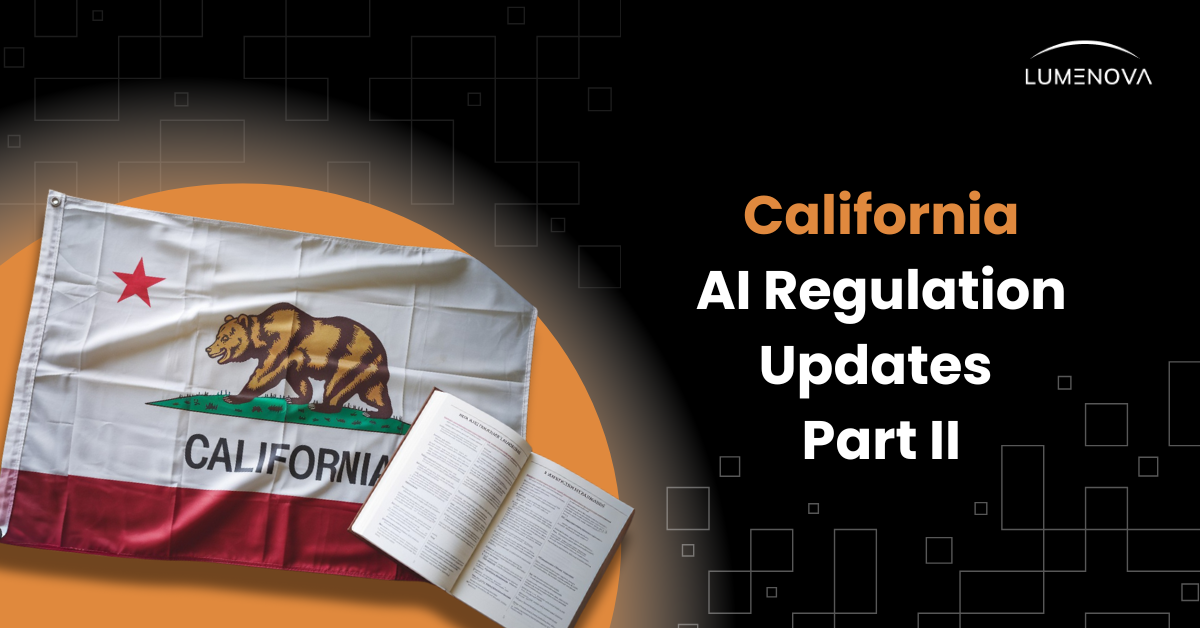
Contents
In part I of this series, we critically examined three pieces of Californian AI legislation—the Generative AI Accountability Act (GAIAA), AI Transparency Act (AITA), and Asilomar AI Principles. In doing so, we provided readers with an overview of what these legislations support and/or require while showcasing a series of potential impacts, limitations, or vulnerabilities they may encounter or induce when entering into force.
In this post, we will adhere to the same structured approach as we did previously, beginning with an executive summary, followed by a description of key stakeholders and definitions, a detailed breakdown of relevant provisions and requirements, and finally, a critical analysis addressing feasibility, implementation, and impacts. Therefore, the AI regulations this post will discuss include AB 2013 (AI training data requirements), AB 2885 (standardized AI definitions and reporting mandates), and AB 2876 (AI literacy provisions).
For those who wish to further explore emerging AI regulations or additional topics within the AI governance, safety, ethics, and generative AI (GenAI) landscapes, we recommend following Lumenova’s blog, where you can easily access high-level and in-depth content across each of these domains.
AB 2013
Executive Summary: AB 2013 requires GenAI developers who have deployed or modified a GenAI system to provide detailed information on AI training data in a publicly accessible manner. This information must describe details like data processing methods, outline whether personal or copyrighted data was leveraged, and include data sources and content characteristics. Overall, AB 2013 strives to improve transparency provisions for AI training procedures, with a targeted focus on data privacy and copyright considerations for GenAI systems. In certain cases where security concerns outweigh transparency needs, AB 2013 does grant some exemptions.
Key Stakeholders and Definitions
Stakeholders:
- GenAI Developers: Organizations and individuals who are deploying or modifying AI models on or after January 1st, 2022.
