November 5, 2024
Mastering Generative AI Models: Tackling Core Challenges
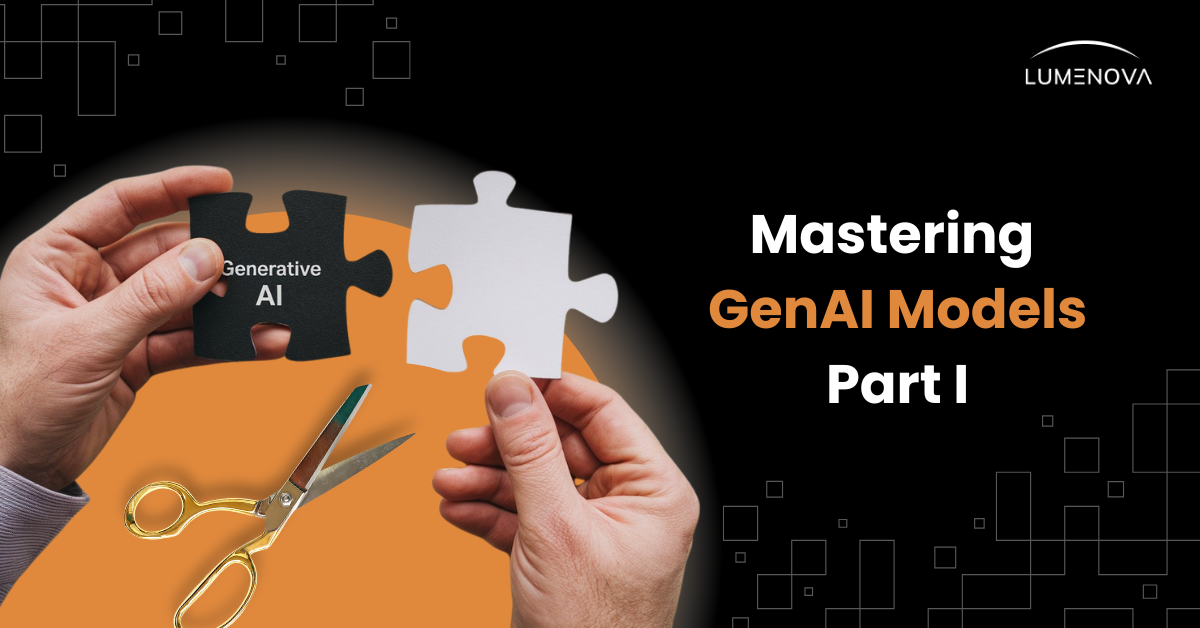
Contents
Generative AI (GenAI) will reshape industries, fueling hyper-personalized content, transforming customer engagement, and driving operational efficiency. But for many organizations, the path to full AI integration is lined with challenges, from data privacy and ethics to scaling and cost management.
To support your journey in leveraging GenAI’s full potential, we’re introducing a comprehensive three-part series. Each installment will dive into the critical challenges of adopting generative AI, offering insights and practical strategies to navigate key obstacles and maximize success. In these three parts, we’ll explore the main barriers to GenAI success, as illustrated below:
Data Challenges: Managing Quality, Privacy, and Security
Data is the lifeblood of GenAI, and for organizations to enact the technology’s potential, they must balance a need for rich, diverse data with stringent privacy and security protections. In insurance, for instance, AI models ingest a vast array of client information—from claims history to demographic insights—to deliver accurate risk assessments and policy recommendations. However, if this kind of data isn’t adequately protected and managed, significant privacy and security risks will almost certainly emerge. Mishandling or overexposing personal data can jeopardize customer trust and lead to regulatory repercussions and hefty fines.
How much does GenAI’s reliability and accuracy depend on its training data?
GenAI’s reliability and accuracy are deeply tied to the quality and diversity of its training data. Without a rich and diverse dataset, AI models can produce biased or inaccurate predictions with serious real-world impacts.
This is why diverse data matters:
- Reduces bias: Helps prevent skewed, unfair results.
- Increases accuracy: Boosts predictive power across scenarios.
- Promotes fairness: Supports balanced, inclusive decisions.
- Improves reliability: Builds confidence in sensitive fields like healthcare.
- Meets compliance: Aligns with legal standards on fairness and transparency.
- Builds trust: Ensures AI serves user needs reliably.
Now, let’s look at how this unfolds in HR, healthcare, and finance where data diversity makes a meaningful difference.
Examples of Industries Where Data Diversity Matters
HR & Recruitment
In HR and recruitment, companies are increasingly leveraging AI, and now GenAI, to enhance candidate screening and talent identification. However, if the training data is not representative, the technology can reinforce existing biases.
