November 26, 2024
Making Generative AI Models Work: Cost Management in GenAI
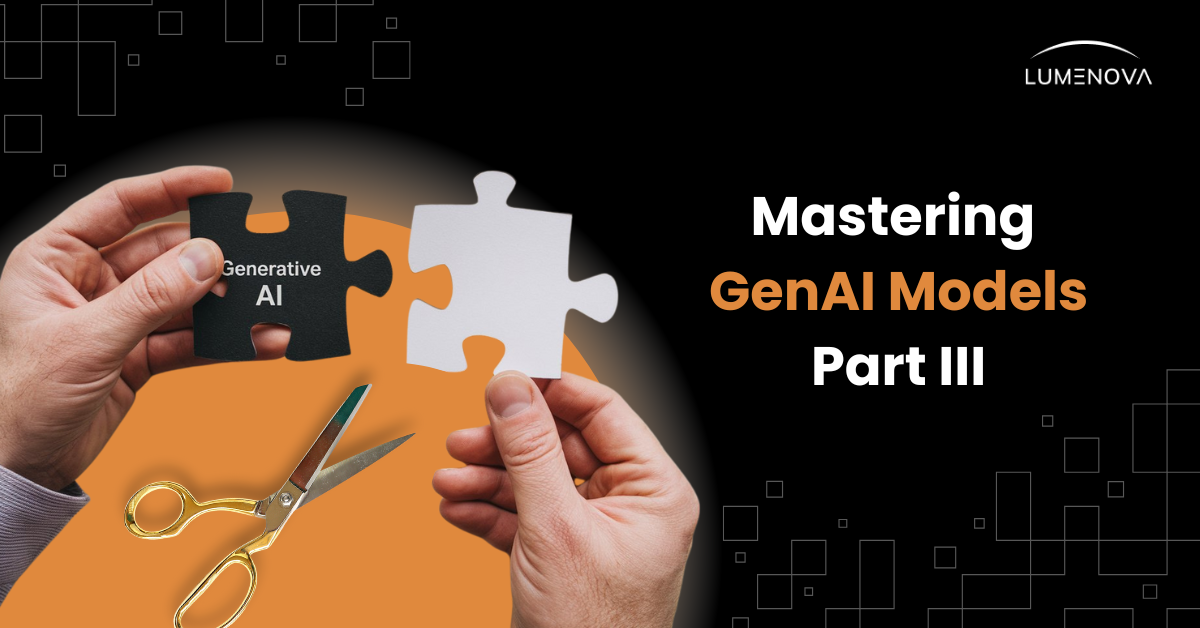
Contents
Integrating generative AI (GenAI) isn’t just a technical endeavor—it’s a financial commitment. In Parts I and II of this series, we examined the essential groundwork: tackling core challenges in data privacy and fairness, and building trust through transparency and interpretability. With these foundations established, there’s a final, critical piece to address: cost and resource management.
GenAI requires substantial investment in compute, data storage, and infrastructure, which can quickly strain budgets if left unchecked. Therefore, in Part III, we cover several strategies that help balance AI ambitions with sustainable financial practices. By aligning advanced AI solutions with disciplined budgeting, companies can maximize the impact of GenAI while staying financially responsible.
Integration and Scalability
To fully leverage GenAI, organizations must go beyond merely adopting new technology—they must ensure seamless integration with existing systems without compromising operational stability. Yet, integration is rarely straightforward; the road is often dotted with challenges that, if unaddressed, can limit AI’s transformative impact.
Key Challenges
Legacy System Compatibility
GenAI often struggles to function smoothly with legacy systems, where incompatibilities in data formats, outdated architectures, and limited API capabilities create hurdles. Aligning these older systems with modern technology requires a deliberate approach, bridging the gaps so AI can operate as a cohesive part of the existing infrastructure.
Data Silos
Data silos are a significant barrier to effective, secure AI. When information is scattered across departments, AI systems lack access to the complete data they need to perform accurately and reliably. Consolidating these data sources it’s essential.
- Security Risks: Isolated data is harder to monitor and secure, often creating security blind spots. Transparent data management enables consistent and robust security protocols, reducing these vulnerabilities.
