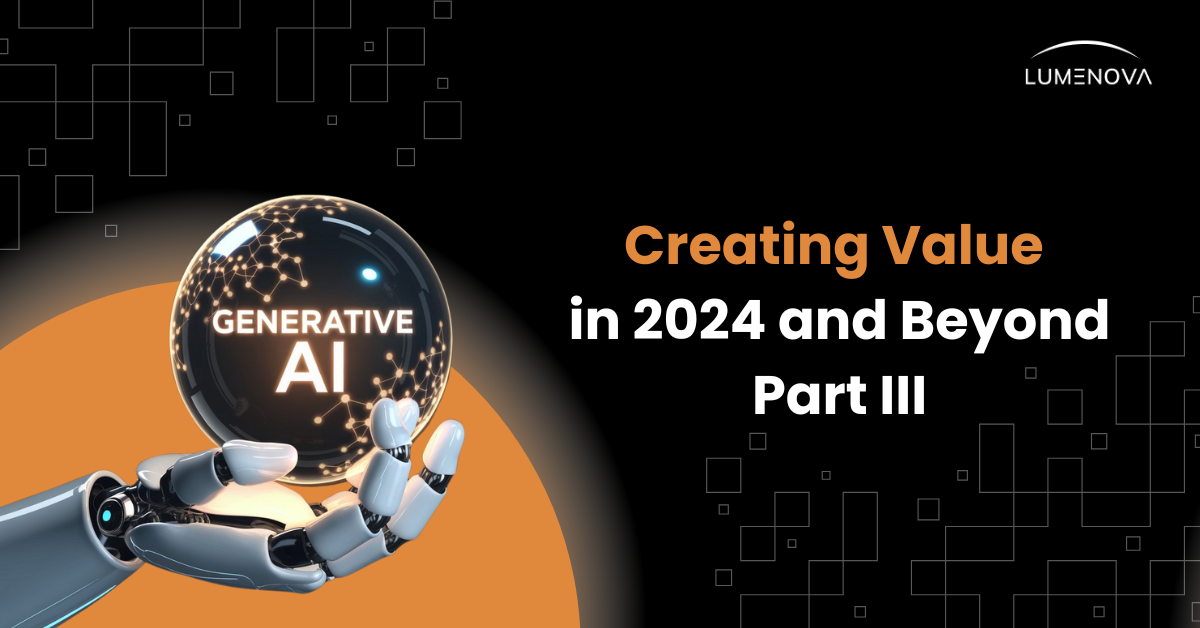
Contents
Accountability and Innovation: The Relationship
Responsible AI (RAI) innovation can’t happen without accountability. Accountability, however, doesn’t only extend to AI companies, developers, research hubs, and policymakers but also to users, who form a major cornerstone of the AI lifecycle. Why? Because, any good product or service must be viable in the real world, which includes the ability to scale safely and efficiently, perform reliably throughout changing environments, and continue delivering value and utility within intended and expanded use contexts. User interactions with deployed AI tools, applications, and features will significantly shape and set the parameters for AI innovation, especially where they provide product feedback or leverage AI in ways that don’t correspond with its intended purpose and use.
Moreover, those who actively use AI are accountable for how they use it. The key term here is “active”—a person who knowingly and willfully uses AI vs. someone who is benefitting from (or being harmed by) AI without being explicitly aware of it. For example, last year, a lawyer leveraged ChatGPT to prepare a court filing, referencing several non-existent court cases during litigation only to discover that ChatGPT had hallucinated these cases when the defendant’s legal counsel was unable to verify their existence. A few weeks later, the lawyer, and his partner, were sanctioned by a New York district judge.
By contrast, there are many instances in which users benefit from AI without explicit awareness—social networks, online shopping, and streaming are all great examples of this phenomenon. Whether you’re navigating a platform like Spotify, Amazon, or Linkedin, content curation algorithms allow you to benefit from a personalized and targeted digital experience that tends to reflect your true preferences and interests quite well, sometimes, even before you are aware of them. Importantly, these experiences almost always come at a considerable covert cost, most notably user privacy.
