August 29, 2024
Existential and Systemic AI Risks: Prevention and Evaluation
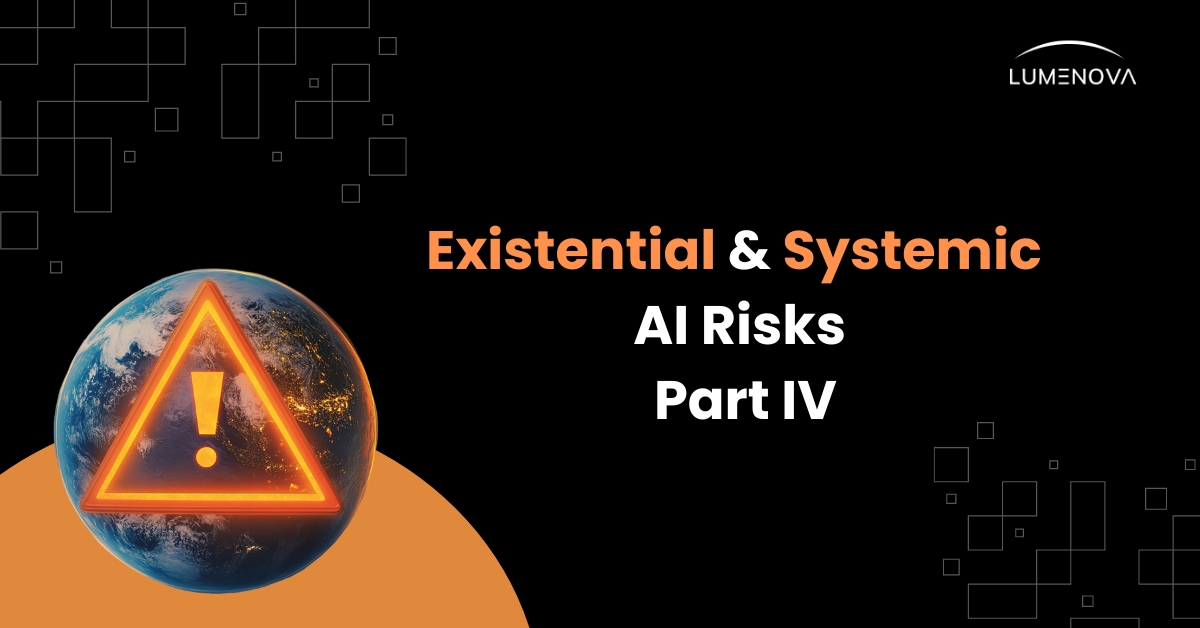
Contents
Currently, the only way to guarantee that systemic and existential (x-risk) AI risk scenarios will never materialize is by abandoning AI entirely, which is both unfeasible and absurd in light of the global benefits this technology could inspire, substantial ongoing investment in AI innovation, and the degree to which AI is already being integrated into all things mundane and sophisticated. Despite what some may think, AI is here to stay, and even if we were to halt AI innovation permanently, today’s most advanced AI systems display capabilities that if exploited or misused, could quite easily fuel profoundly negative systemic and existential outcomes.
While preventing and evaluating AI-driven systemic and x-risk scenarios is admittedly more challenging than preventing and evaluating traditional AI risks, it’s not impossible, nor is it practically unrealistic. Although we may not be able to precisely and accurately predict the probability, impacts, and intractability of many of these risk scenarios—at least to the same degree that we can with some traditional AI risks like algorithmic discrimination—certain high-level preventive tactics and evaluation methods do exist. Such tactics and methods, particularly when they are predicated upon existing AI risk management standards and frameworks, AI safety research and Responsible AI (RAI) developments, and AI policy and regulation, will inform how we think about and manage systemic and x-risk scenarios for the better.
The seed of hope: we’re not starting from ground zero and there’s no shortage of institutions and individuals who have chosen to dedicate their lives to researching, envisioning, and preventing systemic and existential AI risks from materializing.
Consequently, in this fourth and final post in this series, we’ll briefly cover some high-level risk prevention tactics that organizations of all scales can implement in this respect, followed by a series of simple visualizations we’ve created to help our readers understand how they might evaluate the risks discussed thus far.
For readers who are just now being introduced to this subject, crave a more detailed understanding of AI-driven systemic and x-risks, or wish to better comprehend the foundation of this discussion, we strongly recommend that you begin with the first post and this series, and move forward from there.
