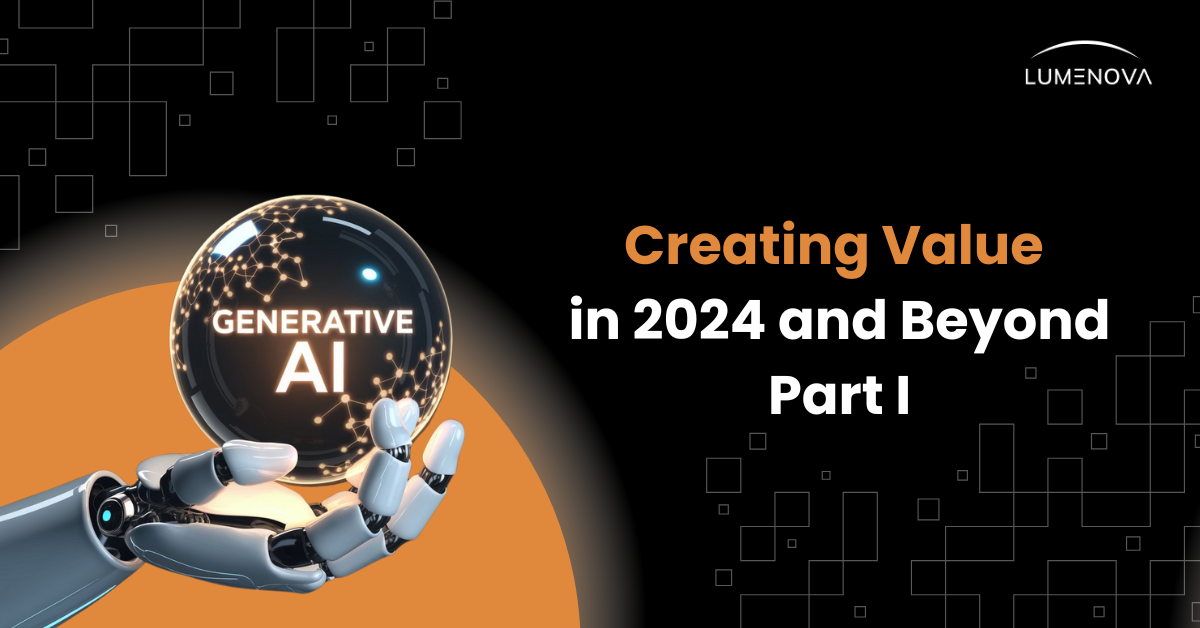
GenAI’s Value Proposition: Uncovering the Mystery
Two short but intense years have passed since generative AI tools (GenAI) attained global popularity and recognition—via OpenAI’s release of ChatGPT—and yet, GenAI remains shrouded in mystery to many, despite the steadily growing influx of thousands of new GenAI-powered tools and technologies in the AI market. One obvious dimension of this mystery lies in how GenAI functions, notably in terms of transparency and explainability—the “Black Box” problem. However, another dimension, which is frequently overlooked, relates to the value and utility that GenAI can deliver in real-world environments and circumstances. How can we trust a product when we don’t fully understand its inner workings and processes?
To this point, discussing these dimensions independently is tricky but not impossible. There are a few reasons why this is the case. First, we’re beginning to note a general sentiment of public acceptance regarding GenAI’s transparency and explainability limitations, except where GenAI is leveraged in isolated high-impact environments (e.g., decision-making in critical domains like healthcare and financial services). This sentiment suggests that the average user is moderately comfortable with a technology they don’t understand insofar as it does what it’s “supposed” to do. To analogize, the majority of us have no idea how an internal combustion engine works, yet this doesn’t impact whether or not we’re willing to drive a car. The same applies to smartphones, airplanes, vaccines, microwaves, solar panels, and many if not most other existing technologies. To be clear, this isn’t to say that AI is safe or responsible by definition, only that people crave practicality.