On the other hand, the notion of an active user also raises a critical question: how will the threshold for what constitutes an active user—someone who is accountable for their use of AI—change over the coming years? A decade ago, reposting a piece of social media content that spread disinformation might have been excusable, but today, as disinformation awareness and concerns continue to grow, are users still the victims of disinformation or are they becoming its key perpetrators? This same logic applies to AI, particularly as the technology becomes more widespread, advanced, and accessible in professional settings where performance and competitive pressures remain steady.
Returning to the lawyer’s case, the judge found his arguments unconvincing because as a lawyer, he was held to a certain standard of due diligence, which he failed to meet by not vetting AI-generated legal content appropriately. The lawyer’s excuse that his understanding of ChatGPT was limited to a “super search engine” is akin to a doctor prescribing a medication whose function and side effects they don’t fully understand only to deny accountability when the patient is harmed.
While the impacts of such cases are not yet obvious at the systemic scale, make no mistake that they will be—legal precedents for user accountability in the age of AI will be set while AI companies simultaneously adapt, modify, and improve their systems in response to real-world use cases (and user feedback), especially those that inspire controversy or receive media attention. This is precisely where user accountability meets AI innovation, and this intersection is likely to manifest as a multi-directional iterative feedback loop:
Example 1 (User Misuse): User leverages AI inappropriately → new legal precedent is defined → AI company refines product according to legal precedent → user misuses product again but differently → legal precedent is adapted → company refines product, etc.
Example 2 (Legal Precedent First): Legal precedent is defined → AI company violates legal precedent → user is harmed and AI company is fined → AI company modifies product to comply with legal precedent → legal precedent becomes more strict in light of other violations → AI company violates legal precedent, etc.
Example 3 (Voluntary Compliance): AI company complies with voluntary ethics and safety standards → multiple users violate standards, causing widespread harm → legal precedent is set to prevent further violation → AI company voluntarily complies with updated ethics and safety standards, etc.
Taking a step back, It’s also worth conceptualizing the AI innovation-accountability relationship more broadly to showcase how many disparate actors are engaged in the innovation process and what they’re responsible for—innovation can’t happen in a vacuum. Below, we’ve highlighted, in order and abstractly, each stage of innovation coupled with a brief description of the core actors involved and their corresponding responsibilities:
- Idea Generation: Identifying a potential opportunity or solution to a problem as an actionable idea.
Responsibilities: Research, market, and trend analysis, customer feedback, and brainstorming.
Key Actors: R&D teams, product managers, marketing teams, and intended end users.
- Idea Screening & Evaluation: Assessing the feasibility, impacts, and alignment of the idea with organizational goals and values.
Responsibilities: Categorization and prioritization of ideas based on feasibility, impacts, and alignment with organizational goals and values.
Key Actors: Executive suite & senior leadership, financial teams, technical experts.
- Concept Development: Operationalizing the idea concretely, in the form of a concept or prototype.
Responsibilities: Viable concepts and prototypes are created, outlining product features, target audience, value proposition, and overall functionality.
Key Actors: Engineering, marketing, legal, and design teams.
- Risk Assessment & Feasibility: Conducting a technical, financial, and operational evaluation of the risks linked to the innovation.
Responsibilities: Risk, cost, and feasibility analysis, and resource planning.
Key Actors: Engineering, risk management, finance, and legal teams.
- Development: Moving the idea from a concept or prototype to a working product or service.
Responsibilities: Iteratively test and refine prototypes to build a minimum viable product (MVP).
Key Actors: Engineering and design teams, product managers, and selected testers (could also be end users).
- Testing & Validation: Validating the innovation’s value and utility through real-world testing with users and/or customers.
Responsibilities: Test MVP in the real world with users via beta testing or pilot programs, then refine MVP accordingly. Evaluate MVP for compliance with existing regulations and business requirements.
Key Actors: Beta testers, customer experience & quality assurance (QA) teams, and regulatory bodies.
- Commercialization: Developing a strategy for launching the product or service into the market.
Responsibilities: Establishing a marketing, sales, pricing, and distribution strategy to ensure preparedness for production, internal support, and customer service processes.
Key Actors: Marketing, sales, customer service, and supply chain management teams.
- Deployment: Launching the product or service into the market, addressing the target audience, and beginning market penetration.
Responsibilities: Making the market aware of the innovation through advertising, public relations (PR), and promotions.
Key Actors: PR, marketing, customer service, and sales teams.