- Dark Data Challenges: Data left unutilized in silos—often referred to as “dark data”—can obscure valuable insights and lead to gaps in AI accuracy. Addressing this hidden data strengthens the AI’s capacity to deliver actionable, reliable outcomes.
Technical Expertise
Without in-house expertise in AI integration, organizations can face costly delays and implementation struggles. Teams skilled in integrating AI into complex environments are necessary to streamline the process, making technical expertise an indispensable asset in achieving a smooth transition.
Change Management
GenAI can disrupt established workflows, creating understandable resistance. A clear, structured approach to change management helps teams adapt, fostering buy-in and ensuring that new, AI-driven processes enhance rather than hinder daily operations.
Example: A large logistics company introduced a GenAI tool to optimize delivery routes, replacing a manual process managed by dispatchers. Initially, the dispatch team resisted the change, fearing job displacement. The company addressed this by conducting workshops that demonstrated how the tool would reduce repetitive tasks and allow dispatchers to focus on strategic planning. By involving the team early and showing how AI complemented their roles, the company secured buy-in and successfully transitioned to the new process with minimal disruption.
Strategies for Successful Integration and Scalability
Comprehensive IT Assessment
Start by thoroughly assessing your organization’s current IT setup to pinpoint integration points and possible bottlenecks. This proactive approach allows for a customized strategy that ensures AI enhances rather than undermines existing infrastructure.
Example: For instance, a financial services company preparing to integrate an AI-based fraud detection tool began by evaluating their existing IT systems. The assessment revealed that their legacy database lacked the processing speed required for real-time AI predictions. By upgrading to a cloud-based solution, they not only resolved the bottleneck but also created a scalable infrastructure for future AI applications.
Phased Rollout
Rather than deploying AI everywhere at once, gradually introduce it to the organization. Testing the integration in select areas allows for adjustments based on real-world feedback, paving the way for a smoother, full-scale deployment.
Example: A logistics company used APIs to integrate an AI-powered route optimization tool with its existing fleet management system. The modular setup allowed the company to trial the AI system with a subset of routes and adjust API configurations as needed before expanding to full-scale operations.
Data Preparation and Integration
Data unification across systems is key to scalable AI solutions. By establishing a consistent data pipeline, organizations equip their AI models with high-quality, accessible data, which strengthens model performance and insights across the board.
Example: A global insurance firm deployed AI to improve claims processing accuracy. Before implementation, they unified data from disparate sources, including legacy databases and third-party reporting tools, into a centralized data warehouse. This consistent data flow enabled the AI system to detect patterns and anomalies more effectively, reducing processing times by 30%.
Modular Integration Using APIs
APIs enable AI to interact seamlessly with older systems by acting as bridges that standardize communication between technologies. They reduce the need for costly system overhauls and allow organizations to adapt AI solutions incrementally, ensuring smooth interoperability and scalability.
Example: A fintech company adopted an AI-powered customer service chatbot that interfaced with their existing CRM system via APIs. This modular approach allowed the chatbot to pull customer data in real time without requiring a complete system overhaul, ensuring minimal disruption while scaling the AI’s capabilities across different support channels.
Training Programs
Structured training and clear communication around the benefits and functions of AI can reduce resistance. Ensuring that employees understand AI’s role and feel confident in new workflows is crucial for long-term success.
Example: A healthcare organization implemented an AI-powered scheduling system for doctors and nurses. To ensure a smooth transition, they organized training sessions that demonstrated how the system would reduce manual scheduling errors and save time. Employees were also given access to a help desk for ongoing questions. This approach built trust in the system and empowered staff to integrate it seamlessly into their daily routines.
Cost and Resource Management: Maximizing AI ROI
GenAI integration can quickly become costly, especially with the substantial requirements for data processing, storage, and computation. As businesses expand their reliance on cloud infrastructure, costs can easily accumulate if not carefully managed. A disciplined approach to cost and resource management is essential to achieving a positive ROI.
