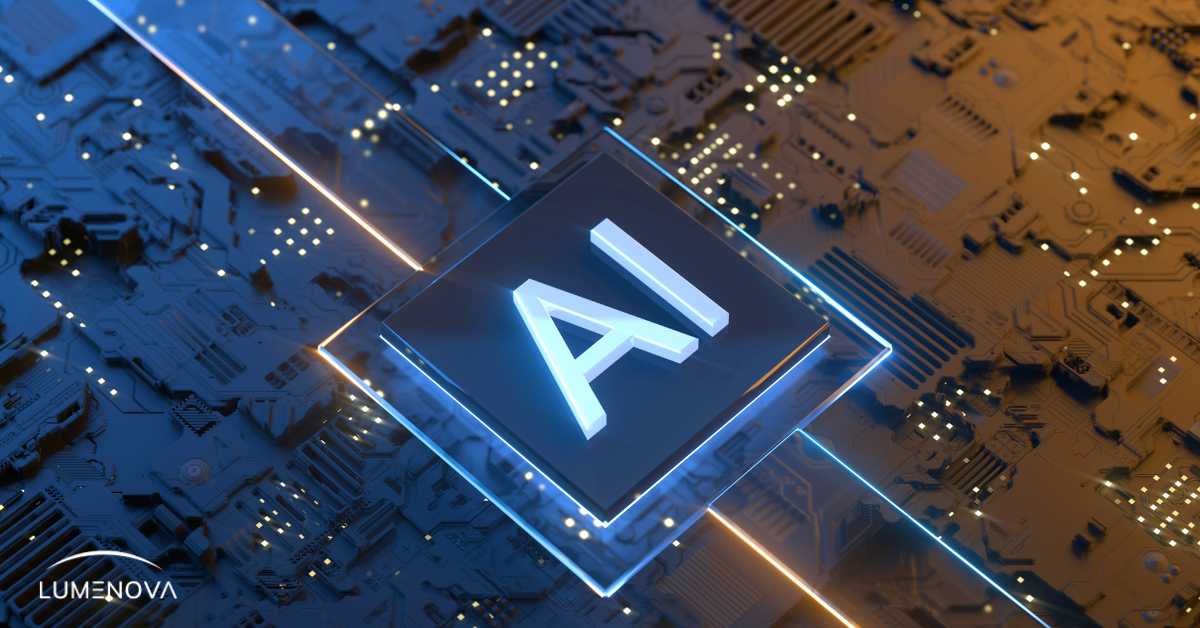
Contents
Slowly but surely, AI models are penetrating each and every layer of business. Their advantages? Many. From hiring the right candidates and approving credit applications, to making online product recommendations, and empowering transformation across the healthcare industry, AI with its superhuman capabilities is, for certain, a business success driver.
Making black box AI responsible
Behind AI models, machine learning algorithms work toward offering the most accurate and relevant predictions. However, increasing a model’s complexity is often required to achieve the best results. As a consequence, the decision-making process is often hidden behind the AI’s black box.
As previously discussed, this lack of algorithmic transparency and understanding gives rise to concerns regarding AI bias and fairness and ultimately translates into a slow adoption rate. As such, the need for accountability arises, both from a human and legal perspective.
Thankfully, there’s a solution that addresses the need for knowledge and clarity: Responsible AI.
The advantages of Responsible AI
Responsible AI can help businesses open the black box and gain insights into what’s driving the decision-making process. Concerns about fairness, transparency, model reliability, and compliance can all be addressed by employing Responsible AI tools.
Since AI models have been known to produce unfair and biased decisions in many instances, Responsible AI can help to build trust and cement stakeholder confidence.
AI Explainability
Gaining an understanding of the “how” and “why” behind an AI’s black box decision is essential for a user to fully adopt and trust the model. It’s only natural to expect people to ask for explanations, especially when faced with unexpected events or results that violate their existing mental model.
This being said, Explainable AI (XAI) facilitates the adoption process by creating a shared understanding between the AI model and the human. It allows you to see how constant an AI model is in its decision-making process and assess the global and local feature impact.
💡 A local explanation enables you to see how the model arrived at a particular prediction. For example, “this particular person will probability pay back the loan because of his high income and excellent credit score”.
💡 A global explanation gives you insight into the model representations of the world. It gives you a sense of how the model “thinks” in general. For example, “people with higher income tend to pay back loans; people with low credit scores tend to default”.
AI Fairness
Another manner in which Responsible AI works for your business is by helping detect predictions that are potentially unfair towards certain population groups. Often enough, AI models unintentionally uphold discriminatory practices due to the biased nature of the training data.
The case of Amazon’s biased recruitment tool which turned out to be sexist against women is well-known. This happened because their AI model was trained on resumes that reflected the male dominance across the tech industry. Naturally, its predictions turned out to be biased as well.
Responsible AI tools work against undesirable outcomes such as these, by presenting the potential biases embedded in the system. Based on insights such as data impartiality, demographic parity, equality of odds, and equality of opportunity, Responsible AI tools facilitate the efficient removal of bias from your model.
There is much to be discussed when it comes to fairness in Machine Learning. However, making sure that AI models do not work in unjustified or biased ways is essential, and can be achieved by the strategic implementation of Responsible AI.
AI Reliability
Only by understanding how your AI model works and why it makes the predictions it does, can you fine-tune it to achieve maximum performance.
Take product recommendations, for example. We receive them every day. But without understanding your model’s behavior, how can you really tell if your algorithm is reliable when it comes to choosing products well-tailored to consumer needs?
By opening the black box of AI, you can understand if some features have an unreasonably high impact on your model’s prediction relative to others and determine if this is, in fact, a valid behavior or just some spurious correlation which the model picked up during training.
Responsible AI creates a window into a model’s predictive process and allows you to take the best pathway toward enhanced efficiency.
Compliance and auditability
As policymakers have started introducing legislation regarding the use of AI, organizations must open up their black box AI in order to ensure compliance with industry standards and emerging regulations.
Here are just a few to keep an eye out for:
💡 UE’s General Data Protection Regulation (GDPR) states that the automated processing of individuals must be done in a way that ‘safeguards the data subject’s rights and freedoms and legitimate interests’.
💡 The California Consumer Privacy Act (CCPA) of 2020 dictates that users must be informed about how their data is being used by AI models.
💡 The Algorithmic Accountability Act of 2022 if passed, will require companies to create transparency about when and how automated systems are used, empowering consumers to make informed decisions regarding the use of AI.
💡 New York City’s Local Law 144 requires employers to undertake yearly bias audits if they employ AI and algorithm-based technologies for recruiting, hiring, or employee promotion purposes.
Key takeaways
It is now, perhaps more important than ever, to employ Responsible AI tools and techniques in order to meet the ethical and legal requirements pertaining to AI.
Businesses around the world will need to open the black box to ensure transparent and fair AI interactions.
Lumenova AI is an end-to-end platform that takes your ML model from black box to Responsible AI, so you can:
- Ensure a fair and bias-free decision-making process
- Enhance model performance, reliability, and robustness
- Meet existing and upcoming regulatory requirements
To schedule a demo, please get in touch with our experts today.