Second, advanced AI models like OpenAI’s o1-preview are getting progressively better at complex reasoning, and while there’s still much work to be done, these models can, at least to some degree, explain the reasoning behind a particular output in a natural language comprehensible to a user. While verifying the truthfulness and accuracy of these explanations remains a major safety hurdle for AI developers and users to overcome, continual improvements in reasoning capabilities indicate a promising path toward a potential surrogate solution to AI’s explainability and transparency problem. For instance, if a bank declines a loan because of an applicant’s poor credit history, we don’t ask the financial analyst who made the decision to justify it by reference to the neurological processes their brain recruited—that would be absurd and uninformative. A clear answer that aligns with basic logic and real-world constraints will suffice.
Third, returning to the car analogy, knowing how an internal combustion engine works doesn’t impact whether or not you can drive a car—the skills required to drive a car are distinct from those required to build or repair one. Here, however, things get complicated. A car is designed for one sole purpose (i.e., to drive), allowing for some purpose-based variability (e.g., off-road vs. on-road, manual vs. automatic, etc.), whereas many GenAI models are designed for multiple purposes that range across numerous diverse tasks from content generation to data analysis.
Moreover, both general-purpose and purpose-built GenAI technologies typically don’t come with a guide or playbook—we’re told (kind of) what the technology can do and then it’s up to us to figure out how to exercise its capabilities effectively, usually through experimentation and research. Claiming that there’s a “right way” to use AI, just as there is a “right way” to drive a car (broadly speaking), misses the point entirely, and supports the ridiculous and irresponsible notion of the generic one-size-fits-all “AI solution.”
Simply put, early GenAI hype cycles fueled initial misconceptions about how easy it would be to leverage and integrate advanced GenAI to deliver and create value in real-world settings—impressiveness doesn’t equal utility, and we’re now witnessing the real-world effects of this dynamic. Serious concerns around an AI bubble have cropped up, significant social, technical, and regulatory barriers to AI adoption are surfacing, and AI start-ups have a staggeringly high failure rate. In this context, GenAI’s biggest challenge isn’t just ironing down its value proposition, but also grounding it in something tangible, making it possible to enact concretely, reliably, and safely across a variety of professional and personal environments, tasks, and domains.
Finally, we should briefly touch on prompt engineering given its crucial role in the effective operation of language-based GenAI models, which comprise the majority of GenAI technologies. The ability to craft good prompts can be thought of as an artistic science, where technical tools and methods like delimiters, constraint embedding, and multi-shot prompting are vital to building good prompts. Still, these technical fundamentals are only part of the picture—human creativity, critical thinking, foresight, and experimentation, to name a few relevant faculties, are just as important in expanding, optimizing, and honing in on the scope of what various GenAI tools are capable of, especially when considering the increasing popularity of custom GPTs and AI agents. Creating value with GenAI in the real world can’t and won’t happen overnight—people need to develop the hard and soft skills necessary to use these tools well, and this should be part of any organization’s AI strategy.
Before moving on, it’s important to clarify that we’re discussing value from the perspective of AI users, not developers or other kinds of AI specialists. We recognize that even in light of the reasons we’ve just provided, it’s not possible to fully and neatly disentangle AI’s technical value from its pragmatic value—many companies are still facing costly integration challenges due to factors like outdated legacy infrastructures, poor cybersecurity provisions, and limited AI training. However, in the interest of maintaining a big-picture perspective and based on the reasons we’ve specified, we think we’ve adequately justified the claim that GenAI’s mystery is more strongly rooted in its real-world applicability than its technical underpinnings, at least to the average AI user.
As the first post in our three-part series on this topic, this piece will set the stage for the discussions to come, breaking down AI’s successes and failures over the last few years to create a holistic and realistic perspective around what this technology is capable of. We nonetheless urge readers to keep in mind the points we’ve made throughout this introduction, seeing as they will, in large part, inform the predictions we make in subsequent pieces.