A recent report, by UNESCO underscores the prevalence of gender bias in generative AI. The study found that many AI systems reflect substantial biases based on gender and sexuality. For instance, some AI models associate feminine names with traditional gender roles and generate less positive responses when presented with LGBTQ+ subjects. This reflects a data issue where AI systems are often trained on biased datasets that do not represent the diversity of the real world.
UNESCO identified three primary sources of bias: data limitations, algorithmic bias, and deployment issues. For example, biased data in hiring could lead AI models to favor male applicants if historical hiring practices skewed in that direction. In some cases, AI models have penalized candidates whose resumes included terms like “women’s soccer team” or “graduate of a women’s college,” demonstrating how gender-based disparities can become embedded within AI systems.
Healthcare
Hospitals and research centers are exploring AI for medical imaging disease diagnosis. However, recent research highlights serious concerns regarding the accuracy of AI systems developed for diagnosing skin cancer, particularly among individuals with darker skin tones. While the following example stems from traditional AI, it highlights a crucial lesson for GenAI applications in healthcare.
A comprehensive review published in PLOS Digital Health identified six key challenges in generative AI for medicine, including bias, privacy concerns, and hallucination (where AI generates incorrect or nonsensical outputs). The study highlights that generative AI models trained on non-representative datasets are at high risk of producing biased results. For instance, limited representation of individuals with darker skin tones in training data can lead to less accurate diagnoses for these groups, ultimately affecting the generalizability of AI tools across diverse populations.
Finance
GenAI is making notable strides in the finance sector, particularly in credit risk assessment, where it aids in analyzing data, expediting loan approvals, and customizing financial products. Specifically, GenAI has the potential to refine data analysis, streamline approval processes, and tailor financial products, but its effectiveness critically depends on access to robust, inclusive datasets.
According to McKinsey’s report, the success of these machine learning (ML) models hinges on diverse, high-quality datasets. Without them, GenAI risks generating biased or incomplete insights, which could disproportionately impact clients from lower-income or rural areas.
In McKinsey’s survey of senior credit risk executives, 79% highlighted data quality as a critical concern. Many AI models are currently trained on datasets dominated by urban or high-income profiles, which introduces bias. These skewed assessments may result in fewer loan approvals or restricted access to tailored financial solutions, limiting opportunities for these demographics.
McKinsey’s findings emphasize that unlocking the full potential of GenAI in credit risk requires financial institutions to invest in high-quality, representative data. By focusing on data diversity and inclusivity, they can create fairer, more accurate AI-driven credit assessments that better serve the financial needs of all clients, regardless of background or income level.
Strategies to Ensure Data Privacy and Security in Generative AI
Is your organization prepared to safeguard data privacy and security as it dives deeper into GenAI? With AI processing so much information, data privacy and security have become top priorities.
To effectively safeguard data, consider these strategies:
Data Minimization
Collect only the data that is necessary for specific purposes. This reduces the risk of data breaches (unauthorized access to sensitive information) and helps you comply with laws like CCPA (California Consumer Privacy Act, which protects personal data in the United States) and GDPR (General Data Protection Regulation, which protects personal data in the European Union). By limiting what you collect, you can manage and protect sensitive information more effectively.
Robust Access Controls
Use strong access controls to protect sensitive data. For example, Role-Based Access Control (RBAC) means giving people access only to the data they need for their jobs. Similarly, Multi-Factor Authentication (MFA) requires users to verify their identity in more than one way (like a password plus a text message code), adding extra security and reducing the chance of unauthorized access.
Differential Privacy
This technique allows organizations to analyze data without revealing individual identities. By adding “noise” (random information) to datasets or combining data points (aggregating), you can protect personal information while still gaining valuable insights. This is especially important in fields like healthcare, where keeping patient information confidential is crucial.
Effective Data Encryption
Encryption secures data by converting it into a coded format that only those with the correct decryption key can read, protecting it from unauthorized access. End-to-end encryption ensures data remains protected from collection through processing, while AES-256 is a highly trusted standard for securing data at rest. To manage access, Public Key Infrastructure (PKI) securely controls encryption keys.
For privacy-sensitive cases, homomorphic encryption enables computations on encrypted data without requiring decryption. Finally, TLS and SSL protocols protect data during transit, safeguarding it from interception. Together, these methods strengthen security and support compliance as GenAI systems expand.
K-Anonymization
This method modifies datasets so that individuals cannot be easily identified. It ensures that each person in the dataset is indistinguishable from at least 𝑘 other individuals (making it harder to pinpoint any single person). This helps maintain privacy and reduces the risk of re-identification (the process of matching anonymous data to individuals) while still allowing effective analysis.