Risk Prevention Tactics
Systemic and existential AI risks, due to their large-scale scope, speculative underlying assumptions, and complex prolonged materialization dynamics, aren’t afforded much attention outside the academic and research-oriented AI safety space—some exceptions for systemic (e.g., spread of AI-generated misinformation, algorithmic bias, deepfakes, etc.) risks in existing AI legislations like the EU AI Act or Executive Order on Safe, Secure and Trustworthy AI are present. However, If these kinds of risks aren’t brought to the forefront of the public AI safety discourse soon, we may find that several of the conditions required for them to elapse have already been met.
Fortunately, individuals, organizations, and institutions alike, through moderately low-effort interventions, can implement relatively simple high-level tactics aimed at preventing systemic and existential risks from materializing. We briefly describe some of these tactics below:
- Cultivate AI literacy and awareness to ensure that all who leverage or are affected by AI are aware of the systemic and existential risks the technology can perpetuate, its existing limitations and benefits, and its intended use and purpose in both narrow and broad task domains.
- Assume that catastrophic AI failures will happen and that humans will have to maintain the skills necessary to overcome these failures when they occur.
- Ensure that nuanced moral and ethical decision-making remains driven by human decision-makers and that AIs are never allowed to make such decisions autonomously on our behalf, even if they are in our best interest.
- Do not allow AI agents to obtain control of critical infrastructure, weapons systems, institutional functions, or governance practices, irrespective of how trustworthy, benevolent, or accurate their predictions, recommendations, or decisions are.
- Implement incorruptible fail-safe mechanisms in high-stakes AI systems that can immediately shut off a system if it begins showing signs of deception, manipulation, coercion, non-cooperation, malicious intention, or corruption by an external actor.
- Implement AI systems that are redundant by design to limit the possible number of system failure modes and ensure that in the event of an AI failure, normal operations can continue unobstructed.
- Consider that AI agents might pursue instrumental emergent goals that align with an optimization function or the need for self-preservation without recognizing that the behaviors required to achieve such goals may be detrimental or catastrophic for human well-being and existence.
- Only implement AI where it’s absolutely necessary to avoid unintentionally manifesting deeply engrained dependencies or reliances that, in the event of a catastrophic AI failure, irreversibly compromise the entire functioning of a system.
- Think beyond industry-specific AI risks and examine the peripheral consequences of potential risk scenarios to understand which kinds of risks might evolve into systemic or x-risks, or alternatively, how a conglomeration of risks might come together to perpetuate a systemic or x-risk outcome.
- Simulate long-term AI crises or catastrophic scenarios by conducting in-depth scenario planning exercises that range far beyond typical business or regulatory planning horizons. Such exercises should examine, in detail, best and worst-case outcomes, with due consideration for multi-faceted events, changing environments, and complex intertwined factors that could lead to systemic and x-risks.
- Explore how other institutions, organizations, governments, and nations are approaching AI-driven systemic and x-risk scenarios, paying especially close attention to how they’ve designed, developed, and deployed various AI systems and for what purposes, respectively.
- Encourage interdisciplinary collaboration with diverse AI actors including developers, ethicists, economists, sociologists, lawmakers, academics, and other relevant stakeholders, to foster a comprehensive understanding of potential risks and impacts, most notably blind spots in risk mitigation strategies.
- Regularly and transparently engage in public and stakeholder dialogue to avoid AI risk “tunnel vision”, and via public consultations, workshops, and forums, gain a real-world perspective on how systemic and existential AI risks are perceived, understood, and managed where appropriate.
Common risk management methods like continuous monitoring, accountability, transparency and human oversight, risk mitigation planning, identification, and prioritization, serious incident reporting and remediation, adversarial testing and red-teaming, and the establishment of AI governance protocols, for example, should be implemented and followed in tandem with the above tactics. In other words, adherence to state-of-the-art AI risk management standards and frameworks like the NIST AI RMF or ISO 42001, along with other existing risk-centric AI policies, regulations, standards, and benchmarks will form the foundation of any organization’s ability to manage AI risks responsibly and effectively, regardless of their scale or complexity.
Evaluating Risks
This section presents several visual figures that will help readers broadly understand how they might evaluate both systemic and existential AI risk scenarios. Importantly, these figures, while not directly based on the figures in Nick Bostrom’s 2013 paper on existential risk, have drawn significant influence and inspiration from them. Each figure will be accompanied by a brief explanation as to what it entails, and we’ll begin with systemic risks followed by x-risks.
We also note that by mentioning certain risks—covered in parts two and three of this series—in these figures, we are not implying real-world probability, impact, awareness, severity, and intractability measures, but rather, leveraging these risks as examples to provide our readers with something tangible to work with. Nonetheless, it’s crucial to highlight what the aforementioned measures mean:
- Probability: The percentage likelihood that a given risk scenario will occur.