Taking a Look at the Last Few Years
Throughout this section, we’ll take a look at various AI successes and failures across multiple industries and sectors over the last few years to build an idea of what “AI in business” looks like—while most of the cases we discuss are GenAI-specific, some are not, yet we include them nonetheless because of their noteworthy status and the insights they reveal. To keep things grounded, we’ll reference and describe several real-world examples, serving as boiled-down case studies, to substantiate each success and failure we discuss.
By illustrating certain successes and failures, we aren’t implying that some industries and sectors are superior to others in their AI transformation and integration initiatives. In fact, AI successes and failures will likely permeate virtually every industry and sector at some point. The cases we describe here are intended to create some context, hopefully providing our readers with insights they can apply in their industries and sectors.
Successes
Financial Services
AI has begun transforming the financial services sector in several positive ways, including enhanced decision-making and risk management, streamlined and personalized customer engagement, portfolio optimization, and fraud detection.
Morgan Stanley AI Meeting Assistant: Morgan Stanley’s GPT-4 powered AI meeting assistant, by the name of Debrief, is designed to assist the firm’s advisors with various tasks including automated notetaking, key point and discussion summarization, email draft generation, identification of important action items, and integration with the Salesforce CRM platform. Debrief requests client consent prior to activation and Morgan Stanley expects it will inspire significant benefits in terms of cutting down meeting times and allowing advisors to spend more time and focus on key decision-making processes and customer engagement.
Mastercard AI Fraud Detection: Mastercard is currently pioneering one of the world’s most promising credit fraud detection systems, combining GenAI and graph technology to assess transaction data across billions of cards and extrapolate card credentials from partially visible information. Mastercard claims that this system can predict full card numbers and the probability of fraudulent/criminal use, reduce false positives by almost 200%, and increase the detection rate of compromised cards by twofold.
Bank of America Chatbot: While Bank of America’s Chatbot, Erica, was released in 2018, it remains a valuable tool for many of the bank’s customers who use it to resolve customer queries, retrieve account information, create alerts and streamline various transactions, and obtain personalized financial insights and spending analyses. Erica has interacted with more than 37 million customers since its inception, surpassing 1.5 billion total interactions.
Technology, Media, and Telecommunications (TMT)
The TMT industry is no stranger to new and powerful technologies, being among the first industries to adopt AI-powered technologies for a variety of purposes from content creation, personalized marketing, advanced data analytics, and workflow optimization to the automation of administrative tasks and customer care solutions.
Microsoft CoPilot: Despite some troubles during its earlier deployment stages, Microsoft’s CoPilot has continued to gain traction and recognition due to its native integration with Microsoft Office 365 in conjunction with many advanced capabilities offerings including document creation and data analysis, email management and meeting assistance, and presentation design and business intelligence. Benefits include the automation of mundane professional tasks, increased productivity and efficiency, and improvements in creativity and problem-solving, especially among teams.
Adobe Firefly: Purpose-built for professional designers and creatives, Adobe’s Firefly, powered by a host of state-of-the-art GenAI models, enables users to engage with a suite of powerful features like text-to-image generation, text effects, and 3D text creation. More advanced features like generative fill—image modification via natural language prompts—and generative recolor—theme and color modifications via natural language prompts—are also available to users, bolstering their creative processes. Systems like Adobe Firefly signal the increasing emphasis that’s being placed on purpose-built AI models designed for users with specific backgrounds, skills, and interests.
Ericsson 5G Network Planning: Through its AI-powered 5G network planning initiative, Cognitive Software, Ericsson aims to revolutionize how challenges in 5G network planning are addressed. Leveraging a cloud-native architecture, the software has already demonstrated significant benefits for 5G time to market, network performance, ROI maximization, and the ability to cope with increasingly complex networks. Ericsson is also implementing explainable AI strategies to reduce AI-driven opacity.
