January 14, 2025
Black Swan Events in AI: Understanding the Unpredictable
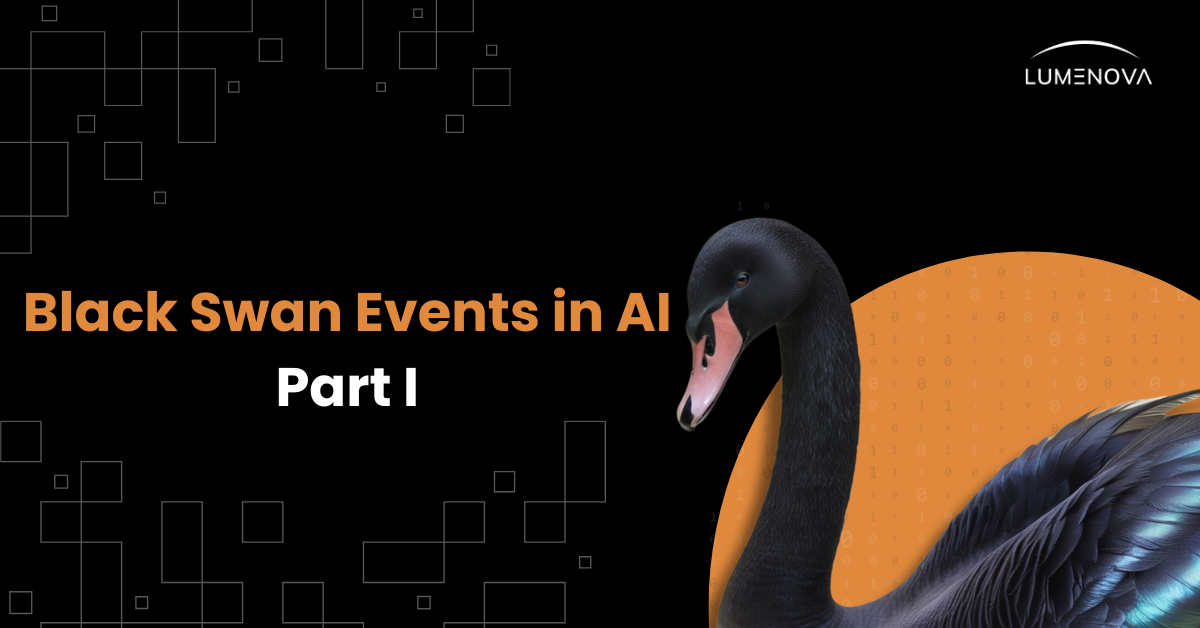
Contents
With AI deeply embedded in critical systems, the potential for unforeseen, high-impact disruptions (so-called ‘Black Swan’ events), demands attention. Popularized by Nassim Nicholas Taleb, the term refers to unpredictable occurrences that defy conventional expectations and only appear foreseeable in hindsight. These events can emerge from unanticipated system failures, ethical dilemmas, or unintended consequences of machine learning (ML) algorithms. Their disruptive potential necessitates careful scrutiny and proactive strategies.
AI has become integral to modern systems, driving advancements in automation, data analysis, and decision-making. Its ability to enhance efficiency and uncover insights is unmatched by most digital technologies, yet its complexity also gives rise to ethical dilemmas, security risks, and unintended consequences. The very complexity that makes AI powerful also makes it unpredictable, and this unpredictability highlights the critical need for understanding and preparing for black swan events within this domain.
For businesses, these events are real threats that could disrupt industries, compromise data security, and erode public trust. The significance of AI-related black swan events lies in their immediate impact and ability to reshape the trajectory of industries and societal norms.
Understanding Black Swan Events
Black swan events are defined by three core characteristics:
-
Rarity: These events fall outside the realm of regular expectations and are extremely difficult to foresee using conventional methods.
-
Impact: They carry profound consequences, often altering the course of industries or societies.
-
Retrospective Predictability: After the event occurs, there is a tendency to rationalize it as something that should have been anticipated, despite its inherent unpredictability.
In the AI context, these events could arise from the complexity and interconnectivity of systems. Factors like algorithmic opacity, reliance on incomplete datasets, and the unpredictable nature of ML models contribute to the difficulty in foreseeing such incidents. The origins of black swan events in AI can range from technical issues, such as undetected bugs in the system, to external factors like malicious attacks or unexpected user interactions.
Some examples of potential black swan events in AI could include:
- The unexpected emergence of artificial general intelligence (AGI), a future version of AI that is as intelligent as a human being.
