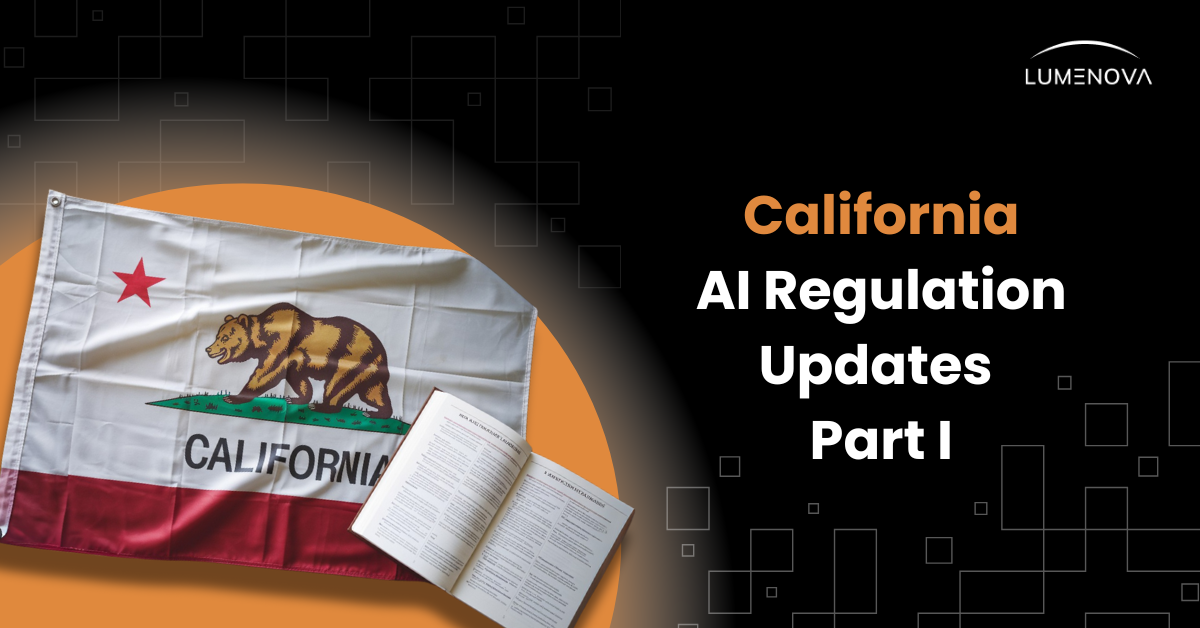
Contents
Despite the recent veto of California’s forward-looking yet controversial SB 1047—Safe and Secure Innovation for Frontier AI Models Act—Californian policymakers continue to represent a force to be reckoned with throughout the US AI policy landscape. In the continued absence of robust federal AI oversight and regulation, certain states have taken it upon themselves to lead the AI regulation charge, and California is one of a few that has emerged as a key player.
Throughout this post, we’ll break down and analyze two newly enacted California AI legislations—the Generative AI Accountability Act (GAIAA) and the AI Transparency Act (AITA)—while also briefly discussing a set of voluntary AI ethics guidelines, known as the Asilomar AI Principles, which were endorsed by the Californian state legislature in 2023.
Our approach will be structured and formal, beginning by identifying the stakeholders a legislation targets, followed by core definitions, an examination of regulatory provisions and requirements, and finally, a critical analysis that centers on feasibility—whether the policy will work as intended—and potential real-world impacts. We’ll start off with GAIAA, followed by AITA, and finally the Asilomar AI principles.
For readers interested in exploring other topics within the AI governance and policy ecosystem, we suggest following Lumenova’s blog, where you can maintain an up-to-date perspective on relevant regulatory developments while also exploring a wide range of additional fields like AI safety, ethics, and generative AI (GenAI).
California Generative AI Accountability Act
Executive Summary: GAIAA lays the foundation for the responsible and secure use of GenAI tools and applications within state agencies, emphasizing responsible AI (RAI) principles like transparency, equity, and protection for civil rights, particularly where AI is deployed in public-facing contexts. Core requirements include risk analysis on AI in critical infrastructure, disclaimers for GenAI in communications, bias mitigation and discrimination provisions, and the adoption of a flexible and proactive policymaking approach in response to GenAI impacts. More broadly, GAIAA also supports engagement with industry and the general public, aiming to build and maintain a forward-looking AI policymaking strategy that considers the course of AI advancements and impacts as they evolve.