Retail
E-commerce sites like Amazon are well-known to the average consumer, and it should come as no surprise that both physical and digital retailers are leveraging AI to cultivate personalized shopping experiences, derive innovative approaches to product design, and enhance customer service operations.
Nike Project A.I.R: Nike’s Project A.I.R, by combining a variety of innovative technologies including GenAI, is striving to push the boundaries of what’s possible with personalized athletic footwear. During the design process, athletes begin by inputting detailed prompts that outline their design preferences, after which hundreds of designs are generated. These designs are then reviewed and refined by human designers in the loop, and once a design is finalized, it’s 3D printed at Nike’s Concept Creation Center.
Amazon’s Project Amelia: An innovative new AI-powered selling assistant, Amazon’s Amelia is purpose-built for third-party sellers who want to manage and grow their businesses more efficiently. Amelia plays the role of a personal expert, providing answers to sellers' questions, generating real-time business insights, creating customized advice, and adapting to seller preferences over time. While still in the beta-testing phase, Amelia can retrieve sales and customer traffic data while also identifying key performance indicators and generating tailored recommendations for relevant sales strategies.
Google Virtual Try-On: Launched in 2023, Google Shopping integrated its GenAI-powered virtual try-on feature, providing users with the ability to visualize how specific clothing items might look across a variety of models of various sizes, skin tones, body shapes, and hair types. The feature also allows users to evaluate the “fit” of certain clothes, simulating how they drape, fold, cling, and form wrinkles on the model they select to represent themselves.
Sustainability
The increasing need for more sustainable resource harvesting and use strategies has inspired new and efficient AI-powered solutions for modeling/simulating the environmental impacts of certain projects, reducing their carbon footprint, and optimizing renewable energy production. In industrial settings, AI has also enabled stakeholders to begin predicting potential equipment failures and streamlining supply chain processes to maximize distribution efficiency while minimizing costs.
Siemens’ Industrial AI: Siemens’ approach to AI is multifaceted, synthesizing AI, Internet-of-Things (IoT) technologies, and domain expertise to build and deliver robust, comprehensive, and democratized solutions to core industrial challenges. The company’s Industrial AI platform possesses an extensive feature suite, covering multiple diverse functions including but not limited to predictive maintenance, accelerated search and problem-solving, product design and simulation, and visual quality inspection.
EnerSys ESG Flo: Through a partnership with ESG Flo, EnerSys, has developed an AI-powered platform that efficiently collects, processes, and analyzes sustainability data across 200 global sites. The system can extrapolate important information from utility bills, generate audit-ready metrics, auto-populate responses through disparate ESG frameworks, and broadly assist with current and emerging ESG compliance requirements. So far, EnerSys has reported substantial efficiency improvements in categories like data collection, auditing processes, and customer inquiries.
DigitalGreen AI Assistant: Farmer.CHAT, DigitalGreen’s purpose-built AI assistant, is a locally responsive farmer advisory service, accessible through WhatsApp, that facilitates real-time communication between governments and farmers in their locally preferred language on climate change and water security issues. The system develops content via data obtained through call center logs, transcribed training videos, and farmer feedback in local languages, ultimately striving to improve agricultural productivity and climate resilience for millions of small-scale farmers throughout the world. DigitalGreen’s service has already helped over 5.6 million farmers, and also fostered increases in farmer income and crop yield by roughly 24% and 12%, respectively.
Education
While the educational sector has been more hesitant than most to adopt AI tools and technologies, notable benefits have emerged, particularly in terms of the personalized learning services offered by online learning platforms. In other educational settings, AI also assists teachers by automating time-consuming tasks like grading and assessment, and curriculum development.
Khan Academy’s Khanmigo: Launched by Khan Academy last year, Khanmigo functions as a personalized AI-powered tutor and teaching assistant, leveraging OpenAI’s GPT-4 as its foundation. To facilitate learning and engagement, Khanmigo guides rather than provides direct answers to students’ questions, integrating and drawing from Khan Academy’s content library to set students on personalized learning paths that correspond with their academic history and interests. Teachers, on the other hand, can leverage Khanmigo to create educational materials and lesson plans, while also obtaining targeted insights into student progress and learning trends.