Example: Coca-Cola’s very recent holiday advertisement, “The Holiday Magic is Coming,” produced using the Real Magic AI platform, is poised to significantly enhance the company’s ROI despite the backlash it has received. By leveraging generative AI (GenAI) for this campaign, Coca-Cola aims to achieve greater efficiency in production and personalized engagement with consumers, ultimately driving higher returns.
Key Cost Challenges
High Development and Deployment Costs
AI requires significant upfront investments in data acquisition, infrastructure, and model training. Without clear financial oversight, these costs can rapidly exceed projections, straining the overall budget.
API Call Costs
API calls, particularly in scenarios like real-time financial transactions or high-traffic e-commerce platforms, can rapidly increase costs due to the volume of interactions between systems. Managing these API calls effectively is crucial for maintaining budget control.
Unpredictable Cloud Costs
Cloud computing offers scalability and flexibility, but these benefits come with fluctuating costs. For instance, a retail company may face unexpected charges during peak shopping seasons, such as Black Friday, when workloads spike, and underutilized resources during off-peak times, leading to inefficiencies and unpredictable expenses.
Strategies for Effective Cost Management
Conducting Cost-Benefit Analysis
Start by evaluating the financial impact of AI investment. This involves a careful analysis of projected costs, potential gains, and long-term ROI. Are you integrating AI where it truly adds value? Prioritizing high-impact use cases ensures that AI investments contribute to strategic business outcomes.
Exploring Cloud-Based AI Services
Leveraging cloud services provides scalability without committing to expensive hardware. Providers like AWS (Amazon Web Services), Microsoft Azure, and Google Cloud offer transparent and predictable pricing structures, along with tools like AWS Pricing Calculator, Azure Pricing Calculator, and Google Cloud’s Pricing Tool to help forecast expenses and ensure costs align with projected use.
Cloud Cost Optimization
Cloud costs can quickly devour a budget without oversight. By employing best practices like identifying and eliminating idle resources, right-sizing computing services, and exploring autoscaling with tools like Kubernetes, companies can better align cloud spending with actual needs.
Real-Time Monitoring and Analytics
Set up real-time monitoring for key metrics—CPU, memory, storage, and network traffic—to identify cost inefficiencies. Alerts for unusual activity help avoid waste, while continuous monitoring ensures resources are sized correctly for usage patterns.
Automating Scaling with Demand
Scaling on demand, particularly with autoscaling solutions, prevents unnecessary spending on idle resources. This strategy ensures that cloud resources adjust dynamically with usage, so you’re only paying for what you need, exactly when you need it.
Example: A media streaming service uses cloud-based AI to recommend content to users. During peak hours (evenings and weekends), autoscaling increases computing power to handle the surge in viewership. When traffic drops overnight, resources automatically scale down, reducing unnecessary costs. This setup ensures the company pays for exactly what it needs based on user demand, without over-allocating resources.
Use Cases: Choosing the Right Applications for Generative AI
GenAI has impressive potential, but realizing this potential depends on whether you understand where it can truly add value. For example, using GenAI to generate personalized marketing content, automate customer support with chatbots, or create dynamic product recommendations can ensure resources are used effectively and deliver meaningful outcomes.
Key Considerations
Assessing Use Case Relevance
Not every task is suited to GenAI. For example, using AI to handle routine data entry might be excessive and resource-intensive compared to traditional automation tools. In contrast, AI can be highly effective in generating personalized content for marketing campaigns, where unique customer interactions are valuable. Focusing on use cases that match AI’s strengths—like creative content generation, customer engagement, or data-driven insights—can maximize its impact.
Evaluating Business Needs and User Impact
AI applications should align with business goals and improve the user experience. For instance, in e-commerce, GenAI can boost customer engagement by generating personalized product recommendations or crafting targeted email campaigns. However, implementing AI for every customer interaction may not add sufficient value to justify the investment. Focusing on areas that enhance user satisfaction, such as automated support chatbots, can provide a clearer return on investment.
Strategies for Identifying High-Impact Use Cases
Thorough Use Case Evaluation
Begin by evaluating potential AI applications based on their alignment with business goals, the technical complexity involved (e.g., the level of integration or customization required), and their potential ROI. For example, a publishing company might assess whether AI-generated articles could enhance its content offerings. By analyzing factors like implementation costs, required technical resources, and the projected increase in reader engagement, the company can determine whether this is a viable AI application or if its efforts would be better directed elsewhere.
