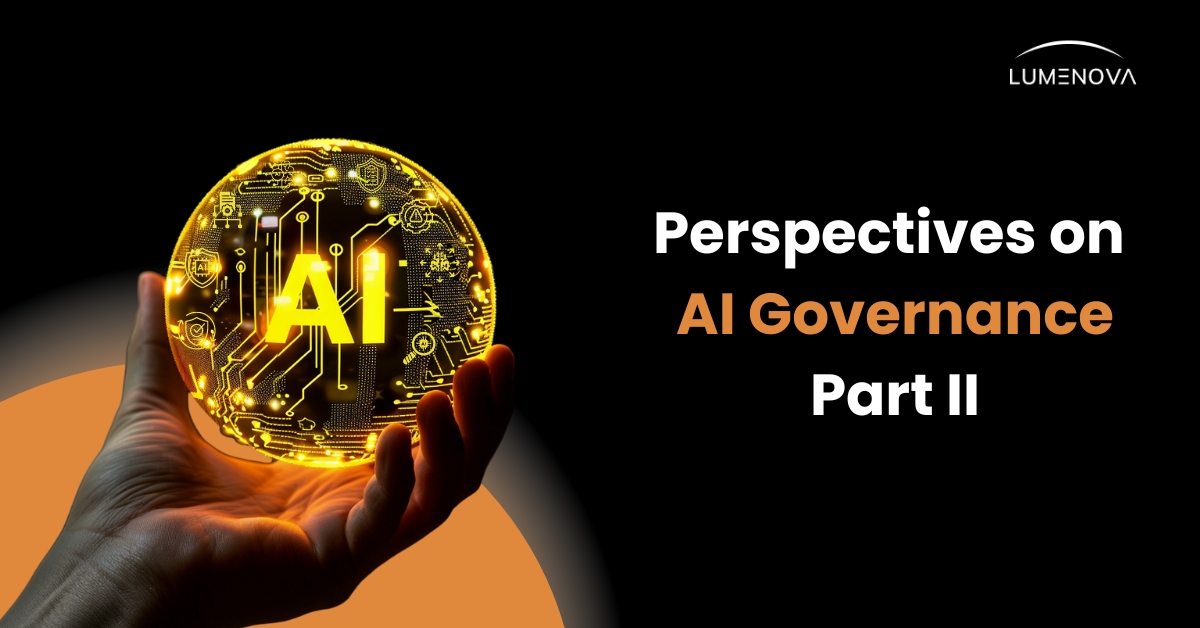
Contents
In our first post in this series, we examined the fundamental properties, core principles, and mechanisms integral to any AI governance framework. What we didn’t mention, however, was that even if a framework happens to possess most of these characteristics, we still can’t guarantee that it will be successful.
AI governance itself is a nascent concept that has yet to be holistically tried, tested, validated, and explored—even the most resilient and intelligently designed frameworks and approaches will not always produce reliable results. In other words, the pace at which AI innovates and proliferates makes it extremely difficult to design and implement AI governance protocols that are both robust and resilient in the face of AI-inspired changes and impacts.
Still, this doesn’t mean that we shouldn’t take AI governance seriously—quite the opposite, such frameworks are essential to identifying and managing AI risks, enacting AI benefits, anticipating and mitigating potential AI impacts, preserving fundamental rights, democratic rule of law, and business operations, and accommodating as well as adapting to AI-driven changes as they alter the socio-economic and socio-political landscape of our world. In simple terms, AI governance is an important tactic through which we can significantly increase the probability that AI works for us rather than against us.
Consequently, this post will adopt a more future-oriented normative perspective, whereby we’ll explore what an AI governance framework should and shouldn’t do (assuming that it already possesses all of the characteristics described in our previous post). To make this more concrete, consider the following analogy:
Take a minivan, sports car, or truck on the race track and the sportscar will win every time. Take them off-roading, and the truck will leave the other two in the dust. And when it comes to taking your kids to school, no one can beat the minivan.
