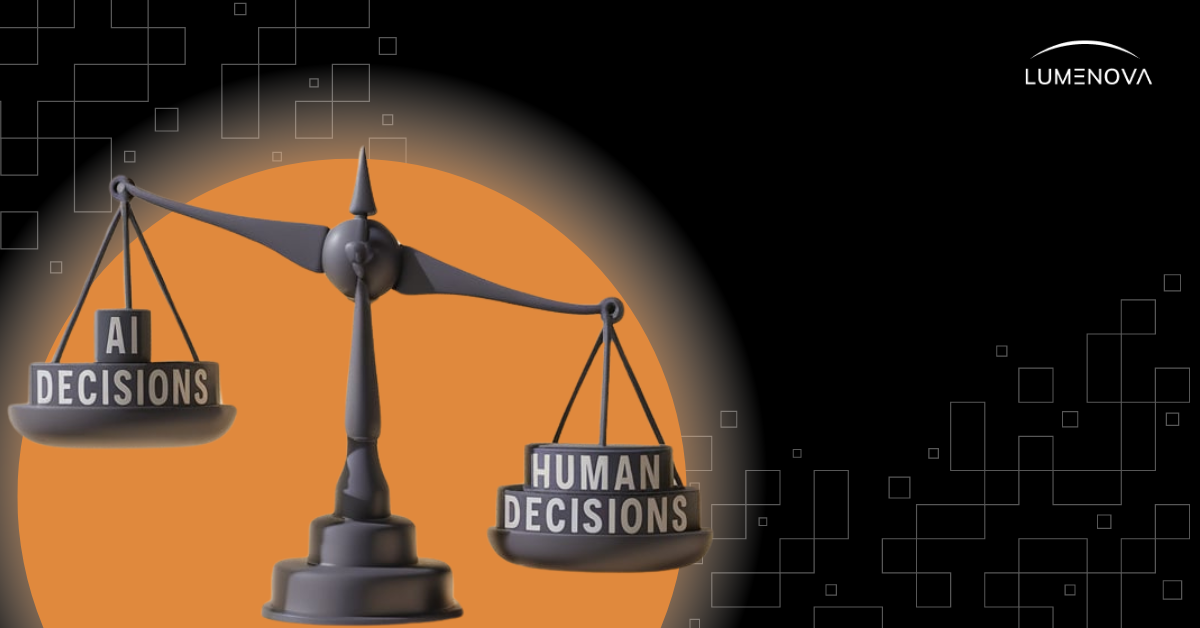
In our high-tech age, AI serves as a crucial tool, enhancing innovation and operational performance across various industries, including finance, banking, and healthcare. For leaders like CEOs and CTOs, AI offers the potential to transform their organizations, delivering insights that can revolutionize business strategy and decision-making. However, with the rapid adoption of AI comes the risk of overreliance—a subtle but significant challenge where human judgment is overshadowed by the perceived infallibility of AI systems. This phenomenon, known as automation bias, poses a critical risk, particularly in sectors where decisions can have profound impacts, from patient care in hospitals to financial investments in global markets.
The implications of automation bias are far-reaching. As AI systems become more complex and their outputs more influential, the danger of accepting AI recommendations without sufficient scrutiny grows. This overreliance can lead to errors that not only undermine the effectiveness of AI but also jeopardize the very goals that organizations seek to achieve through digital transformation. Understanding the nuances of overreliance on AI is essential for business leaders who are responsible for navigating the delicate balance between leveraging advanced technologies and maintaining rigorous oversight.
This article explores the causes, mechanisms, and consequences of overreliance on AI, offering insights and strategies to mitigate this risk and ensure AI is used to its fullest potential without compromising human judgment.
What Is Overreliance on AI, and Why Is It a Problem?