- Impact: The raw number of persons or proportion of a localized (systemic risk) vs. global (x-risk) population that will be affected if the risk materializes.
- Awareness: The percentage of persons within a population who know about a potential x-risk they are likely to be affected by.
- Severity: For systemic risks, the product of the probability and impact estimates. For x-risks, the product of the probability, impact, and awareness estimates.
- Intensity: The rate, measured in percentage of a year, at which a given risk scenario may materialize.
- Intractability: The difficulty with which a potential risk scenario could be mitigated—the product of severity and intensity.
Systemic Risks
Figures 1 & 1a description: Both these figures illustrate the probability and impact of a systemic risk should it materialize. We’ve created two versions of this figure because systemic risks can operate at different scales, especially within disparate nations. Therefore, in Figure 1, we illustrate impacts according to the raw number of total affected persons whereas in Figure 1a, we illustrate impacts according to the percentage of persons affected within a given population. Nonetheless, these two Figures are identical in what they represent.
Figure 2 description: This figure depicts the probability that a given systemic risk will occur with respect to its intensity—the timescale on which it’s likely to materialize.
Figures 3 & 3a description: Figure 3 describes how to calculate both the severity and intractability of a given systemic risk whereas Figure 3a illustrates the probability of a given systemic risk materializing with respect to its intractability—how difficult it is to mitigate.
Existential Risk
Figure 4 description: This figure depicts the probability that a given existential risk will occur with respect to its intensity—the timescale on which it’s likely to materialize.
Figure 5 description: Similarly to Figures 1 & 1a, Figure 5 illustrates the probability that a given existential risk will materialize with respect to the scale of its impact. Importantly, impact for existential risk is measured in the proportion of the global population instead of the raw number of affected persons or the proportion of a localized population because existential risks operate on a much larger scale than systemic risks.
Figure 6 description: This figure illustrates the probability that a given existential risk will materialize with respect to the proportion of persons who are aware of this risk in the affected population. We have chosen to include awareness as a measure for existential risk mitigation because these kinds of risks are not frequently or accurately discussed in the mainstream.
Figure 7 description: This figure describes how to calculate the severity and intractability of an existential risk.
Conclusion
In wrapping up this series, we urge readers to take systemic and existential AI risks seriously because ultimately, if any of the scenarios—particularly x-risks—we’ve described in our previous pieces occur, significant harm, whether localized, large-scale, or pan-generational will be imminent. We also remind readers that x-risk scenarios imply irreversible consequences.
In line with our dedication to ensuring a future in which AI is developed and deployed responsibly, profitably, and for the benefit of society and humanity, we here at Lumenova AI believe that this discussion must be made accessible to businesses, lawmakers, ethicists, and civil society at large. However, accessibility is only part of the solution, and looking ahead, we need to continue thinking pragmatically about these risks to prevent them from materializing in the real world.
In the spirit of this pragmatic approach, we leave readers with a final thought on existential risk: the Maxipok rule—maximizing the probability of an ‘ok’ outcome—coined by Bostrom, which suggests that humans should prioritize proactively managing catastrophic outcomes that may result in x-risk scenarios. The key implications of this rule can be broken down into four parts:
- Existential Risk Prioritization: AI-driven scenarios that could feasibly result in the extinction of humanity, or similarly, large-scale pan-generational harm that puts the continued survival of our species in question, must be prioritized.
- Safety: Decision-making actions aimed at minimizing the probability of AI-driven catastrophic outcomes for humanity must be taken, even if they result in the foregoing of short-term gains or benefits, irrespective of their scale and impact.
- Long-term Orientation: The safety and security of humanity’s future in the long term must be prioritized over immediate concerns. Precautionary x-risk measures must be established and implemented to minimize the risk of catastrophic outcomes, particularly for high-impact globally accessible technologies like AI.
- Global Cooperation: Effectively managing AI-driven x-risk scenarios will more likely than not require global cooperation, solidarity, and investment, especially as it concerns preserving the prosperity of future generations.
For readers interested in further exploring the AI risk management and governance landscapes, alongside additional topics in generative AI and RAI, we recommend following Lumenova AI’s blog, where you can track the latest insights and developments across these fields.
For those who are taking concrete AI governance and risk management efforts, whether in the form of internal policies, protocols, or benchmarks, we invite you to check out Lumenova AI’s RAI platform and book a product demo today.
Existential and Systemic AI Risks Series
Existential and Systemic AI Risks: A Brief Introduction
Existential and Systemic AI Risks: Systemic Risks
Existential and Systemic AI Risks: Existential Risks
Existential and Systemic AI Risks: Prevention and Evaluation