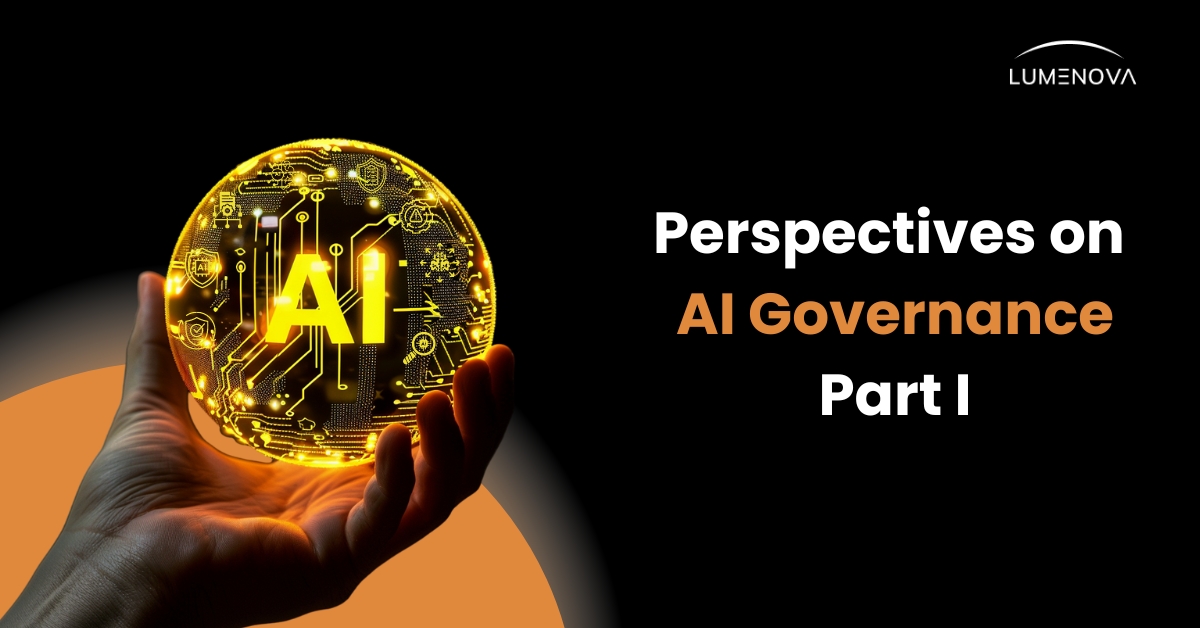
Contents
According to the Microsoft Work Trend Index Report, at least 7 out of 10 workers across all age groups from Boomers (73%) to Gen Z (85%) now actively leverage AI in a workplace setting, whereas 71% of leaders would rather hire less experienced employees with AI skills over those with more experience and minimal AI skills. Additionally, 79% of leaders view AI adoption as critical due to competitive industry pressures despite ongoing uncertainty about how best to align AI integration efforts with an organization’s vision, strategy, and business requirements.
As soon as 2030, 50% of jobs could be automated by AI, and over the next decade, generative AI (GenAI) alone could deliver several trillions of dollars in productivity gains to the global economy. In 2023, global AI spending exceeded $150 billion, and current predictions estimate that by 2030, global AI market volume will surpass $800 billion.
This year also marked the birth of the world’s first comprehensive AI legislation, the EU AI Act. But the EU isn’t alone in its AI regulation efforts—Japan, the US, UK, UAE, Singapore, Israel, Brazil, and Egypt, as well as several other nations across South America, Africa, and Central and Southeast Asia have all, at the very least, taken tangible steps toward establishing and executing national AI governance strategies. This is not to mention The Global Partnership on AI, which was established in 2020 and includes over 20 diverse nations from disparate regions around the world.
While AI governance is integral to addressing AI disruption and transformation, alone, it can’t yet ensure that all AI risks, benefits, and impacts are adequately managed, especially throughout the AI lifecycle. Consequently, responsible AI (RAI) principles and risk management guidelines have emerged as critical resources, and once more, at the global scale. From the US-based NIST AI Risk Management Framework (NIST AI RMF) and Switzerland’s ISO 42001 standard to the private sector, where major international players such as Microsoft, IBM, and McKinsey now offer RAI toolkits, providing services like RAI consultations, impact, and risk assessments. The OECD, UNESCO, ASEAN, and NIST have also outlined and committed to core RAI principles, many of which are shared among them and will likely make their way into future AI regulations.