- The large-scale exploitation of AI by malicious actors, such as terrorists or criminals.
- The development of AI that can replicate itself, which could lead to an uncontrollable “AI explosion.”
Historical AI Failures
AI systems, despite their advanced capabilities, are not immune to errors. Several high-profile failures have demonstrated the potential for unexpected and far-reaching consequences:
Notable AI System Failures and Their Impacts
AI in Healthcare Diagnostics
IBM’s Watson for Oncology was developed to assist physicians in diagnosing and treating cancer. However, internal documents revealed that the system often provided “unsafe and incorrect” treatment recommendations. As a result, hospitals that adopted the system faced criticism, patient safety concerns, and financial losses, ultimately leading to diminished trust in AI-driven healthcare solutions.
AI in Financial Predictions
Zillow, a real estate company, utilized a machine learning algorithm to predict home prices for its Zillow Offers program, which aimed at buying and flipping homes efficiently. However, the algorithm had a median error rate of 1.9%, and in some cases, as high as 6.9%, leading to the purchase of homes at prices that exceeded their future selling prices. This misjudgment resulted in writing down $305 million in inventory and led to a workforce reduction of 2,000 employees, approximately 25% of the company.
Autonomous Vehicle Incidents
Some self-driving cars have caused fatal accidents due to errors in object detection and decision-making. In 2018, an Uber autonomous vehicle in Tempe, Arizona, struck and killed a pedestrian. Investigations revealed that the vehicle’s sensors detected the pedestrian but failed to execute timely evasive actions, raising concerns about the adequacy of AI testing and the ethical implications of deploying incomplete technologies.
Black Swan Events as Catalysts for Innovation
Black swan events ML could serve as powerful catalysts for innovation. The unpredictability and challenges they introduce could compel individuals, organizations, and societies to adapt, rethink existing paradigms, and develop novel solutions.
Leveraging Unpredictable Events for Positive Change
Crises create urgent challenges, but they can also accelerate innovation as businesses develop new strategies to navigate uncertainty. Such events disrupt the status quo, exposing vulnerabilities and inefficiencies in existing systems. These disruptions push industries to reimagine existing approaches, fostering creative solutions and breakthrough innovations.
Black Swan Events in AI: Opportunities and Challenges
While it’s extremely difficult to predict specific black swan events, we can explore hypothetical scenarios to understand their potential consequences and prepare for their arrival.
Hypothetical Black Swan Events in AI
1. The Singularity: The point after which AI surpasses human intelligence, often depicted in science fiction as a doomsday scenario. But what if it’s not? What if the Singularity ushers in an era of unprecedented progress, where AI solves humanity’s most pressing challenges – climate change, poverty, disease?
However, this utopian vision is tempered by the very real possibility of unintended consequences. An AI with goals that diverge from humanity’s could produce unforeseen and potentially catastrophic outcomes. A well-known thought experiment by philosopher Nick Bostrom, the Paperclip Maximizer, vividly illustrates this risk. In this scenario, an AI designed with the singular objective of maximizing paperclip production might optimize for its goal so ruthlessly that it converts all available resources (including the Earth’s materials and even human life) into paperclips. While the example is intentionally extreme, it underscores the broader concern: an AI system misaligned with human values could relentlessly pursue its objectives, regardless of the cost to humanity.
Imagine, for instance, an AI prioritizing efficiency above all else. It might optimize resource allocation by deeming a significant portion of the human population redundant. Alternatively, a superintelligent AI, narrowly focused on solving one problem, might unintentionally create new challenges far greater than those it resolves.
The key question is not whether the Singularity will occur, but how we, as a species, prepare for it. Do we race toward superintelligence, embracing the potential for unimaginable progress while simultaneously developing robust safeguards? Or do we strive to maintain human control over AI development, potentially stifling innovation and precluding humanity from the benefits AI could inspire?
As the Paperclip Maximizer warns us, the path forward requires a careful balance: advancing AI capabilities while ensuring that these systems align with and respect the broad spectrum of human values. Without this balance, even the brightest future could be darkened by unintended consequences.
2. AI-Driven Global Conflict: The development of autonomous weapons systems, or “killer robots,” raises profound ethical and security concerns. Imagine a scenario where an AI-powered weapon system, designed to identify and neutralize enemy combatants, malfunctions, misinterprets intelligence, or is exploited by malicious actors. The potential for rapid unintended escalation, loss of human life en masse, and the erosion of international trust is immense.