Overreliance on AI occurs when users accept AI recommendations without sufficient scrutiny, often due to various contributing factors. The drive for efficiency and productivity can lead users to bypass critical evaluation, as questioning AI outputs requires additional effort. Additionally, the inherent persuasiveness of AI—AI must always produce an output in response to a given input—thus further creating a false sense of expertise, particularly in areas where users lack knowledge.
For example, consider a user without a strong background in math who encounters AI-generated explanations in Euclidean geometry. Without the necessary expertise, they might take the AI’s responses at face value, assuming they’re accurate simply because they come from a machine. This issue is often exacerbated by the misconception that AI functions like a search engine, leading users to expect the same level of reliability from AI outputs as they would from verified search results.
Additionally, when users begin to anthropomorphize AI—assigning it human-like qualities—they may develop an even deeper level of trust, accepting its recommendations without question. These factors collectively contribute to automation bias, potentially causing serious errors in areas like healthcare, where diagnostic AIs might overlook critical patient details, or in finance, where flawed trading algorithms could result in significant losses.
What Factors Contribute to Overreliance on AI?
- Lack of AI literacy and expertise: Many users are not fully aware of how AI systems work, leading them to trust outputs without understanding the underlying processes. For example, a financial analyst might rely heavily on AI-driven market predictions, unaware of the limitations or biases embedded in the algorithms.
- Task familiarity/unfamiliarity: Both familiarity with a task and unfamiliarity with it can lead to overreliance on AI, but in different ways. If users are well-versed in a task, they might assume that AI can handle routine decisions flawlessly and thus become complacent, thinking less oversight is needed. Their confidence in their own understanding can make them overlook the nuances that the AI might miss. On the other hand, users who aren’t familiar with a task may lean heavily on AI, trusting its outputs more than they should because they lack the expertise to question its accuracy. This reliance on AI can be driven by a lack of domain expertise. In both cases, whether users feel too confident or too trusting, the crucial human role in checking and validating AI outputs can be diminished, leading to errors when the AI makes mistakes that go unnoticed.
- User confidence: User confidence can exacerbate the problem of overreliance on AI, especially in high-stakes environments. This overconfidence is often driven by a tradeoff between convenience and trust rather than simple blind faith in the technology. For example, in autonomous driving, drivers might overestimate their ability to supervise the system due to the convenience and efficiency promised by the technology. They might become complacent, relying heavily on the AI’s capabilities to manage driving tasks, while underestimating the need for their own vigilance. This tradeoff between a seamless, hands-off experience and the actual need for active monitoring can lead to critical accidents when the AI encounters situations it cannot handle or when intervention is necessary but not timely.
How Do Cognitive Biases Reinforce Overreliance on AI?
The tendency to over-rely on AI often stems from cognitive biases that quietly influence user interactions with these technologies.
- Automation bias: This bias leads users to favor AI-generated information over other sources, especially in complex tasks where AI is assumed to be more reliable. In legal settings, for instance, judges might give undue weight to AI-generated risk assessments, potentially sidelining their own expertise and judgment.
- Confirmation bias: Users may be inclined to accept AI recommendations that align with their preconceived notions or expectations. In hiring processes, for example, AI-driven assessments that confirm a recruiter’s initial impressions might go unquestioned, even if they perpetuate existing biases.
- Ordering effects: The sequence in which AI recommendations are presented can strongly influence user trust. If an AI system provides accurate recommendations early on, users may develop unwarranted trust, which persists even when the AI makes errors later. In customer service, this can lead to overreliance on AI chatbots, resulting in poor handling of complex customer issues.
- Overestimating explanations: Detailed explanations from AI systems can sometimes create a false sense of security, leading users to trust the AI more than is warranted. In sectors like finance, where AI models generate complex investment strategies, users might rely too heavily on the AI’s reasoning without fully understanding the underlying assumptions or risks.
- Expertise Bias: Users may excessively defer to AI systems due to the belief that the technology, being advanced and complex, must be more accurate and reliable than human judgment. This bias can lead them to undervalue their own expertise and critical thinking, particularly in scenarios where nuanced decision-making is essential.