Key Stakeholders and Definitions
Stakeholders:
- State government agencies that are actively deploying or considering deploying GenAI for public-facing communication and other related purposes.
- The Office of Emergency Services (OES) is responsible for administering GenAI risk analysis targeting GenAI-induced critical infrastructure risks to ensure public safety.
- The Department of Technology (DOT) is responsible for submitting regular reports on GenAI developments to the Governor.
- AI Developers & Industry Experts play a crucial and direct role in industry consultations, contributing to the creation of GenAI best practices.
- Members of the general public who could benefit from disclosure and human contact options when interacting with AI-generated content in the context of state communications.
- State employees and HR departments who are the subject of workforce development and AI expertise initiatives.
Definitions:
- GenAI: “An artificial intelligence system that can generate derived synthetic content, including text, images, video, and audio that emulates the structure and characteristics of the system’s training data.”
- Risk Analysis: “Analysis of potential threats posed by the use of GenAI to California’s critical infrastructure, including those that could lead to mass casualty events.”
Provisions and Requirements
Oversight and Reporting: The DOT must submit regular multi-faceted reports on GenAI developments, informed by academic, industry, and state employees, to the Governor’s office. The OES must also perform risk analyses regarding potential GenAI-induced threats to California’s critical infrastructure, particularly for large-scale events that could result in mass casualties.
Risk analyses must be annually evaluated by the state legislature to transparently identify viable strategies for mitigating emerging GenAI risks and ensure alignment between policymakers and safety concerns on behalf of the general public.
GenAI in State Communication: State agencies must ensure that any AI-generated communication content is clearly marked with a disclaimer—for written content, the disclaimer must be at the beginning, for online interactions, it must be present throughout, for audio, a verbal disclaimer is required at the beginning and end of the clip, and for video, a visual disclaimer must be present throughout the video’s duration.
For each piece of AI-generated communication content, irrespective of the form it takes, information on how to connect with a human representative must be provided.
Regulatory and Legal Considerations: To support and maintain a proactive approach to GenAI regulation, state agencies must regularly evaluate how GenAI advancements are impacting existing policies and legal standards.
State agencies are strongly encouraged to prioritize equity considerations during all stages of GenAI deployment, and must ensure that the systems they leverage never perpetuate discrimination based on sensitive characteristics like race or religion.
Workforce Development and Collaboration: While not a mandate, GAIAA supports the formation of state partnerships with industry and academia that drive AI skills procurement initiatives while centering on AI ethics, privacy, and security considerations. GAIAA also recognizes the importance of cross-agency collaboration in equipping state agencies with access to necessary AI training and expertise.
Consumer and Public Safety Protections: State agencies are required to protect citizens from GenAI-related risks, particularly those concerning financial stability, public health, and civil rights. If concrete rules and legal standards regarding this kind of consumer protection don’t exist, state agencies are encouraged to develop guidelines where relevant.
Critical Analysis
Feasibility & Implementation
GAIAA is fairly straightforward. However, it’s worth noting that it could create administrative complexity and technological viability challenges. From the administrative perspective, the Act’s mandates for regular reporting, risk assessment, and equity consideration could introduce administrative burdens that strain training budgets and human resources. By contrast, on the technological viability side, the ability to continuously provide unobstructed and persistent disclaimers throughout various media modalities may end up requiring robust monitoring systems for ensuring compliance—the act also does not propose any specific technical solutions for assessing GenAI-induced critical infrastructure risks in real-time, which constitutes a technically demanding feat on its own.
With respect to implementation, it is possible that recruiting and preserving AI talent in the public sector will be more difficult than expected due to notable competition and potent financial incentives in the private sector. On the other hand, cross-agency collaboration, depending on what bureaucratic hurdles are in place, could potentially delay or complicate implementation.
Impacts
We expect that GAIAA’s impact will be predominantly positive. In the short term, we predict transparency enhancements in public sector AI-assisted communications, which will contribute to the development of standardized accountability measures for government-specific AI use. The Act’s call to consistently update legislation as AI advances will further bolster this potential while also improving public safety measures by internalizing state-of-the-art methods for analyzing GenAI risks as they evolve.