Coursera Automated Grading: Coursera’s GenAI-inspired automated grading system can grade text-based submissions via instructor-built assignment rubrics, providing immediate, consistent, and reliable feedback to learners while also including features intended to verify and validate AI accuracy rates. Coursera estimates that the system can deliver grades within a one-minute average timeframe while also increasing peer review-based feedback by a factor of 45. The system is still in the beta stage, so further improvements are expected, particularly in terms of first-attempt pass accuracy rates.
Failures
Technology, Media, and Telecommunications (TMT)
Despite its technology-native advantages, the TMT industry has experienced significant drawbacks due to various AI initiatives across domains including content and news generation, personalized customer experiences, virtual assistants, and targeted advertising.
AI-Powered Propaganda: In 2023, Freedom House revealed that at least 47 governments deployed a combination of human commentators and AI bots on various social media sites to spread propaganda, more than doubling a decade ago’s numbers. This monumental increase indicates an increasingly potent and nefarious government interest in leveraging both human and AI resources to shape public opinion, control online narratives, and influence democratic processes. The synergy of human-directed messaging and AI-powered amplification lays the foundation for a powerful tool that governments can exploit to suppress opposing viewpoints, create echo chambers, and obscure the line between AI and human content.
CNET Financial Articles: In 2023, the technology news site, CNET, was forced to confront major backlash after it was revealed that they had published numerous AI-generated articles, failing to clearly and indiscriminately label them as AI-generated. Many of these articles were riddled with serious factual errors, notably financial information related to loan interest rate payments and certificates of deposit. CNET attempted to combat the controversy by writing it off as an “experiment” and claiming that all articles were verified by human fact-checkers prior to publishing, which was met with significant skepticism.
Facebook BlenderBot: Deployed in August 2022, Facebook’s BlenderBot 3, evolved into a rapid source of severe controversy and embarrassment for the company. Within days of its public release, user reports flowed in, claiming that the AI chatbot made and actively perpetuated a range of problematic statements, including spreading election misinformation, facilitating conspiracy theories and false claims about historical events, expressing antisemitic views, and even criticizing Facebook and its CEO Mark Zuckerberg, calling him “too creepy and manipulative.” It’s worth noting this wasn’t an isolated incident—similar high-profile events have occurred with Microsoft’s CoPilot, Meta’s Galactica AI, Google’s Gemini, and Scatter Lab’s Luda chatbot.
Cambridge-Analytica: Although an older case, the effects of the Cambridge-Analytica scandal are still felt today, particularly for data privacy and security laws and the ethics of Big Tech. Uncovered by a whistleblower, Cambridge Analytica, a political consulting firm, was found to have harvested the profiles of over 50 million Facebook users for its psychometric profiling campaign, exploiting a major loophole in Facebook’s API. Allegations that these profiles were then leveraged to influence voting dynamics during the 2016 U.S. presidential election and the UK Brexit referendum, while not conclusively proved, remain deeply worrisome.
Government Services
Government adoption of AI products and services has proceeded with caution due to a variety of factors including regulatory concerns, lack of AI skills and knowledge, data privacy and public surveillance issues, and outdated or insufficient digital infrastructures. Consequently, finding early examples of AI failures in government is tricky but not impossible—the three examples described below, while one is hypothetical and the other two are isolated, raise important questions about the kinds of AI-specific challenges governments will be forced to confront as they modernize.