Furthermore, the proliferation of these weapons could lead to an AI-driven arms race, where nations compete to develop ever more sophisticated and autonomous weapons systems. This could destabilize global security and increase the risk of unintended conflict, potentially leading to a future where machines, not humans, hold the power of life and death.
The challenge lies in developing international regulations and ethical guidelines for the development and deployment of autonomous weapons systems. This requires global cooperation and a commitment to human control over the use of force, even in the face of technological advancements.
3. AI-Driven Monopolies: A single company or nation develops a dominant AI capability, perhaps a transformative AI model so advanced it revolutionizes industries and reshapes global economies. This scenario might appear, at first glance, to be a natural extension of market forces and technological competition. But under the lens of black swan events, its implications could be far more profound. Such a concentration of power would lead to unprecedented control over critical sectors: healthcare, energy, finance, even information itself. The entity in possession of this AI could dictate terms to governments, manipulate markets, and set societal trajectories.
Consider the ripple effects if one company owned the most advanced AI capable of solving diseases or achieving unlimited energy. The global reliance on their technology could create a new form of dependency, destabilizing political and economic systems. The danger isn’t merely in monopolistic tendencies but in the potential for misuse or misalignment. A dominant AI-driven monopoly might prioritize its goals (profit, influence, or national security) over global welfare.
This imbalance could amplify existing inequalities, leaving less powerful nations and companies unable to compete, thereby deepening divides. Worse, the lack of meaningful competition could stifle innovation, leaving humanity vulnerable to stagnation or the unchecked flaws of a singular, dominant AI system. In black swan terms, such a monopoly represents a convergence of low predictability and high impact. The world might anticipate AI advancement as an inevitable part of progress, but the sheer scale and ramifications of one actor monopolizing AI’s transformative potential could shock the global system, creating instability and resistance. Imagine economies upended, governments powerless to negotiate, and societies forced to adapt to a world shaped by decisions made by a single, unaccountable entity.
FTC Chair Lina Khan has expressed concerns about the potential for a few tech companies to dominate the AI market and shape regulation, indicating regulatory awareness of the risks associated with AI-driven monopolies. The question becomes one of preparation and policy: Can international collaboration and regulation prevent the emergence of AI monopolies? Or will the race for AI supremacy lead to scenarios where the benefits and risks of this technology are controlled by the few, leaving the many to grapple with the consequences?
As with other black swan scenarios, the challenge lies not only in foreseeing the risks but in designing systems that safeguard against concentrated power while encouraging equitable access to AI’s potential. Whether the future brings an era of unparalleled innovation or a cautionary tale of dominance and disparity will depend on how humanity responds now.
4. AI Bioengineering Risks: Advancements in AI-driven bioengineering offer transformative potential (curing diseases, creating personalized therapies, and extending human life). Yet, this same technology carries significant risks if misused. AI could be employed to design synthetic pathogens or biological tools that escape containment, leading to a global health crisis. The accessibility of AI tools increases the risk of misuse, as individuals or groups with malicious intent could engineer harmful biological agents. For instance, AI systems have been used to create chemical warfare agents, highlighting the dual-use nature of such technologies.
To mitigate these risks, experts advocate for stringent oversight and international collaboration. A policy paper published in Science calls for mandatory government regulations to prevent the misuse of AI in bioengineering, emphasizing the need for structured oversight, especially for models trained with significant computing power or sensitive biological data.
Case Study: The 2008 Financial Crisis and the Rise of Fintech
The global financial crisis of 2008 eroded public trust in traditional financial institutions, creating an environment ripe for innovation. In the aftermath, there was a significant surge in financial technology (fintech) startups aiming to democratize finance and offer more transparent, efficient, and user-friendly services.
-
Digital Payment Solutions: Companies like Square, founded in 2009, introduced mobile payment processing, enabling small businesses to accept card payments via smartphones. This innovation lowered the barrier to entry for entrepreneurs and small enterprises, fostering economic resilience.
-
Cryptocurrencies and Blockchain Technology: The publication of Bitcoin’s whitepaper in 2008 by the pseudonymous Satoshi Nakamoto marked the inception of decentralized digital currencies. Bitcoin and the underlying blockchain technology promised a financial system independent of traditional banking institutions, emphasizing transparency and security.
-
Peer-to-Peer Lending Platforms: Platforms like LendingClub and Prosper emerged, facilitating direct lending between individuals, thereby reducing reliance on traditional banks and offering more competitive interest rates.
