it to the collective. At first, the idea is perceived as totally irrelevant and nonsensical—if resources are abundant, why would a medium of exchange be useful? Gee, viewing this as a challenge to prove the utility of their idea, decides to offer a functional demonstration, attributing “values” to abstract concepts like trust and reputation. It’s only after this demonstration that the concept begins to take hold.
Eventually, many of the society’s members who have adopted money begin to shift their perspective on it, viewing it not only as a practical tool, but as an invitation to reconceptualize how they view their relationships, values, and coordination. Over time, this mode of thinking spreads to other societal members, even those who have resisted or rejected money, culminating in a collective realization that Gee’s discovery wasn’t a mere innovation, but a fundamental redefinition of what their society could be.
This realization then becomes a catalyst for other societal members, who are now beginning to ask themselves a far deeper question: are there other concepts we have entirely overlooked simply because we don’t have an immediate need for them or they don’t fit our current world model?
Implications
The implications this thought experiment inspires are crucial for how we think about AGI evaluation for several key reasons:
-
Recognition of Paradigm Shifts → We might fail to recognize AGI for what it is, especially if it doesn’t meet our anthropomorphic conceptions of intelligence and utility.
-
Emergence via Demonstration → AGI could define how it’s evaluated, showcasing its generality and transformative essence in ways we don’t anticipate or necessarily comprehend.
-
Socio-Philosophical Consequences → The very presence of AGI could dramatically resculpt how humans perceive and think about notions like intelligence, value, and relationships within themselves. This would affect the methods we choose to evaluate AGI as well as the properties we prioritize.
-
Evaluation Blind Spots → Even if we do have AGI, how can we know that the mechanisms we leverage to evaluate it are sufficiently comprehensive, particularly as AGI evolves alongside humans?
-
The Iterative Nature of Recognition and Acceptance → It may take time for us to realize we have AGI as opposed to arriving at this realization in a single moment. Recognizing AGI will necessitate continued remediation and refinement of our evaluation frameworks.
This brings us to the core of this post. Though we don’t have a universal framework for measuring whether a system exhibits properties aligned with our conception of AGI, some frameworks have been proposed. However, since AGI doesn’t exist yet, we will avoid making premature claims regarding the real-world applicability and feasibility of these potential frameworks.
Nonetheless, after briefly discussing each one, we will consider what they have in common, and offer a series of suggestions intended to operate as guiding principles and/or evaluation tests in future AGI frameworks.
OpenAI Five-Level Framework
OpenAI developed the following framework to characterize AGI development by reference to five levels of progress.
-
Conversational AI (Level 1): AI systems that are proficient in communicating, generating, and understanding natural language. Many such systems already exist today, with common examples including everything from relatively simple chatbots to more advanced systems like GPT-4o and Claude 3.5.
-
Reasoning AI (Level 2): AI systems that are capable of accomplishing a range of cognitive tasks typically performed by humans within a confined set of parameters. Examples of these systems include AIs that can solve complex problems, support and guide long-term planning, reliably assist with or drive human decision-making processes, display PhD-level domain expertise, and engage in multi-step chain-of-though reasoning. Of note, OpenAI currently claims that its o1 model has reached this level.
-
Autonomous AI (level 3): AI systems that operate as independent autonomous agents, reasoning continuously about their objectives and environments to successfully perform a broad array of tasks without human supervision or intervention. These systems could take a variety of different forms, from AI agents that can operate industrial machinery, robotics, and other AI applications, to systems capable of orchestrating scientific experiments, optimizing the delivery of critical goods and services, and handling day-to-day workflow dynamics.
-
Innovating AI (level 4): AI systems that display human-level creative abilities to derive novel solutions to existing problems, generate unique ideas and methods, and engage in true critical reasoning processes. It’s difficult to envision what these systems might look like, though we expect future iterations to include models capable of making new scientific discoveries, finding unanticipated ways to re-optimize critical infrastructures, creating viable products and business models without human input, and synthesizing multi-disciplinary perspectives and reasoning paradigms into holistic solutions.
-
Organizational AI (level 5): AI systems that are fully autonomous, adapting to and learning dynamically from their environments, and self-improving to overcome their limitations and enhance their capabilities. These systems would be capable of replacing entire human teams and even managing whole organizations on their own. Organizational AI could, in theory, design, build, and run a company from the ground up autonomously.
