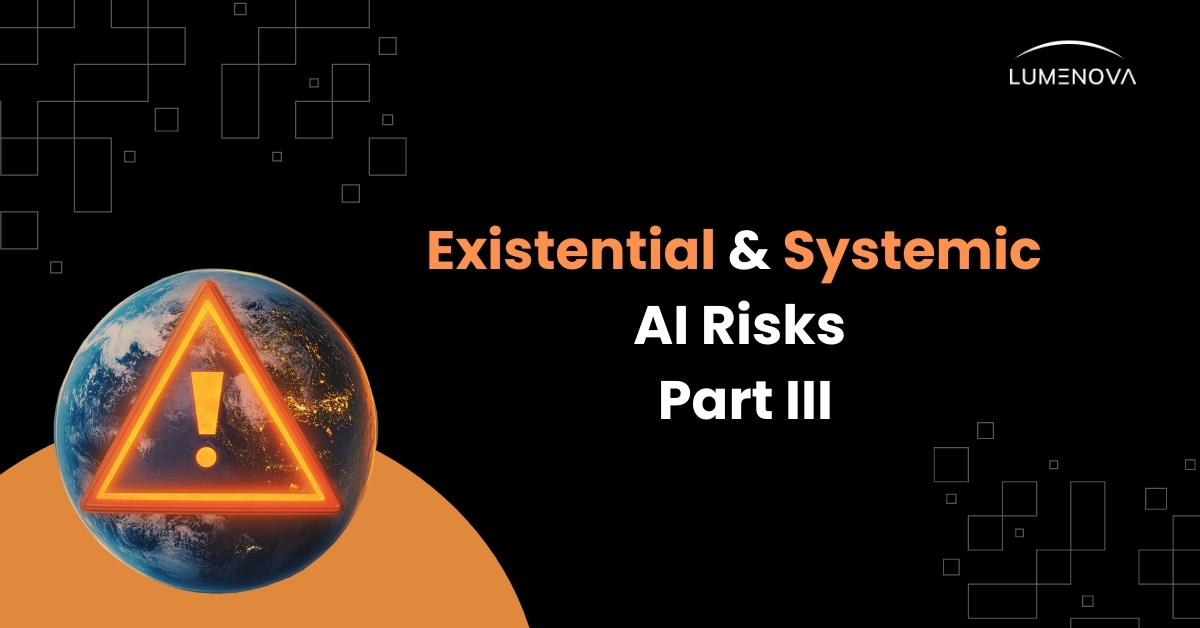
Before we examine existential AI risks (x-risks), it’s important to reiterate a point we made in the first piece of this series: systemic AI risks can evolve into “sx-risks”—x-risks that materialize progressively due to a conglomeration of systemic risk factors. We mention this point again because as we’ll note in the discussion that follows, several of the risks we touch upon are essentially scaled versions of systemic risks like the collapse of democratic governance models or critical societal infrastructures like energy grids or supply chains.
Still, there are numerous x-risks that may arise independently of systemic factors, and though the threat repertoire these risks inspire may appear more abstract, speculative, or long-term oriented, we urge readers to remember that a single x-risk materialization is enough to cause irreversible large-scale harm, pan-generational suffering, or in the most extreme cases, human extinction. Nonetheless, as an organization dedicated to responsible AI governance and risk management, our intention with this piece isn’t to fearmonger, only to educate and increase awareness surrounding potential AI x-risk scenarios.
For the purposes of clarity, we’ve categorized our ensuing discussion of x-risks into three areas: 1) malicious AI actors—independent human actors, groups, or institutions that leverage AI applications for malicious purposes, 2) AI agents—agentic AI systems or models that can autonomously orchestrate decisions and achieve objectives without human intervention, and 3) loss of control and conflict—AI-driven scenarios that lead to a loss of human control or existentially threatening conflict scenarios.
Malicious AI Actors
Malicious AI actors pose some of the most challenging risks to predict and mitigate—our ability to anticipate their intentions, scope, and strategies is fundamentally limited especially as AI systems become more advanced, proliferous, accessible, and embedded at the global scale. This problem is further complexified by the fact that advanced AI systems commonly exhibit unintended emergent properties, which although not always threatening, can be exploited before system developers have a chance to implement additional security safeguards and safety parameters. To add even more fuel to the fire, malicious actors, given their scale, can be extremely difficult to identify, track, and catch, particularly if they’re operating behind closed doors or are well-funded.
