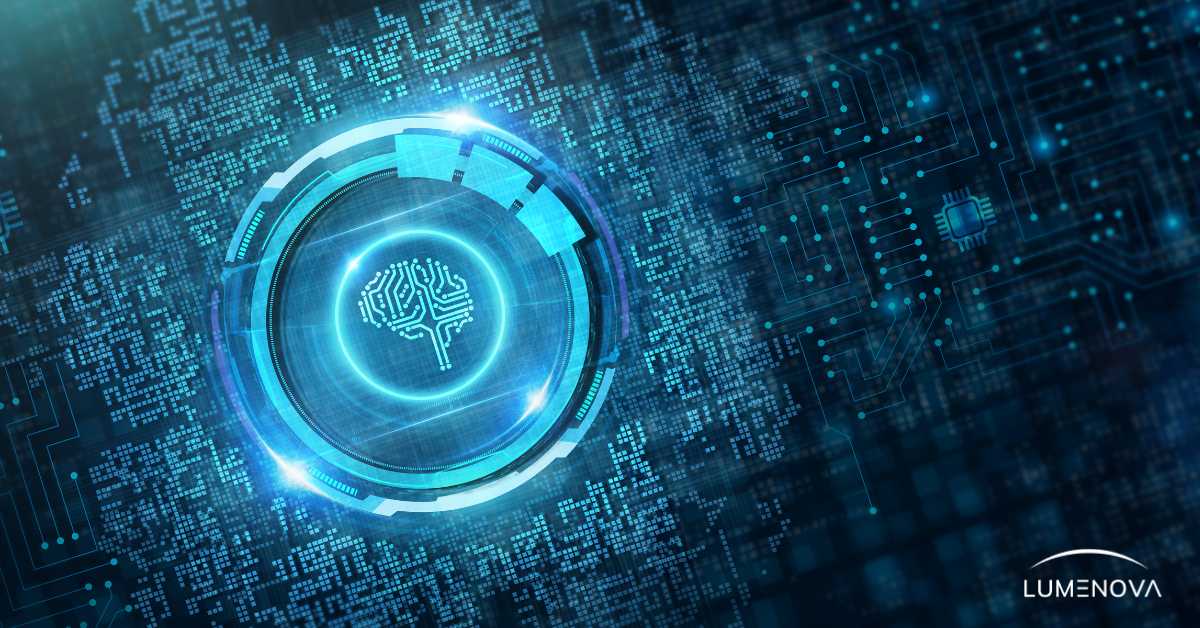
Contents
In a world of rapid technological progress, where automation and machine learning (ML) are permeating vast areas of our lives, several questions still remain:
What about AI bias? And what does fairness truly stand for in machine learning?
Algorithmic bias remains a growing subject of debate in the use of AI. It is a complex problem that stands to bridge the path between mathematical analysis and the social implications of determining what is ‘fair’ in decision-making.
Nowadays, machine learning-powered products stand behind a number of important decisions which can directly impact our lives and well-being. Algorithms are used to predict:
- Who should get hired
- Whose loan is approved or denied
- What price a customer is willing to pay for a product or service
However, AI tools trained on historical data can carry pre-existing biases. For example:
- Recruitment algorithms can be biased against women
- Facial recognition software is worse at detecting darker skin
Despite the new opportunities offered by machine learning, there is an ongoing risk that algorithms may identify and use biased patterns in decision-making. Not only can AI-driven products replicate these biases, but they can also further exacerbate existing inequalities.
Understanding the source of AI bias
The primary source of AI bias is the data it was trained on. Unknown to us, this data may contain historical patterns of inequality and discrimination which the algorithm subsequently integrates into its model. Alternatively, AI bias can be a misrepresentation of the ground truth which can arise through inappropriate data collection.
Disparities that emerge in the design of an AI system must, first and foremost, be understood. We must ask ourselves whether they are harmful or justified and whether they are a reflection of potential discrimination.