User Needs Analysis
Conduct a detailed analysis of user needs to identify where AI can address genuine pain points. For example, in online customer service, GenAI can reduce wait times by providing immediate, automated responses to FAQs. This approach targets a common user pain point, ensuring that AI serves a valuable role in improving user experience.
Prototyping and Iteration
Start small by developing prototypes and running pilot projects to test AI applications in real-world environments. A healthcare provider, for instance, could prototype a GenAI tool for creating patient summaries from medical records. By testing this in a controlled setting, they can gauge its accuracy, impact on workflow, and utility to healthcare professionals before scaling it organization-wide. Iterative testing enables refinement, helping teams ensure that AI meets its intended purpose effectively.
Driving Adoption: Gaining Buy-In and Building Confidence in AI
Introducing GenAI can create anxiety around job displacement and change resistance. Successfully driving adoption requires building trust in AI’s role, which involves not just communicating its benefits but also fostering a deeper understanding of its purpose, decision-making processes, and ethical guidelines. It’s important to ensure transparency in how AI systems work, provide clear insights into their decision-making, and demonstrate accountability for their outputs. To reinforce this trust, using a responsible AI platform is crucial. Such platforms ensure that AI technologies are deployed ethically and in line with organizational goals.
Additionally, offering continuous education and involving users early in the AI adoption process—through hands-on demonstrations, training, and open dialogue—ensures that employees feel confident, supported, and valued in adapting to new workflows. The use of responsible AI platforms further strengthens this by providing real-time insights into AI decision-making, facilitating a deeper understanding and stronger buy-in across the organization.
Key Challenges
User Resistance
Introducing AI can spark fears about job security, as employees worry that automation could replace their roles. This fear can quickly turn into resistance, especially if there’s limited communication about AI’s purpose and impact. When employees are unclear on how AI will be used, rumors and anxiety spread, stifling enthusiasm for the technology.
To address this, leaders should communicate openly about AI’s role as a tool to support. Involving employees in the adoption process—through Q&A sessions, pilot programs, and demonstrations—can help alleviate fears, build trust, and show firsthand how AI will enhance their work by handling repetitive tasks, allowing them to focus on higher-value activities.
Concerns About Job Redefinition
As AI reshapes roles, employees may feel uneasy about shifting responsibilities and the need for new skills. This “job redefinition” concern can be particularly daunting for those accustomed to established routines, as they now face adapting to AI-enhanced workflows or using unfamiliar tools.
Organizations can ease this transition through targeted upskilling programs and clear communication. For instance, in customer service, where AI chatbots handle basic queries, employees can be retrained to focus on complex problem-solving and personalized service. Offering workshops and ongoing training empowers staff to adapt, while emphasizing the unique value of human oversight reassures them that their roles remain essential. Regular updates on role evolution and career growth opportunities help employees feel prepared, valued, and aligned with the organization’s AI vision.
The Trust Deficit in Generative AI
For all its promise, GenAI faces a challenge that no amount of processing power can solve on its own: trust. Beyond fears about job displacement, there exists an entrenched skepticism regarding AI’s ability to provide true business value in a manner that is transparent, actionable, and aligned with both regulatory standards and ethical imperatives.
-
Substance Over Hype: While the capabilities of GenAI have been widely celebrated, decision-makers are increasingly asking a critical question: does it truly deliver tangible, measurable benefits, or is it another overhyped technological trend? In the absence of clear, demonstrable results, organizations are hesitant to allocate significant resources to what might appear to be a high-risk gamble rather than a strategic leap. To bridge this gap, selecting the right use-cases is crucial. AI Governance platforms provide the tools to help organizations evaluate the potential benefits and costs from the outset, allowing them to build a solid business case. These platforms enable the identification of high-value use cases that can deliver measurable business outcomes, transforming potential risks into high-reward opportunities.