We could continue sifting through this kind of information for hours, but that’s not what readers are here for. So, what does all of this mean? Below, we highlight some important trends we’ve thus far, only alluded to:
- The idea that “AI adoption isn’t optional” is becoming mainstream, especially in professional and business-oriented contexts. Still, while most people are eager to reap the benefits that AI inspires, many remain weary of the risks and potential adverse impacts it could generate, and many more don’t even know where to begin when it comes to adopting AI and building AI skills.
- People are quickly adopting AI in their professional lives—of the AI users surveyed in Microsoft’s report, 46% adopted AI within the last 6 months—and while businesses are excited about AI-driven opportunities, there’s still a lot of uncertainty surrounding how best to integrate and align AI with organizational needs and objectives.
- The world is waking up to the importance of AI regulation. AI regulation strategies will differ between countries and districts but businesses, regardless of where they choose to operate, will eventually have to deal with some form of AI-specific compliance requirements, and this time will come sooner rather than later.
- AI ethics and governance are deeply interconnected. While the nature of this connection might be inconsistent depending on where you are in the world, ethics will continue to influence governance and legislation, just as it always has.
- The pace of AI innovation and proliferation is still accelerating. Frequent readers might note that we’ve often talked about AI as an exponential technology, so we’ll use this point as a reminder: AI moves much faster than you think, perceive, and expect.
Each of these trends reinforces the importance of AI governance, not only as a guide, but as a mechanism to ensure that the value AI delivers is consistently optimized across different task domains without compromising human safety, agency, and dignity. On a more obvious note, AI governance is a powerful tool that organizations can leverage to comply with existing regulations and business requirements, foster RAI best practices, and build consumer and employee trust.
Therefore, in the following sections, we’ll discuss the elements that we think any AI governance framework should possess. We’ll begin by outlining fundamental properties, then core principles, and finally, mechanisms through which the framework can be maintained and enacted. In doing so, we hope to provide readers with a high-level guide on AI governance that can be fine-tuned for specific business environments.
For readers who’d like to venture further into the AI governance, risk management, and policy landscape, we invite you to follow Lumenova AI’s blog, where you can access a wealth of in-depth resources on these topics and several others.
Fundamental Properties
When we speak of fundamental properties, we mean broad characteristics of an AI governance framework. For example, built-in flexibility and adaptability would constitute a property whereas impact assessments, being much more specific and distinctly actionable, would be a mechanism. In other words, properties are what we use to describe the structure of an AI governance framework, and we illustrate the ones we believe to be essential below:
- Built-in adaptability and flexibility: As we know, AI moves incredibly fast, and this necessitates a governance framework that can adapt itself in light of ongoing AI innovation. Novel AI use cases frequently crop up, foreseeable risks are not always easy to address, and real-world impacts can be extremely difficult to measure, anticipate, and mitigate. Therefore, flexibility is paramount, especially as it concerns the ability to quickly adapt existing mechanisms and thresholds in response to AI-driven changes, irrespective of the form they take.
- A targeted scope: There’s no such thing as a one-size-fits-all AI governance strategy. AI’s value lies in its versatility—the ability to customize or finetune AI models and applications or integrate purpose-built AI tools across numerous task domains stresses the importance of context-specific AI governance protocols. Organizations must have a clear understanding of why they’re using AI, who’s using it and how, what risks are inspired by different use cases, and what AI objectives they hope to achieve.
- Risk prioritization: AI can inspire a variety of diverse risks throughout its lifecycle, but not all of them will warrant equal consideration. An AI governance framework should allow organizations to understand AI risks in terms of their saliency, probability, and real-world impacts so that they can be prioritized accordingly. In other words, most organizations simply won’t have enough resources to address all possible AI risks, so they’ll need to classify them categorically. This will also be crucial to complying with emerging AI regulations like the EU AI Act—which classifies AI systems according to a tiered risk classification structure—establishing the right risk tolerance levels, and mapping AI risks appropriately.