- General Public and Users: Citizens who benefit from a more transparent understanding of how training data fuels AI systems.
- Data Privacy Advocates: Groups or activists that are notably concerned with the sources and characteristics of AI training data, particularly in terms of copyright and privacy implications.
- Regulatory Bodies: The state agencies responsible for overseeing and monitoring compliance with AB 2013. Currently, it remains unclear which agencies, beyond the Department of Technology (DoT), will be responsible for doing so.
Definitions:
- Developer: “A person, partnership, state or local government agency, or corporation that designs, codes, produces, or substantially modifies an artificial intelligence system or service for use by members of the public.”
- GenAI: “Artificial intelligence that can generate derived synthetic content, such as text, images, video, and audio, that emulates the structure and characteristics of the artificial intelligence’s training data.”
- Substantial Modification: “A new version, new release, or other update to a generative artificial intelligence system or service that materially changes its functionality or performance, including the results of retraining or fine tuning.”
- Synthetic Data Generation: “A process in which seed data are used to create artificial data that have some of the statistical characteristics of the seed data.”
Provisions and Requirements
- Data Transparency Obligation: GenAI developers must provide comprehensive documentation on AI training data for systems deployed or modified on or after January 1st, 2022. All GenAI developers are subject to this requirement insofar as their system is available to Californian citizens, regardless of whether it is free or paid.
- Data Documentation: GenAI developers must include all of the following information in their data documentation, making it user-accessible via their website:
- Sources and Ownership: The origin of data must be specified, for instance, whether it was acquired from public domains, private entities, or proprietary datasets.
- Purpose Alignment: Training data must be aligned with the intended purpose of the developer’s AI system. For example, if a system is built for image generation, documentation must describe how training data supports this purpose.
- Quantity: Developers must disclose the total data quantity. However, to account for dynamic or regularly updated datasets, developers are permitted to disclose data ranges (e.g., “between 5 and 10 million data points”).
- Labeled vs. Unlabeled: Where datasets include labels, label types must be specified. Where datasets are unlabeled, a description of the dataset’s general characteristics must be provided.
- Intellectual Property (IP) Status: To ensure respect for IP rights, developers must disclose whether training data contains copyrighted, trademarked, patented, or public domain information.
- Licensing and Acquisition: Clarification regarding whether datasets are purchased, licensed, or freely sourced must be given.
- Personal and Consumer Information: Developers must issue a statement describing whether datasets contain personal information (data that could be used to identify individuals) or aggregate consumer information (data that illustrates consumer behavior patterns).
- Preprocessing and Modification: Where data is modified, data modification methods must be described in terms of how they contribute to or support an AI system’s intended purpose.
- Collection Timeframe: The time period during which data is collected must be specified—where data collection is continuous, a clear notice must be given.
- First Use Dates: To gain visibility into data usage throughout an AI system’s lifecycle, dates for when datasets were first leveraged for training purposes must be provided.
- Synthetic Data: Where an AI system utilizes synthetic data during training, a disclosure must be given alongside an explanation regarding its necessity and contribution to the system’s intended purpose.
- Substantial Modification: Whenever a significant modification or update is made to an AI system, irrespective of whether it concerns training data, it must be documented.
- Exemptions: GenAI systems used for security, national airspace operations, and national defense are exempt from these requirements.
Critical Analysis
Feasibility and Implementation
AB 2013 represents a substantial step in the right direction for the development of robust training data transparency and privacy requirements, especially when considering that both GenAI developers who offer free and paid services are held to the same legal accountability standards. Moreover, the bill’s focus on public accountability, particularly in light of its lack of enforcement provisions, will support unobstructed public access to training data details, bolstering trust in AI systems while showcasing potential data misuse and sourcing concerns, most notably where copyrighted or sensitive data is leveraged.
However, AB 2013 could perpetuate some noteworthy challenges. For instance, the bill’s data lineage and characteristics requirements, which constitute several core provisions, could introduce technological barriers that manifest as logistical difficulties with scaling reliable procedures for tracking and documenting data sources accurately, especially where data is gathered continuously. Additionally, data source disclosure requirements could fuel a counterproductive incentive on behalf of GenAI developers who might now be motivated to risk non-compliance to obscure proprietary information and avoid giving up a competitive advantage.