- Scaling & Improvement: Continually improving and scaling the innovation by reference to market feedback.
Responsibilities: Penetrate new markets and refine, improve, or expand products in response to feedback and alignment with performance metrics.
Key Actors: End Users, and operations, sales, marketing, R&D, and customer experience teams.
- Learning: Understanding the successes and failures of the innovation and crystallizing the lessons learned from them.
Responsibilities: Key performance indicators (KPI), return on investment (ROI), market feedback, and user satisfaction assessments.
Key Actors: End users, customer experience teams, leadership, marketing & sales, and data analytics teams.
Now that we’ve covered the high-level characteristics of the AI innovation-accountability relationship, the subsequent sections will explore the evolution of human’s role in creating value with generative AI (GenAI) over the next few years. In this ensuing exploration, we’ll touch upon three domains of interest—skills development, trustworthiness and governance, and social and economic impacts—viewing each through the lens of the AI-accountability relationship. Seeing as this is the last piece in our three-part series on creating value with GenAI, we’ll conclude by tying it all together with a set of actionable recommendations for navigating the near future of GenAI.
The Human Role in 2024 and Beyond
Skills Development (AI Literacy)
While governments, academia, non-profits, online learning platforms, and tech companies are recognizing the importance of AI skills development, particularly for GenAI tools and applications, the responsibility to develop and maintain AI literacy still falls chiefly on individuals. This isn’t to say that organizations should ignore AI skills development and procurement initiatives—in fact, they should allocate far more time and resources to these initiatives than they do currently—nor is it a critique of those organizations that are already engaged in these practices. Rather, it’s the more subtle idea that individuals are in a better position to learn about, adapt to, and capitalize on AI advancements more efficiently and effectively than the complex systems in which they exist.
What do we mean by this? A complex system, whether it takes the form of a government body or academic institution, has many moving interconnected parts, typically organized in an intricate hierarchical, distributed, and/or sequential fashion under one or several unifying functions or goals (with many additional sub-functions and goals also present). The complexity of such systems makes them vulnerable to a variety of potential failure modes, which tend to manifest as pain points, bottlenecks, silos, or simply gaps in understanding and communication within an organization, among a variety of other granular vulnerabilities like security, data, and IP risks. This suggests that as more “moving parts”—employees, teams, regimented workflows, supply chains, distribution channels, etc—are introduced into the system, measures to mitigate vulnerabilities must increase accordingly.
This is where redundancy comes into play. Failures—security breaches or data silos, for instance—within complex systems can be either isolated or widespread, but as an organization becomes more complex, anticipating which failures will occur in isolation, and consequently only affect a specific part of the system, is increasingly difficult. It’s not uncommon for a seemingly isolated failure to cause a failure cascade that destabilizes the entire system, even to the point of catastrophic failure of the whole—as a side note, this risk will grow as organizations divulge more control to AI systems (which are themselves complex systems) across critical functions like supply chain, hiring, and cybersecurity management. From a cost-benefit perspective, it therefore makes more sense to adopt a redundant failure mitigation strategy—implementing multiple measures to control for one possible failure—despite it being more costly and time-intensive in the short-term.
So, how does all this abstract talk about complex systems apply to the earlier claim that individuals are chiefly responsible for building and maintaining their AI literacy? In real-world organizations, redundancy usually equates to some form of bureaucracy, which is notoriously slow-moving. For instance, let’s take a medium-sized company with 300 employees that wants to implement an AI literacy campaign for its workforce before initiating organization-wide AI integration. In order to do so, the company will need to identify which teams and employees will be using which AI tools and for what purpose, understand the specific AI needs (which will be anything but constant) of different employees across the organization, develop actionable plans for addressing these needs holistically and identifying and resolving future gaps when they arise, acquire the resources necessary to implement, maintain, and adapt these plans, and develop another strategy for measuring the efficacy of the AI literacy campaign at multiple levels of the organization over time.
In addition to all of this, the company will further need to evaluate the compliance, safety, data, and cybersecurity risks associated with the AI literacy campaign, develop robust AI resource distribution channels that consistently allow teams and employees to meet their AI needs, restructure workflows and operations to account for AI-induced changes in work dynamics, and implement reporting and feedback channels that enable the workforce to voice AI-related concerns or risks. In truth, the company would need to do much more than this, but we’re assuming that readers get the point by now: organization-scale AI literacy campaigns, even at the scale of organizations with a few hundred members, are bound to move slowly.
