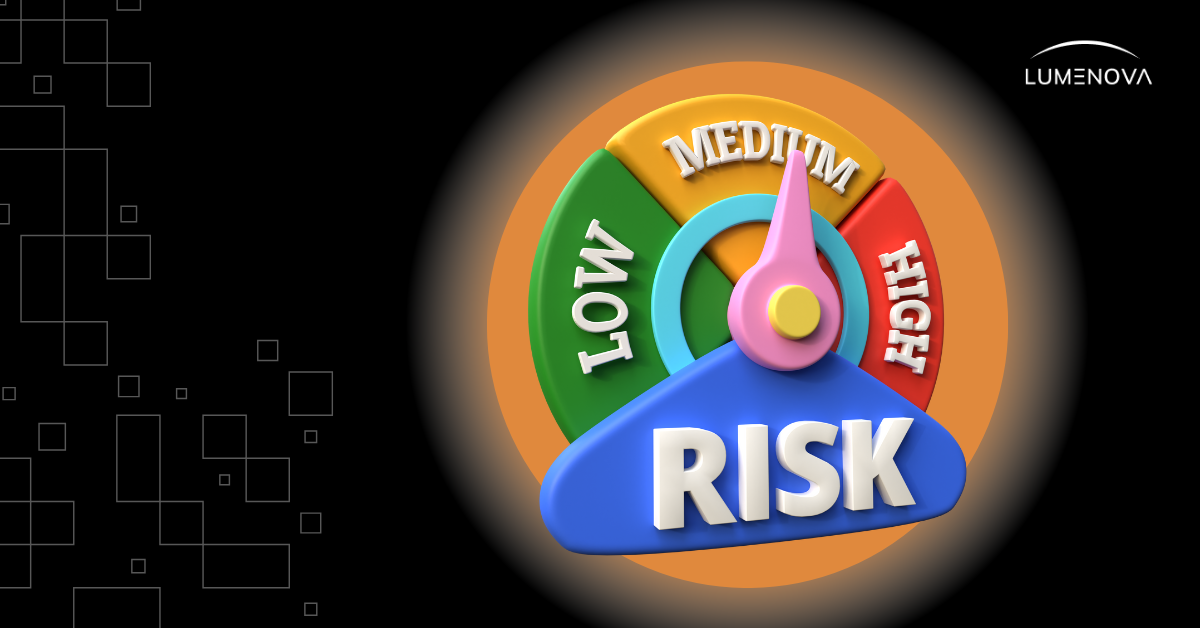
Contents
AI technology is evolving rapidly, and developers are marketing AI tools that promise some pretty incredible benefits. From accelerating the underwriting processes and enabling real-time fraud detection to smarter, algorithmic trading strategies and automating complex claims workflows, the possibilities can seem endless. These tools have the potential to improve the client experience, increase customer loyalty, protect your organization from fraud - ultimately, these benefits all aim to boost your bottom line.
With these shiny possibilities, though, also comes a growing set of AI risks that shouldn’t be ignored. Many AI models function as opaque black boxes, making decisions that are difficult to interpret or justify. When left unchecked, these systems can reinforce biases, make unfair predictions, or even violate compliance mandates. If you’re already operating in an industry under intense regulatory scrutiny, such missteps can lead to serious consequences. If not deployed thoughtfully and responsibly, AI intended to increase profits could lead to reputational and legal consequences.
Even worse, without a thorough AI risk assessment strategy, your organization could even inadvertently amplify these issues. What starts as a small oversight in a model’s training data or logic can evolve into a systemic liability, amplified by automation and embedded into critical business functions. In industries where trust and accountability are non-negotiable, the failure to govern AI responsibly isn’t just a technical issue. It’s a strategic and compliance risk.
What’s at Stake? AI Risks Financial and Insurance Firms Can’t Ignore
As AI becomes increasingly integrated into business operations, AI risks become greater and more difficult to avoid without an intentional strategy. AI can help to inform high-stakes decisions like lending, claims approvals, and fraud detection, but using it in these key processes also increases the risks associated with its performance, fairness, and oversight.
Bias & Discrimination
Models trained on flawed or unbalanced data can unintentionally reinforce discriminatory patterns. For example, an AI might approve loans for one demographic at a higher rate than another when all other factors remain the same. In industries where fairness is both a legal and ethical imperative, these outcomes can lead to investigations, lawsuits, and loss of customer trust.
Explainability Failures
Many high-performing models are inherently complex, making it difficult to understand how decisions are made. But in regulated sectors, “we don’t know how the model works” is not an acceptable answer. Without explainability, organizations struggle to justify decisions to regulators, auditors, and even customers — putting them at risk of noncompliance and public scrutiny.
Model Drift & Performance Degradation
AI systems don’t operate in a static world. Over time, changes in user behavior, market conditions, or data sources can cause models to degrade silently. This drift can lead to inaccurate predictions, improper decisions, or unnoticed policy violations.
Reputational Damage
AI-driven errors can quickly make headlines, especially in the financial and insurance industries that impact the lives of real people in significant ways. Companies are being held accountable for the algorithms they utilize, and transparency is far from optional.
Regulatory Sanctions & Fines
With frameworks like the EU AI Act and evolving national policies on automated decision-making, regulators are watching closely. Failure to demonstrate adequate oversight, documentation, or risk controls can lead to substantial penalties.
An Effective AI Risk Assessment Strategy is the Cornerstone of Responsible AI
Deploying AI on a grand scale at your organization requires structured, repeatable risk oversight. A robust AI risk assessment strategy serves as the foundation for building systems that are not only high-performing, but also fair, explainable, and compliant.
An AI risk assessment should go far beyond model accuracy. It includes a comprehensive evaluation across technical, ethical, and regulatory dimensions. That means examining how models perform under different conditions, how decisions are justified, and whether outcomes align with both internal policies and external legal requirements.
Key components of an effective AI risk assessment include:
- Bias and Fairness Checks: Ensuring decisions don’t systematically disadvantage certain groups
- Robustness and Stress Testing: Validating model behavior under edge cases or shifting data
- Explainability Audits: Verifying that model logic can be clearly communicated to non-technical stakeholders
- Data Lineage & Provenance: Tracking the origins, handling, and quality of input data
- Compliance Mapping: Aligning model usage with evolving regulations like the EU AI Act, GDPR, and industry-specific standards
These assessments should also take place on an ongoing basis. Since model risk evolves over time, your AI governance practices should keep that pace. Ultimately, a structured AI risk assessment strategy doesn’t just protect your organization. It enables responsible innovation.
AI Risk is Inevitable, but Mismanagement Isn’t
If you’re ready to begin your first AI risk assessment, then you should know that the next step once you’ve assessed your position is to develop your AI risk management strategy. The Lumenova AI platform can help. Book your demo today and one of our consultants will help you to understand how to proceed.