- Human-centric design: The main point of AI governance is to ensure that AI is developed, deployed, and utilized responsibly—for AI governance efforts to align with business and compliance requirements, organizational value propositions and overall mission, as well as common standards like trustworthiness, humans must be at their center. In simple terms, AI governance frameworks shouldn’t only mirror fundamental ethics and existing regulations but also maintain a transparent and easily interpretable structure whereby anyone subject to them can understand what’s required of them.
- Outlining clear roles and responsibilities: An AI governance framework that doesn’t outline clear roles and responsibilities for key personnel involved in AI development, deployment, and operations is virtually guaranteed to fail. Leaders and employees need to know what’s expected of them and how to adhere to these expectations in a way that doesn’t compromise or confuse other’s ability to do so. Clear AI-specific roles and responsibilities also allow an organization to hold key personnel accountable when things go south, which is important to consider as compliance requirements strengthen.
- Enabling organization-wide communication and feedback: An AI governance framework that doesn’t enable organization-wide communication and feedback will be far less adaptable and flexible, exhibit a scope that can easily be blurred, unnecessarily complexify the risk prioritization process, and compromise human-centric design by reducing human agency. All of these drawbacks will make compliance with business and regulatory requirements much more difficult, introduce avoidable bottlenecks and pain points into the AI operations process, and eventually compromise employee trust and accountability.
- Fostering continual organization-wide AI awareness and education: At the very least, an organization’s C-suite should possess a strong understanding of AI policy and ethics developments, necessary AI skills and use cases, and potential AI risks, benefits, and impacts. While leadership must undoubtedly maintain a more profound AI-specific knowledge base, it’s equally important that an organization’s workforce has direct access to AI training and awareness initiatives that outline what RAI use looks like and provide opportunities for upskilling and reskilling where relevant. Failure to do this could lead to many negative consequences, most notably, compliance penalties and reputational damages.
- Identifying and protecting AI assets: In addition to evolving into one of the world’s most valuable commodities, AI is rapidly embedding itself into many kinds of technologies operating at different scales. This process will create co-dependencies between AI and the technologies it powers or supports, and it’s therefore pivotal that organizations identify and protect their AI assets, especially in cases where they’re already deeply embedded in an organization’s operational infrastructure.
Core Principles
The principles we cover here represent a conglomeration of trustworthy AI, RAI, and/or ethical AI principles drawn from leading AI regulations, ethics frameworks, and risk management approaches, specifically the EU AI Act, NIST AI RMF, and OECD ethical AI principles. Moving forward, and for clarity’s sake, we’ll group these principles under the RAI category, since these terms are often used interchangeably—readers should also note that most (not all) of these principles concern AI development, deployment, or integration. Consequently, below, are described the core RAI principles that any AI governance framework should include:
- Safety: AI systems should never be designed, developed, deployed, or integrated in a way that compromises human health and safety. Concrete measures must be taken to ensure that AI systems are tested and validated for safety prior to deployment or implementation, and organizations should always leverage RAI tactics and toolkits at their disposal.
- Security: AI systems and the data on which they’re trained should be securely operated and managed throughout their lifecycle. Organizations should also note that as they integrate more AI applications or models into their larger AI system, the corresponding attack surface area increases, expanding the breadth of potential cybersecurity vulnerabilities. In this context, cybersecurity measures must be established for data security, AI lifecycle management, and the networks and cloud infrastructures that support AI integration.
- Privacy: AI systems should not be designed, developed, deployed, or integrated in a way that compromises human privacy. For example, leveraging AI systems to infer sensitive data characteristics of users or employees, or alternatively, for intrusive workplace monitoring practices, would constitute an invasion of human privacy. Moreover, when AI systems leverage sensitive data for training or other purposes, this data must be sufficiently protected, either through anonymization or encryption techniques, or both.
- Robustness: AI systems should be intentionally designed and developed to perform reliably and consistently across changing environments and/or throughout novel circumstances. This is especially important in cases where systems are leveraged within consequential decision-making or high-impact contexts, seeing as consistency and reliability are key aspects of AI safety and performance monitoring.