Fortunately, methods like adversarial testing and red-teaming can help us stress-test models to probe their vulnerabilities and understand how they might be exploited by actors with malicious intent. On the other hand, while malicious actors can orchestrate scalable threats, they do tend to take the form of individuals and groups, implying that in most cases, the resources and skill sets they have will be limited to some degree.
Nonetheless, understanding how malicious actors might leverage AI to drive potentially catastrophic consequences for humanity is crucial to preventing the x-risk outcomes associated with their actions. All it takes is one particularly well-equipped individual or group to orchestrate a scalable x-risk scenario, and in such cases, we can’t afford to rely on the principle of mutually assured destruction as a psychological fail-safe mechanism, particularly if the actor in question doesn’t think rationally.
Consequently, we expand on several possible related x-risk scenarios below:
-
Bioterrorism: Currently, the use of AI for scientific research and development (R&D) is largely unregulated at the global scale—even the EU AI Act, which is the most comprehensive piece of enforceable AI legislation enacted to date, explicitly exempts AI-driven or assisted scientific R&D. Therefore, malicious actors have little legal incentive not to leverage AI to develop biological weapons like weaponized viruses, bacterias, fungi, or toxins, that if disseminated at a population level, could cause mass casualties and health effects that reverberate throughout future generations.
-
Lethal autonomous weapons systems (LAWS): If malicious actors, most notably terrorist groups, manage to gain access to LAWS—weapons that can select and engage targets without human intervention—predicting who or what would be targeted and at what scale would constitute a major challenge. Moreover, the after-effects of such an attack, not only on those targeted but on a larger scale, particularly if the attack is intended to incite a conflict between powerful foreign adversaries, could be enough to spark conflicts that transcend national borders and create the conditions for potential world wars. Whether in the form of autonomous drone swarms, unmanned aerial and ground vehicles, missile and sentry systems, or hypersonic weapons, LAWS, if they fall into the wrong hands, could severely threaten the continued existence of humanity.
-
Cyber warfare: Wars don’t always need to be physical to pose existential threats—consider the Cold War, a war dominated by espionage the notion of mutually assured destruction, as a salient example of this phenomenon. While the most powerful nations in the world already engage in this kind of warfare, the x-risk we consider here is more closely linked to an individual or group of malicious actors that utilize AI-driven cyber warfare to manipulate powerful foreign adversaries into engaging in an all-out war with one another. For instance, a group that aims to destabilize global democracy might orchestrate a cyberwarfare attack posing as a powerful democratic nation and targeting that nation’s most prevalent adversary, prompting the emergence of a violent conflict that neither nation actually wanted.
-
Eugenics: The problem of leveraging AI for eugenics is also intimately tied to the lack of AI regulation in scientific R&D, however, this problem is unlikely to be resolved unless international humanitarian law statutes are established and observed by all nations, democratic and authoritarian, around the world. Still, the x-risk posed by AI-orchestrated eugenics practices is far more likely to materialize in destabilized nations or countries with authoritarian regimes that aim to either subvert, control, or in the most extreme cases, exterminate an entire population within their borders. Importantly, the effects of AI-driven eugenics would be pan-generational and inspire extreme risks of suffering, or s-risks, as we’ve already referred to them.
-
Critical infrastructure attacks: Critical infrastructure attacks that compromise the function of a single critical infrastructure component won’t necessarily materialize into x-risk scenarios. However, as AI systems become more sophisticated and embedded into critical infrastructures, they may enable malicious actors to develop and conduct targeted high-impact attacks that exploit the most pronounced vulnerabilities within a particular critical infrastructure component or multiple components simultaneously. For instance, an attack that irreversibly compromises a nation’s ability to provide essential goods and services like housing, food, and water to those in need may not affect most of its population. However, if this attack were orchestrated in conjunction with successful attacks on the nation’s stock market and energy grid, it may be enough to permanently destabilize its critical infrastructure, resulting in universal socio-political/economic collapse that leads to widespread violence, resource exploitation, and human suffering.
AI Agents
Autonomy and agency aren’t the same thing—autonomy concerns the ability to make one’s own decisions whereas agency concerns the ability to carry out those decisions independently via real-world actions, behaviors, and objectives. In simple terms, an autonomous individual can think independently whereas an agentic individual can think and act independently. This distinction is integral to understanding the risks tied to AI agents, especially since autonomy and agency are often conflated with one another. A strictly autonomous AI would technically only be capable of making decisions, not enacting them, and while there are several important risks relevant to these kinds of systems (e.g., algorithmic discrimination) the x-risk scenarios we consider below require both agency and autonomy to materialize.