When it comes to AI, an exponential technology, moving slowly isn’t an option if you want to stay at the forefront of innovation and maintain a competitive advantage—to be clear, we’re making this claim from a learning perspective, not a development one. Individuals are far better equipped to think and act flexibly and adaptively, responding to innovations in the AI landscape in accordance with their immediate needs, and capitalizing on the personal and professional benefits they may afford. You can wait for your organization to teach you about AI or you can take it upon yourself to do so, but the bottom line is this: if you do it yourself, you’ll be miles ahead of everyone else by the time that first AI literacy initiative comes around.
Obviously, there is a caveat here: an organization might have an anti-AI policy, preventing their workforce from leveraging AI in professional settings. In this case, the message remains largely the same: learn about AI on your own time, and when the time comes, implement it professionally. We would wager the anti-AI organizations, even if they’re well-intended, won’t last for much longer as competitive pressures to innovate and adapt to this powerful technology intensify.
Trustworthy AI and Governance
The question of how to build and maintain trust and confidence in AI, particularly GenAI, is one that should always remain on the center of society’s radar for a few vital reasons.
First, trustworthy AI is intrinsically valuable—it performs reliably, consistently, safely, and equitably across its intended tasks or functions. Second, trustworthy AI paves the way for further AI innovation—if people trust the AI tools and applications they’re working with, they’ll be more likely to embrace innovation rather than reject it (this will also apply to regulatory bodies). Third, trustworthy AI will have a clear application context—knowing exactly what an AI tool or application is designed to do (and how it will benefit you) will allow people and organizations to identify desirable human and AI skills more easily and may also help quell fears of automation-induced human replacement. Finally, trustworthy AI will be adopted quicker and more readily than its less trustworthy counterparts—this phenomenon will showcase where true value lies and evolves within the broader AI ecosystem.
Importantly, AI will not follow a trajectory where it self-calibrates (i.e., things work themselves out) in favor of trustworthiness—recall that in our previous post, we discussed the notion of AI race dynamics, which inspires a “race to the bottom” as some have put it. This process will need to occur with human intent, which begs the question of who is responsible for ensuring trustworthy AI innovation, and on what grounds?
At the highest level, this responsibility appears to fall on federal, state, and local governments, which are tasked with developing policies and regulations aimed at addressing key issues within the AI space like bias, discrimination, deepfakes, and unchecked surveillance. However, policymakers aren’t generally technical people, and attempting to regulate a technology as powerful as AI without technical understanding is a recipe for disaster. Policymakers must work together with AI researchers, academic institutions, scientific bodies, and non-profits to develop laws and regulations that reflect and preserve fundamental rights like privacy and autonomy within a framework that adequately mitigates the technical, social, and economic risks AI presents.
Nonetheless, finding this balance is extremely difficult—as of January, over 400 AI bills have been introduced in the US, counterintuitively suggesting a deeply fragmented and misaligned AI regulatory landscape. The US has yet to enact any federal AI legislation and the recent controversy and failure of California’s Safe and Secure Frontier AI Act, which was quite reasonable even in its nascent forms, was informed, in good faith, by leading AI researchers, and could’ve been a solid blueprint for early federal regulation, further demonstrates our point.
Even though government bodies are primarily responsible for ensuring trustworthy AI innovation, the simple fact is that they’re largely failing to do so currently, and in many instances, not out of their own volition. External pressures, especially those imposed by lobbyists and technological accelerationists, remain highly potent and influential, transparency and explainability limitations continue to raise safety and ethics concerns within the AI space, while in the meantime, leading AI companies steadily roll out more advanced versions of their products and services, expanding their user base and reaping enormous profits. Many other pressures hinder and complexify the process of constructing robust and resilient AI regulation, and in the near term, we may be better off if we shift the trustworthy AI accountability burden to another set of stakeholders, which may be more capable of addressing and dealing with it—regulation is still crucial and necessary, but the US needs to take a step back and figure out its national AI regulation strategy first. Looking at the EU and its AI Act might not be a bad place to start, though the AI Act certainly has many of its own problems.