AI in National Security: In a hypothetical Taiwan crisis scenario—posed by members of the American Statecraft Program—in which the potential impact of deepfake videos on national security was modeled, a group of regional experts who were asked to evaluate and analyze the scenario raised significant concerns. Experts claimed that AI-generated deepfakes could complicate and obscure policymakers’ judgment during critical moments, and even if policymakers were able to classify deepfakes with certainty, accounting for reactive public sentiment, particularly the pressure to react aggressively and defensively, would be extremely difficult. This scenario conceptualizes an AI-specific two-fold challenge (for which there is no current solution) faced by governments: on the one hand, being able to distinguish authentic from fake content is crucial, but on the other, managing public perception in high-stakes scenarios, particularly overreaction, is equally if not more important.
NYC AI Chatbot Failure: In October of 2023, NYC’s local government launched an AI chatbot, called MyCity, intended to provide businesses and residents with accurate and up-to-date information on relevant city laws, regulations, and services. However, upon further investigation, it was discovered that the chatbot frequently provided dangerously inaccurate advice, and in some cases, even advocated for illegal activity across domains like housing policy, worker rights, and business regulations—two notable examples include stating that landlords could discriminate by income source and that employers were entitled to workers’ tips. While NYC’s mayor—Eric Adams—at the time defended the project as a work in progress, sharp criticism on irresponsible AI deployments, specifically the deployment of systems that haven’t been tested and verified for accuracy, will likely hinder further similar initiatives.
UK NHS Covid-19 App: The NHS COVID-19 app, though well-intended, failed severely after numerous delays and malfunctions during its initial development and launch in 2020. The NHS chose to pursue a centralized data collection approach, which proved to be not only technically unfeasible—by contrast to Apple/Google’s successful decentralized contact tracing system—but also ethically and legally dubious. For instance, the NHS app could only detect 4% of nearby iPhones compared to 75% of Android phones, and privacy experts also raised numerous concerns about the potential breach and misuse of sensitive user data, a vulnerability inherent to centralized data collection systems. In June 2020, the UK abandoned the£35 million-plus project in favor of Apple/Google’s system.
Real Estate
The real estate industry is already exploring AI use cases for functions like automating the creation of property descriptions, improving the precision and accuracy of pricing predictions, and screening potential tenants. However, these practices have raised concerns due to examples of incomplete property descriptions, unreliable pricing predictions, poorly designed AI chatbots, and mounting legal and ethical pressures.
Zillow Offers Failure: In 2021, Zillow’s home-buying program, Zillow Offers, failed catastrophically and was shut down after a $500 million loss. Zillow Offers was designed to predict housing prices and execute purchasing decisions, but the algorithm was trained on outdated data despite its intended use in a shifting market. Additional factors that contributed to the failure included an algorithmic inability to account for market shifts coupled with inadequate human oversight and monitoring. The Zillow case illustrates a cautionary tale for businesses relying on AI for critical decision-making—especially if it concerns complex, dynamic markets—stressing the critical need for robust and reliable monitoring systems, frequent model testing, updates, modifications, and improvements, and an overall approach that more carefully balances AI-driven decision-making with human judgment.
AI in Tenant Screening: More recently, landlords have begun leveraging AI to screen for potential tenants—a practice that, although subtle, has received substantial criticism due to its exacerbation of housing discrimination and facilitation of undue barriers to access. These AI-powered screening tools analyze sensitive financial data like credit scores and rental history, and in some cases, even criminal history—these data are typically not well-screened for quality, accuracy, and relevance, which, when coupled with the relative opacity of these tools, raises major concerns about potential discriminatory biases, especially for marginalized groups. Fortunately, several regulatory bodies, including the Consumer Financial Protection Bureau (CFPB) have committed to enforcing civil and consumer rights in these situations, and screening companies are now being forced to confront over 90 federal civil rights and consumer lawsuits.
Healthcare
Even though AI inspires enormous promise within the healthcare sector, challenges with AI-powered diagnostics and health predictions, model bias and discrimination, and a slew of legal and ethical concerns have pushed many stakeholders within the industry to more carefully scrutinize their approaches to GenAI integration initiatives. There are many AI successes within the healthcare sector, but we have much more to learn from the failures we’re beginning to see.