- Resilience: AI systems should be intentionally designed and developed to be resilient to adversarial attacks or potential catastrophic events, whether they are AI or human-driven. In other words, if one component of an AI system fails, this shouldn’t result in the entire system crashing. Conversely, if a catastrophic event does occur, a strategy must be in place that allows the organization in question to quickly recover without severely comprising operations.
- Transparency: The intended purpose, design, function, use, and potential impacts of an AI system should be clearly documented, monitored, and communicated to all relevant interested parties, such as AI vendors, employees, or end users. Moreover, organizations that fail to adhere to transparency standards will have to work hard to overcome compliance hurdles which could result in significant penalties and/or sanctions.
- Accountability: Whether they are organizations or individuals, mechanisms that allow us to hold key actors accountable for the impacts that AI generates must be established. In this respect, accountability doesn’t only constitute a crucial dimension of compliance and RAI best practices, but also consumer and employee trust.
- Explainability: The outputs that an AI system generates should be explainable in terms of the logic by which they are arrived at, and the design/function of AI systems should be interpretable such that we can understand, in simple terms, the role a system plays and the computational processes through which it produces a given output. It’s worth noting that with some kinds of AI systems, namely large models that utilize deep learning architectures, the ability to holistically explain their inner workings and logic is extremely difficult if not impossible (for now)—some might know this as the “Black Box” problem. In such cases, model testing and validation may more heavily rely on other principles such as safety, robustness, resilience, and fairness.
- Fairness: AI systems should not be designed or leveraged to facilitate biased decisions or discriminatory outcomes. In fact, when it comes to utilizing AI in high-stakes decision-making contexts, fairness is one of the most important principles to adhere to, especially when considering that it falls at the heart of many emerging AI regulations and is present in virtually every single RAI and AI risk management framework.
- Validation and verification: Before deployment, AI systems should be validated and verified for safety, efficacy, robustness, resilience, fairness, and privacy. While validation will not always be required across each of these domains, and may sometimes even be necessary across others not mentioned here, one thing is clear: verification and validation can’t happen in the absence of well-defined testing and feedback mechanisms.
While some of these RAI principles may appear more important than others, each one is crucial in its own way. However, the weight that organizations decide to attribute to each of these principles will depend on their business and compliance requirements, mission statement and value proposition, operational and workflow structure, and finally, the resources they have at their disposal.
Mechanisms
The mechanisms we discuss in this section are all important, however, determining which ones to implement in an AI governance framework will depend on organizational context, resources, and needs. That being said, a mature AI governance framework would likely include most if not all the mechanisms described below:
- Risk assessments: Ideally throughout the whole lifecycle, but most importantly before deployment, AI systems must undergo risk assessments. These assessments are especially crucial for high-risk AI systems and should aim to identify foreseeable and preventable risks across all categories from localized to systemic, and by reference to AI risk management standards and guidelines. Risk assessments should also be continuously administered but maintain enough flexibility and adaptability to account for novel or changing AI risk profiles.
- Risk management procedures: Once AI risks are identified, they must be prioritized in line with pre-determined risk thresholds, compliance requirements, and organizational needs. Simply categorizing AI risks, however, is insufficient—an organization should establish targeted risk management procedures specific to its AI objectives, use cases, value proposition, and risk assessment requirements. To ensure that such protocols remain relevant and effective, some degree of built-in adaptability and flexibility is also required here.
- Impact assessments: AI systems should undergo impact assessments before deployment and regularly once a system is integrated into operations. Moreover, impact assessments differ from risk assessments in that they go one step further, identifying and measuring the array of real-world impacts that a given AI risk could inspire on a specific group, organization, critical infrastructure, society, and even democracy at large.
- Recall or remediation protocols: When an operational AI system is determined to pose an unacceptable risk or be non-compliant, inspire harmful yet unexpected impacts, undergo significant changes or updates, or display emergent properties, protocols for system recall and/or remediation must be established and implemented—often, such protocols will involve the administration of another impact and/or risk assessment. Nonetheless, these protocols should not introduce unnecessary operational bottlenecks and facilitate quick and easy recall or remediation of an AI system when it’s required.
- Reporting protocols: Whether for compliance purposes or internal business requirements, reporting protocols that allow key personnel to report AI risks and impacts immediately and to the right audience are vital. Clear reporting channels between key personnel and stakeholders should be created.