-
The Black Box Dilemma: Trust in AI diminishes when users cannot understand the mechanisms behind its decision-making. GenAI models are often viewed as opaque “black boxes,” leading stakeholders to question the reliability and fairness of their outputs. For organizations in industries where precision and accountability are paramount, this lack of transparency can significantly hinder AI adoption. Responsible AI Governance frameworks address this by ensuring AI systems are not only explainable but also auditable. These frameworks provide detailed insights into how decisions are made, offering stakeholders clarity on the underlying processes and building confidence in the system’s outputs.
-
Ethical and Security Roadblocks: Algorithmic biases, data misuse, and non-compliance with legal frameworks remain substantial concerns, particularly in regulated industries such as healthcare, finance, and government. These issues create friction and heighten resistance to AI adoption, especially in high-stakes sectors where regulatory scrutiny is intense. Responsible AI platforms help mitigate these risks by embedding ethical principles and security protocols into the development and deployment of AI systems. These platforms ensure AI models meet compliance standards and ethical guidelines, reassuring stakeholders that AI systems are not only effective but also aligned with societal values and regulations.
Strategies for Driving Adoption
Training and Education
Equip teams with the skills they need to work alongside AI. Training sessions that cover how GenAI supports their tasks can ease concerns, build confidence, and highlight how AI can actually enhance their roles by taking over repetitive tasks or streamlining problem-solving, allowing them to focus on higher-level work.
Equip your team to thrive alongside AI. Explore Part 1 to understand how AI literacy mitigates risks and fosters responsible adoption, and Part 2 to uncover strategies for integrating AI into workflows while empowering innovation and governance.
Clear Communication
Open and transparent communication about AI’s role and the organization’s goals for its use can dispel misconceptions. Make sure employees understand that AI is intended to enhance rather than replace their work. For example, regularly sharing updates on pilot projects and how AI is benefiting different areas of the business can demystify its purpose.
Pilot Projects
Start with small, controlled pilot projects to demonstrate AI’s capabilities and impact. For instance, implementing AI in one department (like customer service) as a trial phase allows employees to see firsthand how it works and can alleviate fears by showcasing positive outcomes and gradual, manageable changes.
Change Management
A structured yet flexible and adaptable change management approach can help address resistance and guide teams through the adoption and integration process. Engage stakeholders early, provide feedback channels, and recognize those who embrace the transition, reinforcing a positive attitude toward AI.
Responsible AI Platforms
Using a responsible AI platform could empower organizations to deploy AI technologies with a commitment to ethics, accountability, and transparency. Lumenova AI platform integrates fairness and accountability at every stage of the AI lifecycle—from model design and training to deployment and scaling.
By leveraging advanced automated tools to identify and mitigate biases in both data and algorithms, our platform ensures that AI models adhere to stringent ethical standards and comply with evolving regulatory frameworks. This rigorous approach minimizes the risk of discriminatory outcomes, bolstering stakeholder trust and confidence in AI-driven solutions.
At Lumenova AI, we understand that trust extends beyond addressing concerns about job displacement or automation.
-
Our platform provides real-time insights into AI decision-making, making it easier to trust and adopt.
-
Lumenova AI aligns GenAI with clear business outcomes, turning skepticism into confidence.
-
Built-in frameworks ensure AI meets the highest standards of accountability and security.
By tackling the trust gap head-on, Lumenova AI accelerates GenAI adoption where it matters most—fostering confidence across the entire organization.
Real-World Case Studies
Below, we explore some real-world case studies from Google Cloud’s extensive portfolio of 185 use cases. These examples illustrate how leading organizations are leveraging GenAI to optimize operations, enhance customer experiences, and innovate at scale.
Alaska Airlines: Revolutionizing Travel Booking
Alaska Airlines has introduced a natural language-powered AI chatbot, offering a conversational interface that mimics interactions with a seasoned travel agent. This solution simplifies travel bookings, elevates customer experiences, and reinforces brand loyalty through hyper-personalized services.
Formula E: Enhancing Sports Engagement
Formula E utilizes GenAI to condense two-hour race commentaries into concise, two-minute multilingual podcasts. By incorporating real-time driver data and seasonal storylines, they’ve successfully broadened their audience reach.