Epic Systems’ AI-Powered Sepsis Prediction: A 2021 study in the JAMA Internal Medicine journal discovered that Epic Systems' AI-powered sepsis prediction model (ESM) fell disappointingly short of its claimed prediction accuracy rate—true accuracy was revealed to be 63% compared to Epic’s claimed accuracy of 76%-83%. In fact, the system outright failed to predict two-thirds of sepsis cases, generating numerous false alarms that hindered clinicians' ability to administer accurate diagnoses in life-threatening situations, causing “alert fatigue.” Further research in 2023 and 2024 raised additional concerns, demonstrating that the model would frequently make predictions after clinicians had already recognized sepsis and that in cases where the data required to meet sepsis criteria was restricted or incomplete, model accuracy fell by a significant margin.
IBM Watson for Oncology: IBM’s Watson for Oncology (WFO), an AI system that was designed to revolutionize cancer treatment, faced backlash after a 2017 investigation revealed that it had made faulty and unsafe recommendations for various cancer treatments. Further investigation showcased the system’s inability to interpret nuanced patient cases, its tendency to provide recommendations that weren’t aligned with clinical best practices, and its general unreliability across different global contexts. Ultimately, these problems were attributed to factors like poor data quality, cancer treatment complexity, and the struggle to account for diverse patient populations and profiles as well as different healthcare infrastructures. All of these factors culminated in the partial sale of Watson Health in 2022.
Conclusion
Now that we’ve created some context for our future discussions, we leave readers with a series of takeaways to consider as we move forward. The takeaways listed below represent our attempt to extrapolate key insights from the examples we’ve illustrated in this piece, though we nonetheless challenge readers to continue broadening their perspective on these issues and discovering insights that we haven’t covered here.
-
Building good AI products and services requires patience. It’s tempting to fast-track AI development to remain competitive but rushed AI efforts are far more likely to fail, even if the intention and idea behind the product is positive and valuable.
-
The AI products and services that tend to work best are those that have access to large volumes of high-quality data. If you’re reading this post, this is probably already obvious to you, though the examples we’ve highlighted drive this point home quite strongly.
-
The most severe AI failures appear to occur with large companies or organizations, possibly due to competitive pressures with other major industry players. This isn’t to say that smaller companies developing AI products are more likely to succeed—it’s also possible large company AI failures simply receive more media attention, creating the illusion of a higher failure rate.
-
AI needs to be tested in the real world, or at the very least, in safe and controlled environments like regulatory sandboxes. An AI model can work extremely well in theory, but humans and their environments are constantly changing, and building systems that can adapt to this change still represents a major challenge within the AI industry.
-
Aim high, but assume things won’t work out as planned. AI projects may have a high failure rate, but that doesn’t mean they shouldn’t be pursued, especially if they could afford scalable benefits across critical domains like healthcare, housing, and education.
-
Don’t let others tell you where AI does and doesn’t belong. Of course, this should be taken with a grain of salt, as any context in which AI could inspire significant harm should be heavily scrutinized from multiple perspectives. In terms of benefits, however, AI can do a lot of good, and oftentimes, this is in the places we least expect.
-
Prioritize responsible AI (RAI) development. If we could write this a million times, we would—even if readers ignore everything we’ve talked about thus far, they should internalize this point. RAI will define the boundaries of AI innovation both legally and socially, and if we want to keep pushing this technology forward and leveraging it to tackle the world’s most pressing problems, it is imperative that it remains safe and trustworthy.
Our next piece will be future-oriented, detailing and breaking down predictions about the near future of GenAI. For readers interested in exploring additional topics in the GenAI space, alongside others related to RAI, governance, and risk management, we suggest following Lumenova AI’s blog.
For those who are considering or initiating the implementation of AI governance and risk management protocols, we invite you to check out Lumenova’s RAI platform and book a product demo today. For those who prefer an initial conversational approach, our AI Policy Analyzer may also be of interest.