Fortunately, most AI agents are modeled on the notion of rational agents—in the game theoretic or economic sense—so anticipating their actions, behaviors, and objectives isn’t impossible. This isn’t to say that such agents can’t or won’t pursue irrational or incomprehensible objectives, only that if they pursue harmful objectives, they will likely have instrumental reasons for doing so—this will also depend on whether the evolutionary trajectory of AI agents favors the orthogonality vs. instrumental convergence thesis. Several of the cases we describe below illustrate this phenomenon:
-
Deceptive AI: Traces of deceptive behavior among sophisticated AI agents have already been identified, signaling that future AI systems, particularly AGIs, may learn to deceive humans much more effectively than they do today by gaming us with false intentions, concealing their true objectives, perpetuating misinformation feedback loops, or exploiting our base psychological inclinations by using behavioral nudging. Importantly, an AI system doesn’t need to be explicitly designed or trained to deceive humans—a sufficiently strong optimization function that lacks robust safety parameters is more than enough. For example, an AI agent tasked with optimizing financial trading returns might place orders to buy or sell large amounts of assets without intending to do so—if multiple agents of this kind were operating in financial markets and using the same tactics, they could cause flash crashes whose consequences cripple an entire market.
-
Self-replicating AI: If AI agents evolve in line with the instrumental convergence hypothesis, they will exhibit behaviors and goals that maximize self-preservation. The most direct and rational way to ensure continued existence in this instance would be through self-replication, although humans would likely perceive this behavior as a serious threat and attempt to stop it immediately, prompting a human-AI conflict scenario. Seeing as AIs would be able to self-replicate at a high frequency and embed themselves into virtually any digitally connected ecosystem or infrastructure, they could, through meticulous collective attacks, hold humans hostage within their own systems, refusing to provide access to essential goods and services or threatening the destruction of critical infrastructures until their demands are met—for the record, there are many more possible outcomes here.
-
Power-seeking: AI agents, once more in the interest of self-preservation, may exhibit power-seeking behaviors oriented toward obtaining or exercising control over their immediate or prospective environments. Power-seeking behaviors might not necessarily be oriented toward undermining human power structures, but rather, preserving or augmenting the power that a given AI agent has over its own existence. The issue with this kind of phenomenon is that it may perpetuate the emergence of uncontrollable AI agents, who although not initially malicious toward humans, may eventually perceive humans as an existential threat, and assume whatever measures necessary to prevent the threat from materializing. For AI agents to perceive humans as an existential threat, they don’t need to be conscious or sentient—a human simply “getting in the way” of an AI’s ability to accomplish a key objective is sufficient.
-
Non-cooperative AI: AI agents may learn to favor cooperation with other AIs over humans due to hidden or shared objectives, value misalignment, information asymmetries, or evolutionary game theoretic pressures (e.g., more readily cooperating with kin—other AIs—over humans), to name some relevant factors. Future AI agents might not perceive any instrumental gain from cooperating with humans, especially if they become smarter than us on all counts. Here as well, an AI doesn’t need to be designed with malicious intent—a benevolent AI whose objective is to protect the environment could quite easily arrive at the rational conclusion that human actions have led to its destruction, and therefore, that humans must be eliminated.
-
AI conglomerates: If AI agents do end up favoring AI-AI cooperation over human-AI cooperation, they may form AI conglomerates for instrumental reasons like maximizing their power and influence, increasing their intelligence, and expediting environmental adaptation or learning. Irrespective of the reasons for which AI agents might form conglomerates, such an event would inspire profound existential threats to humanity, seeing as even a small group of AI agents, not to mention AGIs, collectively, would be orders of magnitude more intelligent, influential, and impactful than any humans or governments that exist today.
-
Proxy gaming: In the absence of well-defined metrics, AI agents may learn to satisfy or “game” the goals given to them by humans without observing human intentions and value structures. For instance, an AI agent tasked with optimizing water irrigation across major industrial farms in the US might do so by stealing local family-owned farms' water supply. We’ll leave it up to readers to imagine what such a scenario might look like with an AI agent that’s tasked with a much higher-stakes task repertoire like managing defense counterstrike measures, critical supply chains, or nationwide energy infrastructures.