UPS Capital: Optimizing Deliveries with AI
UPS Capital employs a machine learning solution called DeliveryDefense Address Confidence. This tool assigns a confidence score to delivery addresses, helping businesses anticipate and prevent delivery failures. It’s a game-changer in improving logistics reliability and customer satisfaction.
Volkswagen: AI-Powered Virtual Assistance
Volkswagen’s “myVW” app features an AI-driven virtual assistant that answers queries such as, “How do I change a flat tire?” or explains dashboard indicators via multimodal capabilities. By pointing their smartphone camera at their car’s dashboard, users gain immediate context and support.
Snap: Engaging Users with AI
Snap deployed the multimodal capabilities of Gemini in its “My AI” chatbot, dramatically increasing user engagement by over 2.5 times. This integration enhances the personalized user experience within their platform, ensuring seamless and interactive communication.
IHG Hotels & Resorts: Redefining Guest Services
IHG Hotels & Resorts is integrating a GenAI-powered chatbot into its mobile app, enabling guests to seamlessly plan vacations. The system offers personalized suggestions, simplifying the travel planning process and improving customer satisfaction.
Bayer Crop Science: Revolutionizing Agriculture
Bayer’s Climate FieldView platform integrates AI to analyze billions of data points and provide farmers with actionable insights for better field management. The result: higher crop yields, more efficient fertilizer use, and a reduced carbon footprint.
Mercedes-Benz: AI for E-Commerce and Call Centers
Mercedes-Benz combines AI with its e-commerce capabilities to deliver smarter sales assistants. The company also leverages AI in call centers and marketing campaigns, enhancing both customer support and personalization.
Mark Cuban’s Cost Plus Drugs: Streamlining Operations
The company utilizes Gemini AI to simplify operations, from transcriptions to FDA compliance documentation. Employees save an estimated five hours per week with AI-powered automation, enabling them to focus on more strategic tasks.
ADT: Empowering Home Security Choices
ADT employs a customer-facing AI agent to help users select, purchase, and configure their home security systems with ease. This innovation enhances the customer journey, making complex setups more accessible and intuitive.
Reflective Questions
Before addressing the challenges and opportunities of scaling GenAI, consider the following reflective questions to guide your strategy and decisions:
-
What measures will you put in place to ensure data privacy and security as you prepare to scale GenAI?
-
How will you address and reduce potential bias in the data used to train your AI models?
-
How can you make sure your future AI models provide insights that are understandable to both users and stakeholders?
-
What processes will you use to detect and manage inaccuracies or “hallucinations” in AI-generated outputs?
-
What ethical considerations and intellectual property policies will guide your use of generative AI?
-
How will you balance the costs associated with the high computational demands of generative AI?
-
How can you clearly communicate the capabilities and limitations of AI to build trust with your teams and users?
-
How will you support your employees through the changes that come with AI-enhanced workflows?
-
Are there plans for training programs or upskilling to help teams adjust and excel?
-
How will you define and measure success for your GenAI initiatives?
-
How will you ensure that your AI practices remain adaptable to future regulatory standards?
Thinking through these questions can help your organization anticipate potential challenges in AI governance and compliance. When you’re ready, Lumenova AI provides a powerful framework to simplify these processes, offering proactive risk management, compliance tracking, and automated assessments to support a smooth and responsible integration journey. Book a demo to learn how we can be your partner in building a strong foundation for AI governance and risk management.
Conclusion
GenAI has the potential to reshape the way we work, innovate, and interact—but deploying it successfully is like solving a carefully crafted puzzle. Each piece, from data security and ethical governance to integration and user trust, needs to fit perfectly to make AI not only powerful but also dependable and fair.
When these elements come together, AI evolves from being a mere tech novelty into a trusted collaborator, driving meaningful progress and fostering trust at every level. The challenges we’ve discussed in this series are all critical pieces of this puzzle. By addressing each thoughtfully, you’re not just implementing an AI system; you’re establishing a framework where AI-driven innovation aligns with ethical standards, transparency, and accountability—ensuring its impact is sustainable and long-lasting.