Philosophy and sociology can help us understand the importance of demographic criteria when it comes to achieving fairness in machine learning, but this is a relatively new direction. As such, the varied perspectives on the subject are often in tension. After all, fairness is situational. It’s not an absolute, one-size-fits-all condition.
How data collection and feedback impact fairness in machine learning
Data collection and AI bias
When we think of ‘measurement’, we usually imagine a clear and straightforward process, an objective analysis of the world as it is. In fact, measurement is a chaotic, subjective, and challenging process.
In a world that refuses to fall neatly into a predefined set of checkboxes, the answer to a seemingly straightforward question might be fraught with assumptions and incorrect stereotypes.
Categories such as race cannot be considered stable, as their measurement depends on our ever changing conceptions and views of the world. In truth, evaluating most human-related attributes is subjective and difficult.
Another relevant example of how data subjectivity can lead to disparity can be found in using AI to measure employee performance. In theory, the efficiency of an employee could be quantified by using performance review scores. However, these scores are determined by managers who could have a bias against one group or the other, thus preventing some employees from reaching their full potential.
As described by Barocas et al, training data can reflect various patterns, sometimes encoding a series of biases and distortions picked up from the real world through the measuring process.
Useful data
“Smoking is associated with cancer” represents the knowledge that we might need to mine using machine learning.
Biased data
“Men are better at engineering compared to women” is a stereotype that we would like to avoid.
Machine learning algorithms have no way of distinguishing between the different kinds of patterns encoded in the training data. With no intervention to mitigate bias, AI models will extract stereotypes in the same manner in which they draw out useful knowledge.
Biased Feedback Loops
Another important gateway through which bias can corrupt an AI system is through feedback. Self-fulfilling predictions are a relevant example in this type of situation.
For example, an algorithm created to predict home sales prices is likely to create a self-fulfilling feedback loop. In this case, houses predicted to have a lower sale price will deter potential buyers, suppressing market demand, and ultimately lowering the price.
This type of effect can also be observed in the case of predictive policing systems. If a specific neighborhood is designated as a high-risk area by the algorithm, more police officers will be deployed to cover it. Naturally, an increase in the number of officers – who might also unconsciously lower their threshold for arresting people because of the initial prediction – will ultimately lead to its validation, regardless of whether or not it was true in the first place.
Striving for fairness in machine learning
The Algorithmic Accountability Act of 2022
The Algorithmic Accountability Act of 2022 strives to protect consumers from AI bias by requiring organizations to ensure a transparent view of the uses and behind-the-scenes processes of automated systems. The Bill’s goal is to empower the public to make informed decisions, based on where and why AI tools are being used for decision-making.
Behind the introduction of The Algorithmic Accountability Act are numerous reports of biased AI systems, which could have been mitigated by appropriate testing.
If passed, the Act would require organizations employing AI or algorithm-based decision-making to perform annual impact assessments and submit these results to the Federal Trade Commission.
The Algorithmic Accountability Act is not the only regulatory initiative that has been introduced. Other federal-level initiatives include:
💡 The White House’s Blueprint for an AI Bill of Rights
💡 The Equal Employment Opportunity Commission’s AI and Algorithmic Fairness Initiative
💡 The National Institute of Technology’s AI Risk Management Framework
Now more than ever, the expectation is for AI to produce transparent and understandable results that reflect the ethical standards of society.
Key takeaways
While there are fundamental challenges and limitations when it comes to achieving fairness in machine learning, striving to understand when disparities become unjustified, unacceptable, or downright harmful should come as a first step.
Machine learning-driven processes aren’t fundamentally flawed, and data-driven decision-making can become more transparent by employing explainability tools and techniques to mitigate AI bias.
Lumenova AI can help companies understand the inner workings of their AI models, so they can efficiently mitigate bias and remain compliant with algorithmic regulations at all times.
To find out more about how your business can benefit from Responsible AI, please get in touch with us.