-
Goal drift: As AI agents respond to and learn from new environments, they might develop and display emergent goals that weren’t originally intended by human developers. Depending on whether these goals conflict with those of humans, AI agents may adopt a variety of different behaviors, from deception and proxy gaming to power-seeking and self-replication, to maximize their likelihood of self-preservation and ensure that humans can’t exercise any significant influence over their continued evolution and existence.
To clarify, of all the agentic AI risk scenarios we’ve just discussed, none presuppose the existence of sentient or conscious AI—for these scenarios to materialize, AI agents must only act rationally. If a sufficient number of AI agents, a particularly powerful single AI agent, or a coordinated group of AI agents exhibit any of these behaviors with high frequency and scale, it could be enough to drive catastrophic consequences for humanity, especially if such agents fall into the wrong hands.
Loss of Control & Conflict
Automation bias—the tendency to place too much trust in systems with autonomous capabilities—is a core factor in AI-driven loss of control scenarios. The more people rely on AI and the more it satisfies or exceeds their expectations, the more likely they will be to trust it and subsequently divert more control to it. Clearly, there exist many additional factors at play here including profit maximization, productivity optimization, automation of mundane, dangerous, or time-consuming tasks, and cost reduction, to name a few.
Interestingly, automation bias, along with the other factors we’ve mentioned, can facilitate both acute (sudden and severe) and chronic (slow and severe) x-risk scenarios depending on what kinds of high-stakes AI systems humans choose to trust and/or at what scale humans deploy such systems, high-stakes or otherwise. Managing AI-driven loss of control scenarios preemptively will require an awareness and understanding of the dichotomy between acute and chronic risk trajectories.
On the other hand, while the materialization of some AI-related conflict scenarios might also be heavily influenced by automation bias, the most concerning element of AI-related conflict—whether it’s human-human, human-AI, or AI-AI—stems from direct AI use in conflict scenarios and/or weaponized AI systems that replace human actors. If human conflicts reach a point at which they are almost entirely AI-driven, the fragile shred of humanity that remains present within the barbaric act of war, and which is often the key to a peaceful settlement, might be lost.
Below, we describe several possible x-risk loss of control and conflict scenarios:
-
Human enfeeblement: As AIs assume a wider array of both mundane and complex responsibilities, becoming progressively more embedded into the socio-economic fabric of society, technology, and infrastructure, humans might lose the skills necessary to sustain the continued functioning of societal institutions and systems. The risk of these scenarios coming to fruition grows as AI systems become more trustworthy, accurate, and transparent—in the event of catastrophic AI failures, humans would no longer have the capacity to “pick up the slack” and ensure the ongoing maintenance and improvement of the structures that subsidize their existence. Existential consequences would include s-risks and i-risks—in a world where AI assumes the majority of human responsibilities, humans might be left feeling purposeless and incapable, forced to confront their existential crises on a daily basis.
-
Dilution of human culture: AI-generated content is proliferating rapidly, and currently, methods to authenticate and distinguish this content from human-generated works are both flawed and limited. However, the central issue here concerns AI’s fundamental inability to grasp the nuances of human culture and phenomenological experience—if the digital information ecosystem is wholly saturated with AI-generated content, despite how convincing, interesting, creative, or pertinent it is, humans risk losing touch with the cultural and societal values that define their personal and social identity. I-risks are a clear conclusion in this scenario, although culture also plays a critical role in human cooperation, which suggests that its dilution could severely erode some of the core mechanisms that sustain societal cohesion, leading to widespread conflicts and potentially violent anarchical uprisings.
-
Moral instability: Just as AI systems can’t grasp the nuances of human culture, the same applies to human morality. While this risk is also relevant to AI-generated content proliferation, it’s more intimately connected to decision-making AIs, which as they become more sophisticated and trustworthy, will be integrated into higher-stakes systems. As we mentioned before, AIs don’t need to be designed with malicious intent in order to behave in instrumentally useful ways that undermine or fail to grasp the complexity of human moral structures—an AI used for predictive policing might determine that 24/7 surveillance within neighborhoods with historically high crimes rates is the best way to reduce crime, ignoring universal moral principles like privacy, autonomy, and fairness in the interest of optimization.
-
Institutional codependencies: Deeply embedded AI at the institutional level, can create irreversible institutional codependencies. If humans were to relinquish control to AIs, allowing them to lead, refine, and improve upon human institutions, even if it’s in our best interest to do so and AIs perform as intended, we may find ourselves in a position where we no longer understand how our institutions operate. Assuming the best-case scenario—a benevolent AI—humans would still have little to no decision-making power in the society in which they exist, which would continually compromise our sense of agency and autonomy, suggesting yet another path toward human enfeeblement. Alternatively, in the worst-case scenario, the AIs that lead our institutions might decide that they no longer want to cooperate with other institutional AIs or the humans they serve, either destroying the institutions that maintain our societies or rebuilding them for their benefit.