In the long term, we hope that GAIAA will lay the groundwork for a more AI literate government workforce, significantly reduce the frequency of bias and discrimination-related incidents in state-run AI initiatives, and foster the development of strong collaborative relationships between state and industry, allowing Californian policymakers to proactively design future AI governance strategies.
Potential Avenues for Improvement
- Mandate—rather than encourage—state-sponsored initiatives that leverage industry partnerships to provide the necessary resources and training for state workforce AI upskilling and compliance programs.
- Implement a centralized oversight body responsible for streamlining cross-agency collaboration and coordination to ensure the reliable and consistent application of AI regulation, governance, and risk management best practices.
- Initiate a public awareness AI literacy campaign that not only educates citizens on GenAI skills and requirements but also allows them to understand what rights they have when interacting with AI systems.
California AI Transparency Act
Executive Summary: California’s AITA targets AI developers with more than one million monthly users, requiring developers to provide users with no-cost and easily accessible AI detection tools. These tools must enable the detection of AI-generated content while also allowing for the transparent verification of system provenance data. On the disclosure side, AITA further requires that developers attach both visible and embedded disclosures to AI-generated content, allowing users and viewers to peer into the origin of the content they interact with. As of now, AITA will enter into force on January 1st, 2026.
Key Stakeholders and Definitions
Stakeholders:
- AI Developers: Companies or individuals, with a user base that exceeds one million, that develop and deploy AI systems.
- Users: Individuals who interact with AI systems and require AI-generated content disclosure and detection tools.
- Third-Parties: Companies and individuals that license AI systems, regardless of whether they modify them or not.
- Regulatory Authorities: Regulatory authorities include the Attorney General, city attorneys, and county counsel, all of whom possess the authority to enforce AITA and impose penalties.
Definitions:
- GenAI: “An artificial intelligence that can generate derived synthetic content, including text, images, video, and audio, that emulates the structure and characteristics of the system’s training data.”
- Covered Provider (AI Developer): “A person that creates, codes, or otherwise produces a generative artificial intelligence system that has over 1,000,000 monthly visitors or users and is publicly accessible within the geographic boundaries of the state.”
- Manifest Disclosure: A permanent, directly visible, easily understandable, and content-appropriate disclosure that identifies AI-generated content.
- Latent Disclosure: A permanent but not directly visible disclosure that is detectable by a covered provider’s AI detection tool.
- Metadata: “Structural or descriptive information about data.”
- Personal Provenance Data: Data that contains “(1) personal information” or “(2) Unique device, system, or service information that is reasonably capable of being associated with a particular user.”
- System Provenance Data: “Provenance data that is not reasonably capable of being associated with a particular user and that contains either of the following: (1) Information regarding the type of device, system, or service that was used to generate a piece of digital content. (2) Information related to content authenticity.”
Provisions and Requirements
AI Detection Tools: Covered providers must build AI detection tools that allow users to determine whether content across image, video, audio, or some combination of these modalities, is AI-generated. However, if an AI detection tool is determined to pose tangible security threats, covered providers can enact reasonable access limitations and restrictions on the tool’s use. Moreover, AI detection tools must:
- Be easily accessible to members of the general public in addition to any other relevant stakeholders requiring the verification of AI-generated content.
- Reveal relevant system provenance data, including user-agnostic information regarding the origin of AI-generated content (e.g., device type and authenticity metadata).
- Never reveal personal provenance data to maintain robust user privacy standards.
- Enable content uploading either directly or via a URL.
- Support API access that allows other developers to seamlessly integrate the tool into their platforms and systems, bolstering the potential for widespread adoption.
- Be improved over time through user feedback collected and analyzed by covered providers—providers may not retain user contact details unless they are explicitly permitted to do so.
Manifest Disclosure: AI-generated content must be clearly and understandably labeled as AI-generated or manipulated, and labels must be designed such that they are nearly impossible to alter from a technical standpoint. Disclosure labels must also be appropriate to the kind of content that has been generated—a video might be watermarked whereas audio may have a verbal disclaimer.