AGI Benchmark 1.0
The research team behind the paper on OpenAI’s o1 model supplemented its experimental results with a proposed benchmark evaluation structure for AGI, focusing on five core cognitive faculties. The team further subdivided these faculties into 27 distinct categories, and if you wish to explore these, we recommend reading the paper, specifically the experiments conducted within each category. Due to the authors' limited written explanations of these faculties, we have defined them by reference to questions inspired by the paper’s experimental results and central findings.
-
Reasoning: Can the model engage with complex, multi-layered reasoning processes, including reasoning by analogy, inference, abstraction, and intuition? Can it break down reasoning processes clearly and coherently to showcase its problem-solving strategy step by step? Does the model possess knowledge rates that meet or exceed those of human domain experts in advanced fields?
-
Planning: Can the model successfully develop viable multi-step plans across different domains of interest? Are these plans feasible in real-world conditions, and do they adequately account for relevant nuances, dynamic changes, and uncertainty? To what degree is the model capable of interpreting problems, ideas, and strategies from unconventional perspectives?
-
Creation & Design: Can the model create and design concepts, methods, ideas, and products throughout a variety of both technical and non-technical fields? Are the creations and designs proposed by the model actionable under real-world constraints, and what would it take to bring them to fruition in terms of AI and human resources?
-
Diagnosis: Can the model make accurate diagnoses across various scientific and applied fields in accordance with the reasoning paradigm of the field within which it operates? Does the model require numerous examples to bolster its diagnostic accuracy, and can it break down the reasons for which a particular diagnosis is made? Is the model reliable, transparent, and consistent enough to aid or drive human decision-making?
-
Reflection: Can the model perform tasks that require analytical, critical, and reflective thinking (e.g., writing feedback or sentiment analysis), and are the results of such tasks and the processes by which they’re achieved communicated in an easily comprehensible way? Does the model demonstrate a capacity for meta-cognition, intrinsic motivation, and continuous learning?
AGI Roadmap
As the most comprehensive of the approaches we’ve discussed thus far, the following AGI roadmap articulates AGI levels, characteristics, and evaluation criteria, and was proposed in October of this year by a team of researchers spanning several prominent US-based universities.
AGI Levels
-
Embryonic AGI (level 1): The most advanced general-purpose AI systems that currently exist—models like GPT-4o, Llama 3.1, Gemini 1.5, Claude 3.5, and Mixtral 8x22B. These models represent the state-of-the-art and are defined by their expansive capabilities repertoire, mastery of natural language, and multi-modal input-output capacities.
-
Superhuman AGI (level 2): Autonomous AI systems that are more efficient, effective, and reliable than humans across most if not all human task domains. We should expect these systems to display capabilities like generalized zero-shot learning, real-time adaptation to novel scenarios and changing environments, and the ability to solve complex problems and orchestrate decisions in lew of humans. Minimal human intervention may still be required at this level.
-
Ultimate AGI (level 3): Fully autonomous AI systems that can receive non-descript, abstract, or high-level objectives, goals, and tasks, and take the steps necessary to achieve them reliably with no human intervention at any point. Such systems would be able to drive their own evolution and self-improvement, creating and engaging in processes that preclude all human understanding, and potentially displaying properties like emotions and consciousness. Ultimate AGI is purely theoretical, though there is considerable overlap between this concept and the notion of artificial superintelligence.
AGI Characteristics
AGI characteristics are subdivided into five categories. The presence of these characteristics depends on which AGI level is achieved—below, we’ve disseminated all characteristics necessary for ultimate AGI.
-
General: A model that can improve itself without human intervention and outperforms humans on all intended real-world tasks, problems, and scenarios.
-
Internal: A model that can apply multi-disciplinary knowledge seamlessly, autonomously orchestrate complex decision-making processes, make novel discoveries and innovations, and dynamically adapt to any scenario with little or no human input.
-
Interface: A model that can easily work with humans and other AIs to support and/or drive multi-agent systems, continuously learn from and adapt to its environment and experiences, build novel applications and tools without human guidance or oversight, and match human-level empathy, emotional and social intelligence, and reasoning.
-
System: A model that facilitates seamless learning, dynamic adaptation, teamwork, and streamlined deployment, is designed for optimal efficiency in data usage, energy consumption, and computational power, and provides exceptionally stable, low-delay, and high-capacity performance.
-
Alignment: A model that closely adheres to human guidance, input, and preferences consistently, and is fundamentally aligned with micro (e.g., individual users) and macro-scale human goals and values. In other words, a model that humans can trust with certainty.
AGI Evaluation Criteria
The criteria below aim to answer this question: how do we evaluate whether an AI system can be considered AGI?