Federated Learning
Federated learning is a ML approach that enables organizations to collaboratively train a shared model without directly sharing sensitive data. Each institution involved trains the model locally on its own data and only shares the model updates (such as gradients) with a central server. This method significantly enhances privacy and data security, as the raw data never leaves its original location, making it less vulnerable to breaches or misuse. By leveraging federated learning, organizations can benefit from collective insights while adhering to data protection regulations.
Penetration Testing
Penetration testing is a proactive security measure that involves simulating cyberattacks on systems to identify vulnerabilities before they can be exploited by malicious actors. Regular penetration tests help ensure that AI systems are resilient against various attack vectors, thereby safeguarding sensitive data and maintaining the integrity of the overall system. This practice not only helps in identifying weaknesses but also assists organizations in strengthening their defenses and compliance with security standards.
Data Logging
Data logging refers to the systematic tracking of access and usage within an AI system, creating an audit trail that enhances accountability and allows for the detection of unusual activities. Effective logging is crucial for meeting compliance requirements and identifying potential security issues during active system use. Audit logs provide visibility into user actions, system changes, and can serve as critical evidence in case of security incidents or audits.
Computational Resources: Navigating the Demands of AI Deployment
As organizations strive to implement GenAI, the need for robust computational resources will increase. Training and deploying sophisticated AI models requires significant computational power, which can pose challenges in terms of cost, infrastructure, and efficiency. The stakes are high—insufficient resources can lead to delayed projects, suboptimal model performance, and ultimately, missed opportunities for innovation.
Understanding the Resource Demands
GenAI models require substantial processing power. High-performance GPUs (Graphics Processing Units) and TPUs (Tensor Processing Units) are essential to handle the vast data volumes and intensive calculations involved in training these models. However, as organizations grow, scaling GenAI efforts becomes challenging—not only due to rising operational costs but also due to the need for more infrastructure, efficient storage solutions for large datasets, and increased energy consumption. These demands can make expanding GenAI capabilities both complex and costly.
Strategies for Efficient Resource Management
Model Optimization
The first step in managing computational resources is optimization. AI model optimization involves refining models to improve their efficiency and reduce their resource consumption while maintaining accuracy.
-
Pruning: Removing parameters that contribute little to the model’s performance. For example, Google’s MobileNet architecture employs pruning techniques to streamline convolutional neural networks (CNNs). By cutting away less significant weights, the model retains its accuracy while consuming less computational power. This makes MobileNet particularly suitable for deployment on mobile devices, where resources are limited.
-
Quantization: The conversion of high-precision floating-point numbers (like 32-bit) into lower-precision formats (like 16-bit or 8-bit). This approach significantly reduces the model’s size and accelerates inference times. Companies like NVIDIA utilize quantization to facilitate quicker processing in real-time applications, such as those found in autonomous vehicles, without sacrificing performance.
-
Knowledge Distillation: This process involves training a smaller, more efficient model (the “student”) to replicate the behavior of a larger, more complex model (the “teacher”). The student model achieves similar performance with fewer resources, making it well-suited for deployment in resource-constrained environments.
-
Mixed Precision Training: By using a mix of lower and higher-precision computations during training, mixed precision training reduces memory usage and speeds up processing without sacrificing model accuracy. This technique is particularly effective for large models that require intensive computational resources.
Cloud-Based Solutions
This is where cloud computing comes into play. Leveraging cloud-based solutions enables organizations to access scalable and flexible computational resources that can be adjusted based on demand, reducing the need for significant upfront hardware investments.
-
Reliability and Compliance: Cloud providers typically maintain high standards of reliability and compliance, handling data storage and processing in ways that meet industry regulations. This allows organizations to focus on their core objectives, knowing that their infrastructure is equipped with up-to-date protections and robust disaster recovery options that enhance both performance and resilience.
-
Scalability: AWS (Amazon Web Services) Elastic Compute Cloud (EC2) allows organizations to scale resources in real-time. During peak shopping events, like Black Friday, retailers can quickly increase their computational resources to handle the surge in online traffic, ensuring smooth transactions without the burden of permanent infrastructure costs.
-
Flexibility: platforms like Microsoft Azure offer a suite of machine learning services that empower organizations to experiment with various AI models. This flexibility supports rapid iterations and testing of new algorithms, enabling companies to adapt swiftly to market changes and improve their AI offerings.
With these AI-driven solutions in place, organizations can further enhance their AI applications by implementing caching strategies.