Furthermore, in many cases, the datasets leveraged to train GenAI systems are not only enormous and frequently dynamic, but also contain information from multiple disparate sources. This suggests that cases where documentation must be simplified are likely to emerge, which begs the question of whether such simplified documentation will prove equally useful in demonstrating a commitment to transparency requirements. Alternatively, on the public-facing side, AB 2013 implicity assumes that non-AI and data literate citizens will have no trouble interpreting data documentation, which, frankly, is not a realistic assumption.
Impacts
Within the next few to several years, we expect that AB 2013 will generate significant impacts:
- Increased AI Scrutiny: As members of the general public and other key stakeholders (e.g., data privacy advocates) develop a more profound and grounded understanding of AI training data, GenAI developers will start facing increased public scrutiny which could heighten litigation frequency, most notably for IP rights infringement claims.
- Greater Trust and Reliability: As AI companies deal with public scrutiny, they will need to prioritize maintaining a positive reputational standing, showcased by their unwavering commitment to data transparency and privacy standards, which will manifest in terms of the trust and confidence end-users place in their AI systems.
- Standardized Data Documentation: Early compliance challenges and emerging GenAI advancements will reveal the need for standardized tools and methods for tracking and reporting training data usage in GenAI systems.
- Legal Implications: AB 2013 could become a foundational piece of AI legislation due to the concrete and targeted transparency boundaries it enacts for AI training data, driving widespread influence on future AI-related IP and data protection laws in California and perhaps even other states throughout the US.
Potential Avenues for Improvement
- Develop and establish accessible and easy-to-interpret guidelines on acceptable thresholds for data abstraction to help GenAI developers navigate situations in which the need for transparency must be balanced with practicality.
- Require GenAI developers to include publicly accessible educational resources on their websites that enable members of the general public to clearly understand and evaluate data documentation.
- Build direct communication channels with members of the general public so that data-related concerns can be more quickly identified and resolved.
- Support and encourage the creation of technical tools and methodologies that standardize data documentation processes for GenAI developers, either via industry, research, and/or academic partnerships.
- Implement enforcement penalties, either in the form of fines or legal action, for GenAI developers who violate any of the bill’s training data transparency provisions.
- Consider whether open-source AI developers will be subject to training data transparency requirements, and what these requirements might look like.
AB 2885
Executive Summary: AB 2885 lays the foundation for a standardized framework through which to define, inventory, and oversee AI, and more specifically, the use of high-risk automated decision systems (ADS) within government bodies. By enforcing strict AI use, impact, and/or reporting standards for state and local agencies as well as social media companies utilizing AI for content moderation purposes, AB 2885 aims to create a robust sense of public accountability while offering Californian citizens legal protection against AI-induced biased outcomes and other potentially harmful impacts.
Key Stakeholders and Definitions
Stakeholders:
- State Agencies, namely the DoT and other interagency bodies (where appropriate), must build an inventory of all high-risk ADS that are already deployed or proposed for deployment.
- Local Governments are required to assess economic AI impacts, especially with respect to how they affect economic development subsidies and workforce automation practices.
- Social Media Companies must regularly report on content moderation initiatives and specify what role AI plays in monitoring and removing certain kinds of content.
- General Public: Citizens who are on the receiving end of economic AI-induced impacts, particularly in consequential decision-making and automation contexts.
Definitions:
- AI: “An engineered or machine-based system that varies in its level of autonomy and that can, for explicit or implicit objectives, infer from the input it receives how to generate outputs that can influence physical or virtual environments.”
- Automated Decision System (ADS): “A computational process derived from machine learning, statistical modeling, data analytics, or artificial intelligence that issues simplified output, including a score, classification, or recommendation, that is used to assist or replace human discretionary decisionmaking and materially impacts natural persons. “Automated decision system” does not include a spam email filter, firewall, antivirus software, identity and access management tools, calculator, database, dataset, or other compilation of data.”
- High-Risk ADS: “An automated decision system that is used to assist or replace human discretionary decisions that have a legal or similarly significant effect, including decisions that materially impact access to, or approval for, housing or accommodations, education, employment, credit, health care, and criminal justice.”