Regardless, if governments aren’t doing enough to ensure trustworthy AI, who can bridge this gap? Those who have the technical and social resources and expertise to do so: tech companies. If the alarm bells just went off in your head, you’re right—many tech companies, particularly Big Tech, have a well-documented history of irresponsible, unsafe, and anti-democratic technology deployments, both on the national and international scale. Self-governance within the technology industry has been tried and tested, and the results have been anything but encouraging, if not utterly demoralizing. So, in the age of AI, where governments are still playing catch-up, how can we force tech companies to pick up the slack while governments iron down the right regulatory strategy?
Once again, we come back to individuals, and more specifically, AI users and the concept of AI literacy. As users become more AI literate, their corresponding knowledge of the various risks, benefits, and impacts AI creates will deepen. This will happen as AI tools and applications continue to become more accessible at progressively larger scales, failing and succeeding dramatically throughout a plethora of emerging use contexts.
If it’s true that most AI companies only exist because the services they provide are valuable to their end users, then it would seem that users possess power and influence in determining the trustworthiness standards of AI development. AI literacy is the most individually and collectively empowering mechanism through which to democratize the overarching institution of trustworthy AI. The refusal to use an AI product, provide in-depth feedback, or publicly criticize it due to safety, ethics, or trustworthiness concerns, especially when exercised at scale, is one of the most potent incentives through which to force companies into developing and deploying this technology responsibly. We’re not suggesting this should replace regulation in the long-term, but it should absolutely inform it and fill in its gaps in the short-term.
Before moving on, we must address a possible counterargument: why would AI literacy be any more empowering than other forms of digital technology literacy? For example, billions of people use social media, and yet, only a fraction of social media users are aware of the countless infringements on fundamental rights committed by the very companies whose platforms they leverage. To this, we answer that conventional social media users don’t fit the concept of an “active user” and are therefore far less likely to be curious about how the technology works and the impacts it generates. While not all AI users will be active users, we think that the majority will fall into this category, especially as AI tools and applications, most notably purpose-built GenAI models like AI agents and Custom GPTs, are explicitly designed to enhance and augment users’ abilities throughout various personal and professional task domains. Humans must work to create value with AI.
It’s also worth noting that sci-fi lore has cemented the idea of “bad AI” within humanity’s collective consciousness, and while this idea can be seriously damaging, in the context we’ve just described, it could be beneficial. A healthy amount of fear might be the push that less AI literate users need to begin recognizing their role in ensuring a trustworthy AI future.
Social & Economic Impacts
Anticipating the social and economic impacts AI will inspire, whether positive or negative, short or long-term, localized, systemic, or existential, is paramount to cultivating a safe and beneficial AI future. While we won’t dive into any specific impacts here due to our extensive coverage of them in other pieces on our blog, we will try to understand who will be responsible for predicting and managing them in the near future, and in doing so, demonstrate the fundamentally multifaceted and multidisciplinary nature of AI.
The process of anticipating a potential AI impact scenario can be broadly subdivided into several concrete steps:
-
Identify the key variables or sources linked to potential risk and benefit outcomes, and understand how changes within these variables or sources could impact risk and benefit trajectories.
-
Categorize and identify the possible risks and benefits the tool or application could inspire in one or many real-world settings that correspond with its intended use context.
-
Assess the timescale of potential risks and benefits to figure out the rate at which they might materialize.
-
Understand how many people and/or systems (e.g., energy grids, supply chains, government bodies, etc.) will be affected by said risks or benefits.
-
Understand whether these effects will be evenly distributed within the target population or disproportionately throughout certain sub-groups.
-
Assess the severity of potential risks and benefits to determine a prioritization structure.
-
Stress-test AI systems in controlled real-world environments during pre-deployment stages to probe for vulnerabilities that may create risks or undermine benefits. This includes manipulating risk and benefit sources and variables to understand the dynamics of potential impact scenarios.
-
Construct an actionable strategy through which to enact intended benefits or prevent unintended risks and envision where it might fail.
-
Independently test the strategy at regular intervals, especially during the early stages of product or service deployment, and then refine it accordingly.
-
If novel risks or benefits are uncovered or change significantly, or the AI tool or application undergoes modifications or updates that alter its risk-benefit profile, repeat this process from end to end.