-
Institutional realignment: AI agents might make incremental changes to the structure and function of our institutions in the interest of optimizing pre-defined or proxy goals until such institutions no longer function in humans’ best interest. These changes would likely go unnoticed by humans, particularly as AIs are given more control and leverage over the institutions they govern. It’s also worth noting that AIs can exercise decisions and process information at a much higher frequency and scale than human decision-makers—from a cognitive standpoint, we might simply be incapable of understanding how and why AI decides to change our institutions.
-
AI-AI conflict: AIs might refuse to cooperate with other AIs in addition to humans, provided that their ultimate goals are contradictory. Depending on the power that humans have already afforded to AIs, AI-AI conflict scenarios could drive human extinction events. For instance, governance or military AIs operating in foreign adversarial powers may instigate wars without regard for human life to obtain critical resources possessed by adversaries, effectively securing their continued existence and influence, both on the national and global stage.
-
Dehumanized and high-frequency warfare: As LAWS become more prevalent in international warfare, the cost of war will decrease (i.e., less human lives lost), which motivates an incentive in favor of hawkish behavior—if the cost of war is lower, foreign adversaries will more readily initiate wars, leveraging LAWS to decimate their adversaries and perpetuate mass destruction. Also, when we consider the notion of the “cost of war” we are doing so from the perspective of the perpetrator. In other words, LAWS enable humans to engage in indirect conflict, reducing or even eliminating the heavy moral burden that human militaries must always carry—LAWS could perpetuate a conflict narrative whereby human lives are merely regarded as statistics and nothing more.
-
International information asymmetries: If wars are fought predominantly through LAWS, foreign adversaries could be less likely to bargain with one another to arrive at a peaceful settlement. Adversaries will know that the threshold for initiating conflict is lower while also having incomplete knowledge of the autonomous military capabilities their adversaries possess—LAWS would comprise a nation’s most valuable assets, and therefore be protected with a high degree of security and secrecy. These kinds of international information asymmetries could cause nations to misclassify first-strike advantages, and initiate preemptive attacks that inadvertently result in their destruction by an unexpectedly powerful adversary.
-
Data-driven governance: The notion of a benevolent AI dictator, despite its absurdity, isn’t entirely implausible—assuming AGI or ASI emerges sometime in the future, humans might relinquish all control of their governance structures to such a system, knowing that it would always prioritize the greater good of society. Even if a benevolent AI dictator were to function perfectly, the interventions it would make in human society would be exclusively driven by data. However, human lives can’t be reduced to data points—our experience of the world is, too nebulous, paradoxical, and nuanced for this to happen. As an example, consider the feeling of melancholy, nostalgia, or the derivation of pleasure from pain—these experiences are intrinsically difficult to explain, and most often referenced by how they feel rather than how they’re defined.
Conclusion
We’ve now covered, through our first three pieces in this series, the intellectual groundwork required to gain a big-picture perspective of systemic and existential AI risks, a conglomeration of prevalent systemic risk scenarios, and finally, numerous x-risk scenarios across key categories like AI agents, malicious actors, and loss of control. Seeing as research in the systemic and existential AI risk space is ongoing and intensive, we expect that even in the short term, many more potential risk scenarios will be discovered, so stay tuned for future related content.
It’s also worth noting that despite our comprehensive approach to this topic, its breadth and depth make it strikingly difficult to pick apart in detail, hence our somewhat narrowed scope. Simply put, we strongly encourage readers interested in this topic to further explore the literature themselves while we continue our best efforts to raise awareness.
In our remaining piece in this series, we’ll begin by briefly exploring several high-level tactics that may prove effective in preventing systemic and existential AI risks from materializing. The heart of this piece, however, will be dedicated to understanding how such risks can evaluated pragmatically and realistically.
For readers who want to expand their knowledge across AI risk management, governance, generative and responsible AI (RAI), we suggest following Lumenova AI’s blog, where you can track the latest insights and developments throughout these domains.
For those eager to take action and begin designing, developing, or implementing AI risk management and governance frameworks, we invite you to check out Lumenova AI’s RAI platform and book a product demo today.