- Economic Development Subsidy: “Any expenditure of public funds or loss of revenue to a local agency in the amount of one hundred thousand dollars ($100,000) or more, for the purpose of stimulating economic development within the jurisdiction of a local agency, including, but not limited to, bonds, grants, loans, loan guarantees, fee waivers, land price subsidies, matching funds, tax abatements, tax exemptions, and tax credits.”
Provisions and Requirements
- Standardized Definitions: AB 2885 standardizes AI, ADS, and high-risk ADS definitions across multiple government codes.
- Inventory Mandate: The DoT, in tandem with relevant interagency bodies, must build a comprehensive inventory including all high-risk ADS that are actively deployed or intended for deployment by state agencies. Inventory content must cover:
- Decision Descriptions: The decisions a system drives or supports, and their intended benefits, must be described.
- Justification: Alternatives to high-risk ADS must be identified and documented, illustrating why the high-risk ADS is the preferred option.
- Comparative Performance Assessments: Where there exist comparative studies or evaluations between an ADS and other potential solutions, results must be included.
- Data Disclosure: ADS data categories must be disclosed and specify whether any personal data is involved.
- Risk Mitigation Measures: Comprehensive details on ADS accuracy metrics, privacy safeguards, cybersecurity provisions, bias and discrimination audits, and contestability options (mechanisms or procedures through which those impacted by an ADS can contest its decisions) must be provided.
- Annual Reporting: The DoT must submit standardized and accessible annual inventory reports to the Assembly Committee on Privacy and Consumer Protection and the Senate Committee on Governmental Organization. By contrast, local agencies must:
- Report on Economic Development Subsidies: Annual reports detailing AI-induced net job impacts and subsidy performance (e.g., whether job creation and workforce retention goals/strategies are successful) must be made publicly available. Local agencies must also hold annual public hearings dedicated to openly discussing the reports they submit.
- Economic Development Subsidies: Prior to granting subsidies, local agencies must publish information on recipient details, subsidy terms, economic impacts (e.g., tax revenue generated and number of jobs created), workforce composition, and plans for disadvantaged workers.
- Social Media Obligations: Semiannual reports covering the use of AI in content moderation must be submitted to the Attorney general. The details of these reports must include an explanation of how AI flags or removes content, the role of human reviewers in content moderation, and user-driven content moderation efforts (e.g., community moderators or external partners).
- Exemptions: Certain AI tools that do not pose a tangible threat to human rights and economic opportunities—spam filter or antivirus software for instance—are excluded from the high-risk ADS classification.
Critical Analysis
Feasibility and Implementation
Under AB 2885, state agencies will need to construct reliable systems for tracking and auditing high-risk ADS, which will include training key staff members to manage inventories and administer risk mitigation measures effectively. This could place a heavy administrative burden on state agencies—one that will be further exacerbated by regular reporting requirements and the need for sustained coordination and cooperation across several relevant interagency bodies, a process that is easily complexified by bureaucratic needs and procedures.
Moreover, the ability to comprehensively compile data on high-risk ADS systems will also prove challenging, particularly when considering the increasing rate at which diverse AI applications are being applied across state agencies. We might also question the supposed effectiveness of public hearings seeing as their efficacy depends on public engagement levels and agencies’ ability to adequately integrate feedback-driven changes. There is a possibility that these kinds of hearings will become performative regulatory formalities that do not actually reveal any meaningful substantive information.
On the upside, AB 2885 does standardize key technological definitions—the impact of this milestone should not be underestimated—while building on existing data protection legislation (e.g., California Consumer Privacy Act) to drive the development of more robust and transparent AI inventory/reporting and social media governance standards. In this respect, AB 2885 will inspire an increasingly potent sense of public accountability, not only on behalf of state agencies and social media companies but also the general public.
Impacts
In the short term, we hope that the inventory and reporting provisions AB 2885 enforces will provide policymakers with direct insights into understanding, anticipating, and mitigating AI-related risks proactively, particularly where systems are leveraged for consequential decision-making in critical domains like housing and education.