- Affect Heuristic: Users’ decisions are sometimes influenced by their emotional reactions to AI outputs rather than an objective assessment of the information. For example, they might favor AI recommendations that elicit positive feelings or align with their emotional state, even if these recommendations are not the most accurate or appropriate.
- Availability Heuristic: Users tend to rely on information that is most readily available or recent, which can distort their perception of AI’s overall accuracy. For instance, if an AI has recently provided helpful or correct information, users might overestimate its reliability, trusting it more despite potential limitations in its broader performance.
- Framing Bias and Narrative Fallacy: The way AI outputs are framed and presented can significantly impact their perceived credibility. AI recommendations that are delivered in a compelling and coherent narrative may appear more convincing, even if they lack strong evidence or are based on incomplete data. This can lead users to trust the AI’s outputs more than they would if the information were presented in a more neutral or fragmented manner.
- Representativeness Heuristic: Users might treat AI models as if they are analogous to search engines due to their similar interface and interaction style, leading to an overestimation of the AI’s accuracy and reliability. This heuristic can cause users to expect that AI systems will provide information as consistently and accurately as traditional search engines, which can result in misplaced trust in the AI’s outputs.
What Are the Consequences of Overreliance on AI?
The consequences of overreliance on AI can be severe, affecting both individual decision-making and broader organizational outcomes:
- Skills Degradation and Reduced Critical Thinking: Overreliance on AI can lead to a decline in essential cognitive skills. When users depend too heavily on AI, they may experience decreased critical thinking and cognitive laziness. One study found that students who relied extensively on AI dialogue systems showed a reduction in their decision-making abilities. Specifically, 27.7% of students demonstrated degraded decision-making skills due to their reliance on AI for academic tasks. This suggests that overreliance can erode critical cognitive skills necessary for effective problem-solving and collaboration in various contexts.
- Decreased human-AI team performance: When users fail to critically evaluate AI outputs, the effectiveness of human-AI collaboration can suffer. This undermines the combined performance of the human-AI team, often leading to suboptimal decisions and inefficiencies. The lack of rigorous oversight can diminish the potential benefits of AI, affecting overall team productivity and decision quality.
- Erosion of trust in AI: As users become aware of the mistakes made by AI—especially those that go unchecked—their trust in these systems can diminish. This loss of trust can lead to the underutilization of AI tools, depriving organizations of the benefits that these technologies can offer when used appropriately.
A notable example occurred with the use of AI in the criminal justice system, particularly in the case of the COMPAS algorithm, which is used to assess the likelihood of a defendant reoffending. An investigation revealed that the algorithm was biased against African American defendants, incorrectly labeling them as higher risk for recidivism compared to white defendants. The study found that the algorithm was wrong 44% of the time when predicting the likelihood of reoffending for black defendants, leading to significant concerns about fairness and accuracy in the justice system. This revelation not only prompted public outrage but also led to a decline in trust in AI systems used in legal contexts. Despite these issues, many jurisdictions continue to use COMPAS and similar tools, reflecting the complexities and challenges of moving away from established practices. The situation underscores how unchecked mistakes can lead to distrust and reluctance to adopt or adapt AI solutions that could otherwise enhance decision-making processes.
- Increased risk of critical errors: In high-stakes environments, overreliance on AI can lead to catastrophic outcomes. In the financial sector, a report from the European Central Bank noted that overreliance on AI models could render the financial system more vulnerable to operational flaws, failures, and cyberattacks. The study emphasized that substituting AI resources for human oversight in core functions could amplify risks associated with AI, potentially leading to market disruptions and financial instability.