Latent Disclosure: Latent disclosure labels must be detectable by the provider’s AI detection tool, enabling the verification of AI-generated content metadata, including specific provider information, system details, a timestamp, and a unique identifier. Latent disclosures must not be directly visible.
Third-Party Licensing and Compliance: Covered providers must ensure the presence of contractual obligations that require licensees to meet disclosure provisions. If providers discover that licensees have violated disclosure provisions, they must revoke their license within 96 hours and licensees must immediately cease further use of the AI system.
Enforcement and Penalties: Where providers violate AITA, they will receive civil penalties of $5,000 per incident and may also be subject to legal actions pursued by relevant regulatory authorities. Where licensees incur violations, they may be subject to civil lawsuits.
Exemptions: Entertainment-related and interactive content such as video games, movies, TV shows, and streaming services.
Critical Analysis
Feasibility & Implementation
Like GAIAA, AITA is also fairly straightforward, although we expect it will encounter some more significant challenges. For example, attaching permanent latent disclosures to AI-generated content could perpetuate unnecessary barriers for content distribution platforms that strip metadata. Alternatively, compliance costs, especially if they accumulate, could impose crippling financial consequences on smaller GenAI companies and start-ups, allowing big players, who can easily afford to pay these costs, to acquire even more control within the AI innovation landscape. On the enforcement side, the ability to track and prove violations could introduce further problems, especially since regulatory authorities would be relying on non-standardized AI detection tools built by AI providers.
This last point brings us to a major implementation challenge—developing universally compatible AI detection and disclosure systems that enable reliable and consistent content verification across multiple modalities and AI systems. This could further compromise the ability to adequately monitor third-party modifications to AI systems, which could complicate the enforcement process.
More subtly, AITA appears to facilitate some tension between security and accessibility, illustrated by the ambiguous leeway it grants providers in imposing limitations or restrictions on their AI detection tools, despite the requirement to make them widely available.
Impacts
In the short term, we will note a major uptick in the development of AI detection tools and content labeling systems, however, this will occur in the absence of standardized metrics for evaluating these systems’ performance and efficacy. We expect that this phenomenon will motivate legal controversy regarding the Act’s enforcement and interpretation, particularly as GenAI systems become more sophisticated while standardized content verification methods emerge.
In the long term, we hope that AITA will drive the development of standardized AI detection tools that can be consistently and reliably applied across various forms of GenAI technology and content. The development of such tools could also contribute to technical best practices for GenAI transparency in government and industry—more specifically, we envision the creation of detection tools that are purpose-built for specific GenAI-assisted government functions or industry-related tasks. Taken together, these factors could emerge as a driving force in the evolution of technical standards for AI content labeling, not just for performance monitoring, but also for the specific tools that we use.
Potential Avenues for Improvement
- Ensure that data provenance requirements do not end up stifling or preventing open-source AI research, despite potential data transparency improvements.
- Leverage industry and research partnerships to standardize the process of AI content disclosure, both in terms of technical development/maintenance and detection tool performance assessment.
- Small GenAI companies can accumulate significant user bases—exemptions and innovation grants for smaller companies should be granted where appropriate to offset potential compliance costs.
- While user feedback is required to improve AI detection tools, robust and easily accessible user-centric communication channels must be established.
Asilomar AI Principles
Executive Summary: Developed during a 2017 multi-disciplinary conference held in Asilomar, California, the Asilomar AI principles, of which there are 23, are intended to promote and uphold the safe, ethical, and beneficial development of AI. The principles target three main categories: research, values and ethics, and long-term risks. At a high level, the principles encourage and support the creation of AI systems that are aligned with human values, foster equitable and widespread AI benefits distribution, and maintain robust safety controls and mechanisms. The Asilomar AI principles, despite being endorsed by the California state legislature, are voluntary and serve as an ethical compass for the future of AI policy in California.
Seeing as the Asilomar AI principles are not an enforceable piece of legislation, we approach this non-binding ethical framework somewhat differently. First, the principles are transcendental, applying to AI developers and researchers, policymakers and government agencies, for-profit and non-profit organizations, and civil society, while stressing the imperative for global cooperation and engagement in AI ethics.