Relatedly, and on the local government side, the requirement to transparently evaluate and publicly report on the economic implications of AI-driven automation could inspire preemptive economic adjustments that materialize as forward-looking workforce development strategies, aiming to positively impact job retention and creation goals. Taken together, these factors could position relevant Californian government bodies as leading national regulatory authorities on AI impacts and workforce automation trends.
Looking further down the line, we expect that the process of policy-making will become inherently more data-driven as we build increasingly comprehensive AI impact knowledge bases. However, whether these knowledge bases will equally represent the interests of the general public as they do those of state and local governments and social media companies, remains unclear—a method to incentivize members of the general public to join in on public hearings should be developed.
On a more worrisome note, we do not have good reason to expect that major social media companies will readily adhere to the bill’s reporting provisions due to their well-established track record of violating data protection and privacy laws globally. For social media companies, substantial regulatory enforcement penalties, that range beyond legal action, will likely be necessary.
Potential Avenues for Improvement
- Develop and implement standardized templates for AI impact and risk reporting within state and local government agencies. These templates should be regularly reviewed by industry and academic partners to ensure continued relevance as AI advances.
- Mandate investments in AI literacy training for public officials and local agency employees—a specific annual budget for this training should be established and reviewed yearly.
- Implement financial compliance penalties for major social media companies—classified according to user base—that accrue on a violation-by-violation basis.
- Encourage and support the creation of direct interagency coordination and communication channels that eliminate undue bureaucratic inefficiencies.
- Provide members of the general public with free access to AI education and upskilling resources to incentivize attendance to and engagement with public hearings on AI-driven economic impacts.
- Consider allowing state agencies to test high-risk ADS in regulatory sandbox environments before deployment and mandate that testing results are publicly reported.
AB 2876
Executive Summary: AB 2876 supports two major notions: AI and media literacy. Specifically, the bill requires the Instructional Quality Commission (IQS) to begin integrating AI and media literacy provisions into state curriculum frameworks across several core subjects, equipping students with the knowledge and skills necessary for critically engaging with and analyzing various kinds of AI technologies and media content. AB 2876 also offers a concrete AI literacy definition, which, broadly speaking, includes knowledge of foundational AI principles, potential use cases, limitations and social impacts, and relevant ethical considerations.
Key Stakeholders and Definitions
Stakeholders:
- Instructional Quality Commission (IQS): The government body tasked with updating educational curriculums and making recommendations on the adoption and integration of various instructional materials concerning AI and media literacy.
- State Board of Education: The board that will adopt the curriculums and recommendations provided by the IQS.
- California Public School Teachers and Students: Educators will receive updated teaching resources and materials whereas students will begin learning about AI and media literacy across core academic subjects.
- Educational Organizations: Primarily educational content developers and publishers, these stakeholders are required to ensure alignment between their resource offerings and newly established curriculum standards.
Definitions:
- AI Literacy: “The knowledge, skills, and attitudes associated with how artificial intelligence works, including its principles, concepts, and applications, as well as how to use artificial intelligence, including its limitations, implications, and ethical considerations.”
- Media Literacy: “The ability to access, evaluate, analyze, and use media and information and encompasses the foundational skills that lead to digital citizenship.”
- Digital Citizenship: “A diverse set of skills related to current technology and social media, including the norms of appropriate, responsible, and healthy behavior.”
Provisions and Requirements
- AI Literacy Integration: The IQS must include AI literacy content across curriculums for mathematics, science, and history-social science upon their next scheduled revision following January 1st, 2025.
- Instructional Materials: When the Board of Education integrates new instructional materials across the aforementioned curricula, AI literacy must be evaluated as part of the selection criteria.
- Media Literacy Integration: The IQS must incorporate media literacy content across all educational subjects and ensure that such content is aligned with Model Library Standards.
- Instructional Material Evaluation: The IQS, when reviewing and recommending instructional materials, must include AI and media literacy in its core criteria.
Critical Analysis
Feasibility and Implementation
Due to the lack of specific AI and media literacy provisions, it is difficult to say to what extent AB 2876 will meaningfully affect California’s K-12 education system, though it is impossible to deny the value of mandating AI and media literacy in public schools. Overall, we do believe that the bill will lay at least some groundwork for allowing students to learn how to be responsible and proactive digital citizens in the age of AI.