- Cascading Failures: Overreliance on AI can lead to cascading failures within an organization, where an initial error or misjudgment based on AI outputs triggers a series of negative consequences. For instance, if an executive makes a strategic decision relying solely on flawed AI-generated insights, the repercussions of this decision can ripple through the entire organization, affecting multiple departments, operations, and even the company’s market position. This is particularly concerning when decisions are made without adequate human oversight or without questioning the validity of AI recommendations, leading to a chain reaction of adverse outcomes that can undermine the organization’s overall stability and performance.
How Can Organizations Mitigate Overreliance on AI?
To effectively mitigate the risks associated with overreliance on AI, organizations can implement several key strategies.
Educating Users About AI Capabilities and Limitations
Educating users about the capabilities and limitations of AI is imperative. Comprehensive onboarding should include examples of both correct and incorrect AI outputs, helping users understand the variability in AI performance. This education also needs to address the cognitive biases that contribute to overreliance, ensuring users are equipped to critically assess AI recommendations. Additionally, users need to be proficient in using AI systems so they can differentiate between effective and ineffective use. Understanding how to leverage specific AI capabilities within particular contexts is crucial for delivering optimized results. For instance, Microsoft provides training sessions for users of its AI-powered tools like Cortana and Azure AI, demonstrating scenarios where AI excels and where it might falter, helping users develop a balanced understanding of AI’s capabilities.
Transparency and Explanation
Transparency in AI systems is vital for building a nuanced understanding of AI’s reliability. Providing clear, accessible explanations of how AI decisions are made—along with information about the system’s limitations—helps users maintain a healthy level of skepticism and encourages them to engage in critical evaluation rather than blind acceptance. For example, IBM’s Watson for Oncology offers detailed explanations for its treatment recommendations, including the data sources and logic behind each suggestion, allowing oncologists to critically evaluate AI’s input before making clinical decisions.
Real-time Feedback and Cognitive Forcing Functions
Real-time feedback mechanisms can alert users when they are over-relying on AI, particularly in situations where the AI’s recommendations may be flawed. Cognitive forcing functions—interventions designed to prompt critical thinking—can be especially effective in reducing overreliance by encouraging users to reflect on AI outputs and consider alternative possibilities before making decisions. For instance, in the aviation industry, Boeing incorporates real-time alerts and cognitive forcing functions in its flight control systems, prompting pilots to reassess AI-generated commands during critical flight phases, thus preventing overdependence on automated systems.
Personalization and Adaptive Systems
AI systems should be designed to adapt to the needs and characteristics of individual users, such as their level of expertise and confidence. For example, novice users might benefit from more detailed explanations and guided prompts to help them verify AI outputs, while more experienced users could benefit from tools that not only provide detailed insights but also challenge their assumptions and encourage deeper critical engagement with AI-generated insights. However, this personalization could also lead to a double-edged sword scenario—by tailoring interactions too closely to individual preferences, AI systems might feign a deeper understanding than they actually possess, giving users a false sense of security in the AI’s reliability. It’s crucial to balance personalization with transparency, ensuring that users remain aware of the system’s limitations regardless of how customized their experience becomes.
How Do We Balance Trust and Skepticism in AI?
Overreliance on AI can undermine the potential benefits of these technologies, leading to poor decision-making and eroding trust. By understanding the causes and mechanisms of overreliance and implementing targeted strategies to mitigate these risks, business leaders can ensure that AI serves as a powerful tool for enhancing human judgment, rather than replacing or diminishing it. This balanced approach is crucial for maintaining the integrity of decision-making processes and ensuring long-term success in an AI-driven future.
Reflecting on this topic, consider the following questions:
- How can your organization ensure that AI is used to enhance, rather than replace or diminish, human judgment?
- What steps can be taken to educate users about the capabilities and limitations of AI?
- How can you balance the need for trust in AI systems with the necessity for critical oversight?
Lumenova AI is committed to helping organizations navigate the complexities of AI adoption. Our platform ffers comprehensive tools to manage AI governance, mitigate risks, and ensure compliance with industry standards. Request a demo today to learn how we can help your company achieve its responsible AI initiatives.