- Bias audits: In cases where AI systems are leveraged for consequential decision-making, or where algorithmic bias is identified as a preventable risk, bias audits should be administered. Audits should be conducted by an independent evaluator on a regular basis and center on the role that historical data plays in algorithmic decision-making.
- Data anonymization and encryption: To ensure data privacy, security, and integrity, organizations must devise and implement data anonymization and encryption methodologies. These methods are typically most important during the AI training or fine-tuning phases, however, if an organization (or its personnel) intends to leverage a commercially available AI model to gain insights from proprietary datasets, caution must be exercised.
- Continuous monitoring: AI systems should be continuously monitored by a human-in-the-loop, to ensure effective human oversight and RAI best practices. Importantly, procedures for continuous monitoring should clearly describe how to identify potential causes of concern so that alarm bells are triggered appropriately.
- Expert-in-the-loop: When an AI system is leveraged to generate content or orchestrate consequential decisions, an expert-in-the-loop must validate its outputs for truthfulness and accuracy. Where AI-generated content could infringe upon IP rights, an expert-in-the-loop is required.
- AI training and awareness: Currently, no standardized AI training and awareness procedures exist. However, mechanisms such as AI seminars, GenAI skills procurement initiatives, AI upskilling and reskilling workshops, RAI consultations, and various different kinds of privately offered AI toolkits are a great place to start.
- AI governance feedback: AI governance frameworks can’t be static, otherwise an organization risks developing, deploying, or integrating AI in ways that are inadvertently harmful to it or its customer base. Establishing and implementing employee, consumer, policymaker, and AI expert feedback channels can be a highly effective way to ensure that AI governance frameworks are always up-to-date.
- Access controls: Once AI-centric roles and responsibilities for key personnel are defined, access controls should also be implemented to ensure that only authorized personnel can leverage or manipulate an AI system. In addition to protecting AI assets, access controls are also crucial to preserving data and network security as well as the larger digital infrastructure supporting an organization.
- Fail-safes: Most organizations might not be here yet, but in cases where autonomous high-impact AI systems are leveraged for some purpose under minimal human oversight, like automated trading or smart manufacturing, fail-safes must be created whereby a system can be manually overridden or shut off before it does too much damage. These fail-safes should also be applied to high-impact AI systems, that if hijacked, could be used to enact widespread real-world harmful impacts.
- AI lifecycle documentation: Documentation on AI risk, impact assessments, and bias audit results, AI system’s intended purpose, design, and function, risk management, reporting, and continuous monitoring protocols, data security and privacy techniques, cybersecurity procedures, and key roles, responsibilities, and access controls should be regularly maintained. This kind of documentation is instrumentally valuable to maintaining transparency, trustworthiness, safety, and accountability standards and streamlining compliance with business and regulatory requirements.
- Notice and explanation: Any employees or consumers affected by an AI system should be made aware of their interaction with it before using it, the impacts it could generate on them, and procedures for requesting further information on the system, particularly as it concerns the role it may play in consequential decision-making contexts or the data that was leveraged for processing or training purposes.
Conclusion
The AI governance properties, principles, and mechanisms we’ve covered in this post offer a strong starting point, foundation, or growth direction for any organization developing, implementing, or updating its AI governance framework. This isn’t to say that we’ve exhaustively covered everything that could possibly be relevant to an effective AI governance strategy—as we’ve stressed before, AI governance approaches must be tailored to suit an organization’s context, needs, objectives, resources, and likely several other more nuanced factors.
That being said, this is the first piece in our “Perspectives on AI Governance” series. Our future discussions will consider a variety of different angles, each of which will hopefully shed more light on this topic such that readers can eventually craft their own holistic understanding of it. In fact, our next piece will take a more critical yet high-level perspective, examining the “do’s and don’ts” of AI governance in detail.
Nonetheless, we invite readers to follow Lumenova AI’s blog, where they can glean additional insights on the AI governance and regulation landscape alongside topics like AI risk management, RAI, and GenAI.
For readers who are eager to initiate concrete AI governance and risk management protocols, consider trying out Lumenova AI’s RAI platform, and book a product demo today.
Perspectives on AI Governance Series
Perspectives on AI Governance: AI Governance Frameworks