Sadly, we think that educators will run into numerous challenges when adapting to new AI-specific curricula and instructional materials—the vast majority of teachers simply will not have the skills and knowledge required to accurately and effectively teach AI concepts, regardless of whether they are hard science, humanities, or arts-oriented. These issues will apply to the California IQS and Board of Education as well—although perhaps to a lesser degree—which is concerning when considering that these bodies are primarily responsible for recommending and adopting educational AI and media literacy standards.
Building and administering AI and media literacy programs for educators will also prove to be a monumental task because these programs will need to be designed to dynamically suit teachers’ skill sets and knowledge bases, dissuade potential automation or replacement fears, and align with the subjects they teach. Before AI and media literacy training even begin, schools will need to understand what their educators’ AI needs are, and educators themselves may not be inclined to freely disclose this information for fear that they will be replaced by others who are more AI and media literate.
The pace at which AI advances and proliferates will further complexity and augment the problems we have just discussed—AI and media literacy skills requirements will change rapidly and unpredictably while the technology evolves. Comprehending what constitutes a sufficient degree of AI and media literacy in this context remains a crucial issue.
Impacts
In the short term, we predict the following impacts:
- Modernized Education: Even though AB 2876 is vague, it is the first active bill of its kind, and does indicate the Californian education system’s interest in embracing AI to drive its democratization and create a generation that is much more equipped to thrive in an AI-enabled future.
- Curriculum Failures: Many initial AI-specific curriculum and instructional material adjustments will result in widespread failures throughout multiple public school districts across virtually all relevant subjects. These failures will be a product of inadequate AI and media literacy training provided to teachers before curricula are updated and adopted.
- Revised AI Literacy Definition: As early-stage educational AI literacy failures accrue, policymakers will realize the importance of redefining what constitutes AI literacy for educators. Within reason, this may inspire the lowering of certain AI literacy standards, but only for educators.
In the long-term, impacts may include:
- AI and Media Literacy Metrics: As AI and media literacy evolve into core selection criteria for educational content development, educators and students will require metrics through which to reliably assess their AI and media literacy proficiency over time.
- State-Wide Teacher Training Programs: To address teacher needs across AI and media literacy and in consideration of the specific subjects an educator specializes in, the state will need to subsidize low/no-cost training programs tailored for teachers of different backgrounds and skill levels.
- Public Awareness and Engagement: As students and teachers improve their AI and media literacy, parents and local communities may be more motivated to engage in discussions on the role of AI in education and society. This could foster a more open and inclusive dialogue on key issues like AI ethics and safety.
Potential Avenues for Improvement
- Conduct pilot AI and media literacy programs with a selection of high-performing schools throughout diverse school districts to refine teaching methods and materials before initiating state-wide rollout.
- Fund and reward teachers who are interested in improving their AI and media literacy to incentivize educators to embrace the technology and stay ahead of the AI learning curve.
- Run community engagement sessions with parents and local community members to highlight the importance of incorporating AI and media literacy into the modern-day educational curriculum.
- Build a standardized set of metrics, that can be easily adapted when necessary to suit AI advancements, to evaluate AI and media literacy over time.
- Establish AI and media literacy initiatives for students and educators who are struggling to learn about these subjects at the same pace as their peers to minimize the risk of a digital divide.
Conclusion
In this second and final part of our series on California AI regulation updates, we described and analyzed three recently enacted legislations—AB 2013 (training data transparency), AB 2885 (standardized definitions and reporting requirements), and AB 2876 (AI and media literacy). In doing so, we prompted readers to dive into the details of these legislations while exploring each from a critical perspective.
While none of these regulations are perfect by any means, each does represent a significant step forward and we must remember that when policies do not work as intended, failures still tend to be more useful and informative in the long run than successes. Nonetheless, we must move quickly—failures to sufficiently regulate AI now could be dramatically inconsequential in comparison to failures to regulate the technology even two to three years from now.
But it is not just policymakers and citizens that must concern themselves with the future of AI regulations—companies should also be approaching this issue with intensity and proactivity. In this respect, we invite our readers to check out Lumenova’s Responsible AI platform and AI policy analyzer