The process above is a highly boiled-down version of the steps involved in AI risk management or impact forecasting and mediation. Yet, even at the level of a general overview, it’s clear how difficult and convoluted it might become—in theory, a single individual or a small team could be tasked with carrying out this process, and while this may improve its efficiency, flexibility, and agility, following this approach as AI advances and proliferates could prove largely ineffective (except where AI is designed to perform narrow procedural tasks within strict parameters). This is because social and economic AI impacts are influenced by more than just a specific tool or application’s inherent risk profile—factors like adoption rate and scale, end-user experimentation, malicious actors, unintended emergent use cases and AI capabilities, industry regulation, AI knowledge and skills, culture and population dynamics, and business and financial incentives must all be considered, among many other factors as well.
Consequently, anticipating AI impacts, particularly for general-purpose AI (GPAI) models like ChatGPT, Gemini, and Claude, necessitates a holistic approach that envisions social and economic risks and benefits from multiple informed real-world perspectives. Below, we’ve described an array of key stakeholders we believe should be invited into the AI risk-benefit discourse—in fact, we would argue these very stakeholders are essential to the future of AI forecasting. We’ve intentionally omitted AI developers and companies, safety and ethics researchers, risk analysts, and policymakers because of their obviously critical and sustained roles in understanding and predicting AI impacts as they emerge.
-
Behavioral Economists: How human psychology influences economic decision-making is vital to comprehending and analyzing the ways in which AI will modify and redefine individual and collective economic behaviors, purchasing decisions, and market dynamics. Behavioral economists will play a prevalent role in understanding the dynamics of automation in labor markets and the evolution of trust and confidence in AI products.
-
Social and Cognitive Psychologists: The malleability, diversity, and intricacy of human psychology must inform the investigation of AI’s impacts on human cognition and development, decision-making, critical reasoning, and judgment, mental health, and social phenomena and cohesion. Psychologists are integral to the exploration of emerging technologies like AI companions or tutors, and AI-powered social media and virtual reality experiences—we also desperately need an area of study that devotes itself solely to understanding the effects of sustained human-AI interaction on psychological development.
-
Anthropologists: Human culture is a powerful catalyst for innovation, change, and transformation. By providing deep and nuanced insights into the motivating factors driving different communities to accept or resist AI, anthropologists can inform predictions about how AI will alter complex social structures and hierarchies, the notions of individual and collective identity, and the ever-shifting status of cultural norms, both locally and globally. Anthropologists will also be responsible for ensuring that the AI risk-benefit discourse is inclusive, diverse, and representative of all relevant societal interests and values.
-
Philosophers: Driven by the fundamental philosophical tenet of knowledge seeking, philosophers will be tasked with tackling the “big questions” AI presents for the future of humanity. Understanding how to preserve meaning, purpose, and autonomy, maintain robust moral and ethical standards, encapsulate the existential threats AI poses, anticipate how the structure and ingestion of knowledge will change, and adapt to the new phenomenological and metaphysical experiences and environments AI will produce—all these tasks fall squarely within the philosopher’s repertoire.
-
Historians: The future of innovation is informed by the past, irrespective of how novel an innovation may seem—our creations are built on the shoulders of those who preceded us. Historians can help us make sure that we don’t replicate the mistakes of the past or fail to overlook solutions that have worked previously. By drawing parallels between the past and the present, historians will identify patterns, trends, and dynamics that, when superimposed upon current AI innovations, are indicative of technological trajectories that humanity has already encountered and managed, either successfully or unsuccessfully.
-
Linguists: Language is the primary mechanism through which humans communicate with each other, and it will remain the primary mechanism through which humans communicate with AI (at least in the near future). Linguists, particularly those with computational skills, will be instrumental in understanding not only how natural language-based AI models may evolve but also how the structure and semantics of human language and communication could change as a result of prolonged human-AI interaction.
-
Archaeologists: The study of physical artifacts over time provides a civilization-centric perspective on the evolution of human technology. Archaeologists will play a surprisingly formidable role in forecasting the impacts of AI-powered artifacts like smart cities and edge devices within a framework that fosters an understanding of social, architectural, and industrial adaptations to AI over long time periods.