Second, while we won’t describe all 23 principles—this is a short document that readers can easily review on their own—we will summarize each section at a high level:
-
Research Issues: AI research should be guided by the overarching imperative of creating AI technologies that prioritize humanity’s well-being. To do so, investments in AI safety must be significant, mirroring the magnitude of relevant challenges across ethics, law, and social impacts. The need for the establishment of strong and reliable partnerships between industry, research, and policymaking must also be stressed. Overall, AI research should uphold a shared culture of transparency and cooperation while actively managing pressures that could create incentives for a “race to the bottom” on safety.
-
Ethics and Values: AI systems must be aligned with fundamental human values, preferences, and goals, adhere to robust safety standards, promote equitable benefits distribution, and be designed to ensure that humans can maintain control over their decision-making. AI systems should never be leveraged in ways that could undermine democracy and fundamental rights, and where such systems are misused, developers must be held accountable.
-
Long-Term Risks: The assumption that AI capabilities will flatline is a dangerous one, especially when considering the profoundly transformative potential of this technology alongside the existential risks it may inspire. Should future systems possess the capacity for recursive self-improvement, stringent controls must be implemented to prevent loss of control scenarios—should superintelligence ever emerge, humans must ensure that it serves the common good while adhering to ethical standards that clearly benefit all of humanity, not just one society or culture.
Due to their voluntary nature, there’s no way to guarantee that stakeholders will adopt the Asilomar AI principles, and we don’t expect these principles to become operationalized as enforceable legislation, either at the state or federal level, for a few reasons:
-
Related foundational RAI principles like transparency, explainability, robustness, non-discrimination, and human oversight and accountability are already emerging as global ethical standards within the legislative space. The broadness and universality of these principles suggest that they are better suited for customization across specific industries, sectors, state lines, and even national boundaries.
-
In the US, most enforceable AI legislation seeks to mitigate immediate risks posed by AI systems, with limited attention being paid to long-term and potentially civilization-scale risks like loss of control scenarios, human enfeeblement, and superintelligence. We expect that this trend will persist because we have a weak precedent to draw from when developing strategies for existential risk prevention—nuclear weapons are the most salient historical example here, but we must remember that we’re dealing with a fundamentally different technology that can proliferate and advance much quicker.
-
As AI innovates exponentially, the foundation of the socio-ethical fabric that binds society and culture will begin to shift, predictably in some ways and unpredictably in others. For example, surely we would want AI systems that are aligned with human values, but what happens when human cultures change in response to AI innovations? More specifically, what if the only way to reap the future benefits AI inspires is by trading off our right to personal privacy? These questions highlight a fundamental problem: how do we accurately and quickly measure AI-induced cultural and value-based shifts at scale?
-
The principles endorse notions such as shared benefits and the common good. However, in order for these notions to manifest themselves authentically in the real world, we need a general population that understands AI and its potential risks, impacts, and benefits. Currently, we are nowhere near population-scale AI literacy, and insofar as this remains the case, concepts like the “common good” will be defined by those with political and financial power.
Although the Asilomar AI principles are unlikely to become enforceable AI legislation, we do expect in-depth further dialogue regarding their evolution as AI advances, and this will be profoundly valuable, irrespective of whether the principles are maintained or changed. We further hope that this dialogue will engage all factions of society, from regular citizens and AI researchers to industry experts and policymakers while fostering a more open and collaborative AI ethics conversation globally. This kind of cooperation will be crucial in mitigating the effects of potential civilization-scale risks like AI arms races and recursive self-improvement—the Asilomar AI principles are concrete proof that we are seriously thinking about the future of AI and humanity, even if all of society is not on board yet.
Conclusion
Throughout this post, we broke down and analyzed two pieces of recent Californian AI legislation—GAIAA and AITA—as well as a set of voluntary ethics principles, known as the Asilomar AI principles. In doing so, we provided readers with accessible insights into what these regulations support while providing them with some intellectual tools that enable critical interpretation.
Our next piece in this two-part series will adopt an identical approach and cover three additional yet separate pieces of Californian AI legislation. In this respect, we urge readers to stay tuned for what comes next.
For readers who have already begun their AI risk management and governance journey, either formally or informally, we invite you to check out Lumenova’s comprehensive RAI platform and AI policy analyzer.