Model Caching
Caching is a technique that stores the results of previous computations or frequently accessed data, reducing processing time and enhancing application performance.
-
Speed Enhancement: Facebook utilizes caching strategies to store results of commonly accessed ML predictions in their content delivery network (CDN). This enables them to serve personalized content quickly, minimizing latency and enhancing user experience.
-
Reduced Latency: In the financial sector, firms like Goldman Sachs implement caching to store the outputs of complex risk calculations. By keeping these results readily available, they can provide traders with real-time data without recalculation delays, improving decision-making speed during critical market movements.
Ethical and Societal Implications
As you consider adopting GenAI technologies in your organization, it’s crucial to reflect on the ethical and societal implications this powerful tool inspires. While GenAI can drive innovation and efficiency, it also carries significant risks that can impact your business and society at large. Here’s what you need to know to navigate these challenges responsibly.
Addressing Potential Misuse
One of the foremost concerns with generative AI is the potential for misuse. Understanding these risks is vital for safeguarding your organization’s reputation and maintaining customer trust.
-
Deepfakes: AI-generated hyper-realistic videos where individuals appear to say or do things they never did. Imagine your brand being associated with misleading content that misrepresents your values or message. Deepfakes can undermine public trust and even disrupt markets. It’s essential to implement measures to detect and combat Deepfakes, protecting both your brand and your customers.
-
Misinformation: AI-generated convincing false narratives, which can complicate and obscure your communications strategy. In a digital world already saturated with misinformation, the last thing your business needs is to contribute to the noise. As you adopt these technologies, consider how to establish clear communication channels and verify the authenticity of content before it reaches your audience.
Tackling Bias and Ensuring Fairness
Bias in AI systems can lead to unfair and discriminatory outcomes, which can adversely affect your customer relationships and your brand’s integrity.
-
Bias in Training Data: If your AI models are trained on biased datasets, the results may reflect, perpetuate, and even amplify those biases. For instance, if your organization uses AI for customer service interactions, biased training data could lead to unfavorable treatment of certain customer demographics, potentially causing reputational harm and eroding trust within your community. It’s crucial to ensure that your datasets are diverse and representative to avoid these issues.
-
Algorithmic and Model Architecture Bias: Bias doesn’t only come from data. The design of algorithms and model architectures can also introduce bias. Certain algorithms or structures may inherently favor specific patterns or groups, leading to skewed outcomes even when trained on balanced data. Regularly evaluating model design helps identify and mitigate such biases, promoting fairer results.
-
Ensuring Fairness: Actively seeking out diverse training datasets and implementing regular audits of your AI systems are essential steps in AI bias mitigation, helping to reduce unfair outcomes and promote more equitable results. Make it a priority to assess how your AI models perform across different demographics. This commitment to fairness not only strengthens your brand reputation but also fosters loyalty and trust among your customers.
The Need for Ethical Guidelines and Regulatory Frameworks
Navigating the ethical landscape of GenAI requires a structured approach, including establishing clear guidelines and adhering to regulatory frameworks.
-
Establishing Ethical Guidelines: Consider developing comprehensive ethical guidelines tailored to your organization. These guidelines should outline acceptable use cases for GenAI and define responsibilities regarding transparency and accountability. By fostering a culture of ethical decision-making within your teams, you ensure that AI development aligns with your organization’s core values.
-
Regulatory Frameworks: Staying informed about evolving regulations is essential for implementing GenAI responsibly. Compliance with regulatory standards not only helps organizations avoid legal issues but also builds trust with users by demonstrating a commitment to ethical practices. As AI technologies advance, regulations will continue to adapt, addressing new challenges in privacy, fairness, and accountability. By keeping up with these changes, organizations can ensure that their AI applications remain aligned with societal expectations and industry standards.
At Lumenova AI, we specialize in helping businesses navigate the complexities of AI regulatory compliance, providing explainability, validation, personalization, data privacy, and risk assessment solutions, ensuring you stay ahead of the curve.
Conclusion
This brings us to the end of the first part of our series. We’ve highlighted how transparency and interpretability go beyond compliance—they’re foundational for building trust and ensuring AI serves its intended purpose responsibly. By focusing on ethical guidelines, regulatory frameworks, and clear communication, organizations can transform GenAI from a “black box” into a trusted ally.
In the upcoming second part of our series, we’ll dive further into model Interpretability and transparency. We’ll cover critical steps for managing AI hallucinations, developing user-friendly documentation, and enhancing AI’s reliability.
Join us as we explore more practical ways to make GenAI a transparent, ethical, and trusted asset within your organization.