-
Technology Influencers: If leveraged correctly, technology influencers could be enormously powerful agents for spreading AI awareness, shaping public opinion, and driving beneficial AI adoption. Via their large followings, influencers can also obtain granular observational insights into the nebulous social and business processes surrounding critical notions like responsible AI (RAI), the dissemination of AI knowledge, and socio-cultural acceptance or resistance trends.
-
Creatives: While creativity isn’t uniquely human, humans’ creative capacity most likely is. If we are to protect, preserve, and augment this capacity with AI, creatives of all kinds must be involved in developing a comprehensive forward-looking strategy through which to anticipate how AI could metamorphose creative concepts and practices like authenticity and originality, complex problem-solving, artistic expression and freedom, and creative enhancement.
-
CEOs: The more tangible real-world case studies we have to draw from, the more accurate our predictions on AI’s social and economic impacts will be. Being responsible for the big picture, CEOs can tap into a wealth of business-specific knowledge on how AI is affecting workplace dynamics and job roles, economic competition, business models and growth trends, operational transformations, and corporate social responsibility and ethics. If aggregated, insights from numerous CEOs could be the key to unlocking a comprehensive and adaptive model of AI in business.
-
Regular Citizens: At some point, every member of a sufficiently industrialized nation will be affected by AI, either directly or indirectly. Regular citizens aren’t only entitled to participate in the AI risk-benefit discourse but are arguably the most important kind of stakeholder. To predict how the future of AI may unfold, we need to understand how it’s being leveraged by the average person, for what purpose, and to what degree—theorizing behind closed doors in elite institutions can only go so far, and in fact, will likely lead to conclusions that are embarrassingly misaligned with dynamics of the real-world.
In an ideal world, most if not all members of society would be involved in AI impact forecasting seeing as we’re all stakeholders in our future. For now, however, the actors we’ve mentioned, if actively included in the AI risk-benefit discourse, will lay the groundwork that’s required to anticipate the future of AI comprehensively, and in a way that represents society’s best interests as a whole.
Recommendations
We’ve covered a ton of material throughout this series, so before concluding with some actionable recommendations for navigating the near future of GenAI, let’s briefly recap.
In part 1, we began by exploring the mystery behind GenAI’s value proposition, followed by a variety of AI success and failure stories over the last few years, grounding our discussion in a neutral perspective on GenAI innovation. In part 2, we ventured into lesser-known experimental territory, examining the multiplicity of factors driving GenAI innovation and offering a series of bold predictions for near-term GenAI technology advancements. In this final piece, we broadened our perspective and considered the evolution of humans’ role in building a safe and responsible AI future, rooting our discussion in the relationship between accountability and innovation.
Now that we have a complete context, we leave readers with the following recommendations:
-
Don’t wait to learn about AI. Start now, and tell your friends, family, and coworkers to do the same.
-
Ask yourself which of your skills will still be relevant a decade from now. Identifying skills gaps and strengths is essential to enacting value with AI.
-
Explore multiple perspectives on the evolution of AI. Tech experts are by no means the only people worth listening to and the US also isn’t the only country developing advanced AI.
-
Challenge the status quo. Remember that you have a voice in the future of AI, and if something doesn’t sit right with you, share it.
-
Learn from others and their experiences. Keeping up with AI innovation will require an open and collaborative mindset.
-
Be critical of certainty. Plenty of self-proclaimed “AI gurus” will emerge, and while they may be right sometimes, don’t accept their predictions at face value.
-
Push yourself to think non-linearly. If you believe a given AI innovation will take 5 years to materialize, ask yourself what would happen if it emerged in one year.
-
Use different GenAI tools regularly. The GenAI tools landscape is steadily expanding, and different tools will require different skill sets to operate well.
-
Follow the AI safety and regulation discourse. Understanding AI’s risks and benefits will inform how you use AI and for what purpose.
-
Take note of AI failures and successes. Avoiding the AI hype or doomer train can be tricky, but this will help subsidize your AI understanding pragmatically.
-
Consider how AI is impacting your psychology. Being honest with yourself about how AI affects your behavior and thought process will help keep you grounded.
For readers interested in learning about other related topics within the AI space, like governance and risk management, we suggest following Lumenova AI’s blog.
On the other hand, for those who crave tangible solutions to their AI risk management and governance needs, we invite you to check out Lumenova’s RAI platform and book a product demo today.