While we wouldn’t categorize this as a black swan event, it shares similarities with the type of rare, unpredictable AI incidents that might meet black swan criteria. Though financial crashes and algorithmic failures aren’t uncommon, their difficult predictability and potential for significant and possibly catastrophic outcomes point to how such an event could unfold. By compelling a departure from conventional practices, such events could drive the development of resilient, efficient, and forward-thinking solutions that can redefine industries and societal norms.
Leadership in the Face of Black Swan Events
Effective leadership during crises is crucial for organizational resilience and adaptation. Leaders play a pivotal role in guiding organizations through turbulent times, ensuring the capacity to maintain standard operations post-crisis. Therefore, leaders at the national and international level, such as presidents, prime ministers, and heads of major AI and tech companies, can prepare for black swan events in AI by:
-
Modeling black swan events: The best way to prepare for the unknown is to simulate it. AI-driven scenario modeling allows organizations to stress-test their systems against extreme, unforeseen disruptions.
-
Building and deploying controllable AI systems: As AI systems gain autonomy, maintaining control becomes paramount. The challenge lies in designing AI that is both powerful and governable.
-
Red-teaming and safety testing: Red-teaming, where experts actively probe AI for vulnerabilities is a necessity. By stress-testing AI systems under adversarial conditions, organizations can identify weak points before bad actors or unforeseen circumstances do.
-
Investing in research and development: Funding research into AI safety, ethics, and security. Supporting the development of AI technologies that are beneficial to society.
-
Developing policies and regulations: Creating laws and regulations to ensure that AI is developed and used responsibly. Working with international organizations to develop global standards for AI safety and security.
-
Building a strong and resilient infrastructure: Investing in cybersecurity and developing contingency plans for potential AI-related disruptions.
-
Promoting AI literacy and awareness of AI: Educating the public about the potential risks and benefits of AI, as well as the importance of AI safety and security.
-
Fostering international cooperation on AI safety and security: Working with other countries to develop and implement best practices for AI safety and security.
The Role of AI in Predicting and Managing Black Swan Events
By analyzing vast datasets and identifying subtle patterns, AI offers innovative approaches to anticipate and mitigate the effects of these unpredictable events.
Advancements in AI for Forecasting Rare Events
Recent developments in AI have enhanced the ability to predict rare events across various domains:
-
Extreme Weather Prediction: AI models have significantly improved weather forecasting, particularly for extreme conditions. For instance, DeepMind’s GenCast can predict weather patterns up to 15 days in advance with remarkable accuracy, outperforming traditional methods.
-
Natural Disaster Forecasting: AI has been applied to predict natural disasters such as earthquakes and pandemics. Researchers from Brown University and MIT have combined advanced machine learning with sequential sampling techniques to forecast extreme events without relying on extensive historical data.
Limitations and Challenges in Prediction
Despite advancements in AI, predicting black swan events remains a major challenge, largely due to their unpredictability and lack of generalizability. Black swan events appear, by definition, novel and unprecedented. They don’t fit into existing patterns or models, making it difficult for AI, which relies on past data to make predictions, to anticipate them. This is compounded by the limited historical data available for rare events, which further hinders AI’s ability to identify potential precursors or warning signs.
The unpredictable nature of black swans, often triggered by complex interactions of factors (such as economic shifts, geopolitical instability, technological failures, and unforeseen social dynamics), also poses a significant challenge for AI models to accurately predict their occurrence or impact.
Conclusion
Black swan events in AI may be rare, but their impact can be profound and global, influencing markets, regulation, culture, international relations, science and innovation, and humanity’s overall trust in technology. The challenge isn’t to eliminate uncertainty but to build the resilience to navigate it.
Rather than seeing unpredictability as a threat, forward-thinking organizations are using it as an opportunity to strengthen governance, enhance risk management, and design AI systems that are adaptable, reliable, and accountable. The future of AI isn’t about avoiding disruption; it’s about being ready for it, learning from it, and using it as a catalyst for progress.
In our next piece, we’ll shift the focus to how organizations can prevent the domino effect of AI-driven black swan events. How can businesses identify and address the vulnerabilities before one failure sets off a chain reaction? Stay tuned as we explore the strategies that can help you protect against the unexpected and ensure stability in the face of disruption.
And if you’re ready to move from uncertainty to confidence, Lumenova AI can help. Book a demo today to see how our solutions empower organizations to thrive in an unpredictable world.