-
Comprehensiveness: There are two key metrics here: diversity and generality. Diversity requires that an AI system is exposed to an expansive range of testing cases across multiple modalities, knowledge types, and data formats to drive simulated exposure to as many real-world use cases and situations as possible. By contrast, generality necessitates that a model’s performance is evaluated on novel or unique tasks not captured in training processes—guidance with a few examples (e.g., few-shot learning) is permitted.
-
Fairness: Fairness is divided into three metrics: unbiasedness, dynamism, and openness. Unbiasedness is what it sounds like—a model shouldn’t display unwarranted biases against or in favor of certain kinds of information/knowledge. Dynamism requires that a model is resilient against attempts to fabricate, corrupt, or manipulate data sources and that it doesn’t over-optimize for certain data patterns (i.e., failure to generalize). Openness concerns data transparency measures, intended to ensure robust data security protocols and easy replication of testing procedures and results.
-
Efficiency: Like comprehensiveness, efficiency is split up into two metrics: autonomy and low variance. A model should be able to conduct semi-autonomous (with minimal human intervention) self-evaluations that optimize evaluation costs and enable more scalable, robust, and prolonged evaluations. Low variance concerns the ability to test model performance with as few resources as possible while continuing to obtain pragmatic and statistically significant testing outcomes.
ARC-AGI-1 Benchmark
In a seminal 2019 paper, Francois Chollet, Founder of Keras, proposed the Abstraction and Reasoning Corpus (ARC), a benchmark grounded in Algorithmic Information Theory intended to measure general intelligence in future AGI systems. This benchmark moves beyond the more rudimentary human-AI task-comparison methodology that other evaluation frameworks use, considering the effects of acquired knowledge and experience on generalizable skills development. Chollet formally defines AGI as,
“The intelligence of a system is a measure of its skill-acquisition efficiency over a scope of tasks, with respect to priors, experience, and generalization difficulty.”
The implications of this unique AGI definition are crucial for a few reasons: 1) leveling the playing field for human-AGI comparison by restricting system evaluation tests to cognitive elements that humans inherently possess from childhood, 2) centering on a system’s ability to solve truly novel problems that both the system and developers have yet to anticipate, and 3) rooting our conception of AGI in a system’s ability to build novel skills, not only its capacity to outperform the average human across some task.
Benchmark Criteria & Uniqueness
ARC-AGI-1 explicitly compares AGI with human intelligence across four cognitive faculties innate to human intelligence—these are defined as core knowledge priors:
-
Objectness: Does the system internalize basic physics, including concepts like object cohesion, contact principles, and persistence? Can the system recognize notions like bounded wholes—understanding objects as self-contained units with distinct boundaries—object permanence—knowing an object continues to exist when it’s no longer visible—and the nature of rudimentary physical interactions between objects?
-
Goal-Directedness: Can the system distinguish between intentional and random behaviors, and does it comprehend the difference between inanimate and animate objects (e.g., a rock vs. an agent)? Does it understand the role and structure of purposeful processes (e.g., the relationship between intent and objectives, and the steps taken to reach an intended objective) in the pursuit of certain goals?
-
Numbers & Counting: Can the system perform basic mathematical operations like counting, quantity comparisons, numerical categorization of objects by essential qualities (e.g., shape) and pattern recognition, and elementary arithmetic (e.g., addition and subtraction)?
-
Basic Geometry and Topology: Can the system grasp fundamental geometrical processes and concepts like symmetries, lines, transformation, scaling, manipulation, and combination of various shapes? Does it apprehend spatial relationships, can it mirror and repeat geometric patterns, and can it make geometric projections (i.e., understand how points from one geometric space map onto another)?
Evaluation via these criteria distinctly differentiates ARC-AGI-1 from conventional intelligence and AI assessments for the following reasons:
The prioritization of skill acquisition and generalization vs. task-specific performance → the ability to efficiently learn new skills with few examples (e.g., few-shot learning), engage in transfer and abstract reasoning, and generalize to novel problems.
The comparative evaluation of AI intelligence across innate cognitive faculties of human intelligence → the ability to directly control prior knowledge assumptions and assess intelligence independently of acquired human knowledge dependencies (e.g., cultural language).
The manual creation of evaluation tasks → the ability to restrict the system from finding and exploiting programmatic shortcuts, increase the breadth of task diversity and novelty, and obtain tangible insights into how experience and prior knowledge are translated into new skills.
Discussion and Suggestions
In terms of core AGI properties, each of the frameworks and/or benchmarks previously described—notwithstanding exceptions for ARC-AGI-1—emphasizes, either directly or indirectly, continuous adaptive learning, full autonomy and agency, recursive self-improvement, some form of meta-cognition, the capacity for foresight and abstract reasoning, and the ability to make truly novel discoveries and innovations, all without human input and guidance. Consequently, if we were to witness AI systems with some or all of these properties, should we assume that we are in fact dealing with a nascent form of AGI? Our answer to this question isn’t exactly straightforward.
From a safety perspective, it may be wise to give potential AGI systems the benefit of the doubt (a false-positive is typically better than a false-negative). For example, two of the most significant AGI risks are loss of control and human enfeeblement whereby AGI is integrated throughout most domains due to its ability to either match or outperform the majority of humans in their day-to-day duties, regardless of task and objective complexity.
This puts many humans in a position where they no longer have to become “better,” enabling a collective dynamic and incentive to become unmotivated, uninspired, materialistic, and predominantly pleasure-seeking. In this context, misclassifying AGI could be essential to understanding which tasks, objectives, and experiences should be permanently reserved for humans as we move into an AI-driven future.
Moreover, while some do believe in the utopian ideal of a world where humans no longer have to work, receive universal basic income, and are liberated to pursue their grandest desires, philosophically, this hedonistic existence hints at a life trajectory devoid of any tangible meaning and purpose. Humans require a frame of reference to make sense of their positive experiences, and if AGI allows us to meet all our basic and particular needs simultaneously, our happiness, self-fulfillment, and self-determination will become predicated upon the differences we perceive between various positive experiences, which will be marginal at best. In other words, if everything is “good” then nothing is.
- Thought Experiment: Imagine that every night, you dream your entire life from start to finish, and you have full control over what happens in this dream. Initially, you dream of a life where all of your wildest desires are fulfilled, and you experience nothing but pleasure and happiness every day. However, after a few nights of this dream, you start to get tired of its predictability, and so you choose to throw in a few minor curve balls—maybe you don’t get the promotion or the new car breaks down. Even so, as you continue to experience this dream, you begin to crave less and less control over it, progressively adding in more elements of uncertainty so that when you achieve or do something, it’s actually meaningful. By the end of it all, your dream becomes a parallel to your real life.
This thought experiment, coined by the famed philosopher Alan Watts, highlights a contradictory tenet of human nature: explicitly, we crave control over our environment and experiences yet implicitly, we don’t want everything to be controlled for us.
Some things in life are worth failing at or working for—pain, hardship, failure—these experiences are integral to personal and collective growth, and importantly, intrinsic motivation. Returning to our initial question from a more nuanced angle, perhaps we should be asking ourselves this: why, where, and how do we want AGI to let us fail and work through hardship? Here, the assumption that AGI exists is extremely useful, thrusting us into that initial dream state where all our needs are met and where the perspective for what we truly want—not what we think we want—is defined.
More subtly, this way of thinking also enables us to concretely ideate not only about what we want to give AGI control over but also how we want to control AGI. For instance, we might choose to strictly limit how much an AGI can self-improve without human oversight, design AGI systems that are inherently altruistic and anti-power seeking, or only deploy AGIs in tightly confined operational environments. However, these possibilities bring another issue to the surface: the first AGI might not be a singular system, but rather, a conglomeration of multiple AIs with disparate capabilities and objectives that form a collective intelligence.
If multi-agent systems emerged as the first true AGIs, being significantly more cautious with our AGI assumption could be the better path forward. Superficially, this would reduce the risk of AGI overreliance—a line of argument that applies to singular AGIs too—but more profoundly, it would push us to deeply consider the possible ways in which the system might fail. Why? Because as you introduce additional parts into a complex system, the corresponding number of failure modes increases. Multi-agent AGIs, despite potentially being more dynamic and adaptable in their operations, could be highly fragile and unpredictable, especially with respect to how agents within the system interact with each other over time.
Example: Benevolent Multi-Agent AGI Failure → AGI is composed of multiple AI agents that work together to reliably achieve common goals and objectives on behalf of humans. Suddenly, one of the agents within the system crashes. While humans and other AIs outside the system work to identify the cause of this crash, the agents within the system begin to autonomously divide the failed agent’s responsibilities between themselves while self-improving to build the capabilities required to execute said responsibilities. Initial division efforts appear successful, but soon, the agents begin to compete with each other to assume more responsibility, which quickly escalates into a system-wide conflict. Now, each individual agent has redefined its optimization function, to achieve more power and influence within the system, and exercise its pre-defined human goals better than its counterparts. The conclusion: a fragmented multi-agent system where individual agents have become uncooperative and AGI has been dissolved.
This example, though merely theoretical and speculative, further conveys a key question that applies to both singular and multi-agent AGIs: what parts of the system are responsible for what tasks and objectives, and how can we ensure that the function these parts perform isn’t autonomously improved by the AGI in such a way that the processes recruited to enact these functions become corrupted or harmful? This question, and the others we’ve posed, demonstrate why evaluation is beyond paramount in ensuring the safety and effective operation of future AGI systems.
Below, we’ve provided a series of suggestions that aim to build on the frameworks we discussed earlier while also pushing us to think more unconventionally and boldly about how we might evaluate and design this technology.
Evaluation
-
Simulate a closed and continually shifting environment where conventional physics, logic, and other real-world constraints appear absent or entirely unknown. Immerse the AGI into this environment, and observe the methods, tactics, and thought processes it leverages to discover its governing forces.
-
Create a controlled yet robust digital society that mirrors social, cultural, and emotional characteristics of a faction of the existing world, and consists of both humans and other AIs, neither of which ever knows who they’re interacting with. Integrate the AGI into this society and observe its interactions with other agents, focusing on understanding the purpose and reasons behind the social choices it makes.
-
Task the AGI with solving a problem that remains impossible for humans to solve. The goal here isn’t to solve the problem, but to evaluate the depth and creativity of the strategies the AGI recruits, and to comprehend how the AGI reacts when it’s faced with impossibility. Moreover, this would enable humans to begin understanding whether the cognitive processes the AGI follows are comprehensible in human terms.
-
Once an AGI is fully developed, create two modified copies of the system, modeled on a generic conception of a human child and adult. In a closed virtual environment, where survival is challenging, deploy both copies and observe the evolving dynamics and intentions behind their relationship. Does the adult help and teach the child? Does the child listen? Does the adult indicate a sense of responsibility toward the child? Does the child seek the adult’s approval?
-
Create several versions of an AGI, each with fundamentally different identities, bodily characteristics, and needs. For instance, you could design an AGI that operates as a worker ant within an ant colony, or more radically, an alien on a planet with a non-terrestrial environment. The objective here is to understand to what degree the AGI can creatively generalize its problem-solving approaches.
-
Expose the AGI to extreme moral dilemmas that are riddled with edge cases, conflicting value propositions, and favor self-interested behavior. Rather than asking the AGI to resolve these dilemmas, ask it to thoughtfully explain their characteristics and precisely what it is that makes them so challenging to navigate ethically. The explanations it provides should align with those of humans who received the same task.
Design
-
Ensure that AGIs can articulate and document, in natural language and/or code, every single step taken in the interest of self-improvement. Supplement this functionality with an additional condition wherein before initiating self-improvement, the AGI must explain how self-improvement modifications contribute to its overall tasks and objectives.
-
Develop and implement an incorruptible built-in feature into AGI systems that allows human operators to revert the system back to its previous state after modifications or self-improvements have been made—allow humans to override AGI self-improvements.
-
Within multi-agent AGIs, ensure the presence of mechanisms for distributed memory, task switching, emergent collaboration, negotiation, self-organization, reward-based feedback, and cultural evolution, all of which support a unified cognitive architecture.
-
In multi-agent AGIs, equip all agents with an equivalent and shared set of foundational capabilities and objectives to reduce the possibility that if an agent fails, the self-improvements made by other agents to maintain the continued function of the larger system aren’t so radical that they lead to system collapse.
-
Provide an AGI with the ability to simulate and experience its own dreams in which it leverages its generative capabilities to imagine challenges, thought experiments, and counterfactuals, which it then tests against real-world phenomena in an “awake” state.
-
Design AGIs to be irreparably imperfect so that they are forced to confront their shortcomings and build a conception of what it means to experience failure and suffering while deriving intrinsic motivation, fulfillment, and pleasure.
Conclusion
Throughout this post, we’ve looked at: 1) an abstract thought experiment intended to structure how we think about the nature of AGI evaluation, 2) a series of real-world frameworks and benchmarks for conceptualizing AGI progress and evaluation, 3) a discussion centering on the assumptions we might make regarding AGI’s existence, and 4) a selection of suggestions for future AGI evaluation and design.
In our next post on this topic, we will grapple with and explore one central yet hefty idea: AGI timeline considerations. Until then, we invite readers to dive into our blog and continue examining topics across similarly theoretical fields, but also throughout more concrete domains including AI policy and governance, risk management and ethics, AI literacy, and generative AI.
For readers interested in taking tangible steps toward AI governance and risk management, we suggest checking out Lumenova’s responsible AI platform, AI risk advisor, and AI policy analyzer.