Each car is designed with a specific purpose in mind, making it suited to a particular environment. We want the sportscar to be fast, so we lower its center of gravity, widen its wheelbase, and increase its power-to-weight ratio by reducing its size and installing a more powerful engine. But now, the sportscar can’t offroad and is a poor choice for daily commutes. So, we have to take a step back and ask ourselves, is the sports car what we really want? Or, do we want the minivan or truck instead, or if we can afford it, all three?
This analogy highlights the following point: AI governance frameworks should be purpose-built—this isn’t to say they can’t have more than one purpose—which implies that they should and shouldn’t do certain things. And, it’s up to us to figure out precisely what these things are.
In the next sections, we’ll begin by discussing what an AI governance framework shouldn’t do, followed by what it should do, and conclude with some strategies that individuals, organizations, and policymakers alike can utilize to hone in on the purpose (i.e., scope) of their AI governance framework. Before we dive in, however, we should note that the “do’s and don’ts” we’ll identify operate at a high level—AI governance initiatives must possess a targeted scope, and in some cases, they will need to pay close attention to all the “do’s and don’ts” we mention, but in others, only a few will be relevant. Remember, do you want the sports car, truck, minivan, or all three?
For readers who want to explore more content on AI governance, policy, and risk management, we recommend that you follow Lumenova AI’s blog, where you can also begin examining concepts in the generative (GenAI) and responsible AI (RAI) spaces.
AI Governance: Don’ts
At an abstract level, every AI governance initiative strives to do the same thing: ensure that AI efforts are conducted responsibly and efficiently such that AI benefits are enacted and AI impacts don’t result in any unintended harm or consequences for interested parties. Therefore, the “don’ts” we discuss below would, if motivating, underlying, or substantiating an AI governance strategy, directly undermine and/or impede its ability to operate effectively.
- Unnecessary bureaucracy: Finding the right bureaucratic balance is tricky. An AI governance framework should be strict enough to ensure that AI risks, benefits, and impacts are adequately managed at all relevant stages of the AI lifecycle, but not so strict that every single AI-inspired change needs to be vetted and approved by multiple different parties at various ranks throughout an organization. Put differently, the level of bureaucracy an AI governance strategy supports should be roughly proportional to the degree of change management you expect you’ll need to deal with. For example, if an organization decides only to use AI narrowly, its impacts will be confined to a small space, and change management, though important, won’t necessarily be critical. By contrast, if an organization integrates AI at scale, for numerous different tasks, teams, and individuals, its impacts will reverberate throughout the organization, necessitating a much higher degree of change management acumen and speed, which excessive bureaucracy could unnecessarily compromise.
- Stifling innovation: The AI tide is quickly rising, and there’s nothing we can do to stop it. However, we can guide it in the right direction or slow it down if we need to. Simply put, an AI governance strategy needs to strike a delicate balance between enacting AI’s transformative potential and mediating its disruptive impacts. Following in the pro-innovation footsteps of the EU AI Act, UK Proposal for AI Regulation, and White House Executive Order on Safe, Secure, and Trustworthy AI, organizations would be wise to place a stronger and more stringent emphasis on governing AI deployment and integration processes as opposed to design and development procedures. This isn’t to say that governing the early stages of the AI lifecycle is unimportant, but rather, that many of the most salient risks and impacts arise during its later stages.
- Stifling experimentation: AI is an extremely versatile technology that can provide value across many domains. With purpose-built AI applications (i.e., narrow AI systems) intended use cases are typically pre-identified. However, this doesn’t automatically indicate that such a system couldn’t be fine-tuned or re-trained to provide value elsewhere, and things get even more complicated when we consider general-purpose AI systems that can accomplish a variety of tasks across disparate domains. In this respect, it’s crucial that an AI governance framework enables some degree of experimentation, not only so that people can learn how to leverage AI systems responsibly and effectively, but also so that organizations can identify novel areas in which AI is likely to produce substantial value, impacts, or risks.
- Check-the-box mindset: If an organization adopts a check-the-box mindset on AI governance, it’s basically guaranteeing that its governance approach will fail. AI moves so fast that AI governance strategies will require regular updates, revisions, and improvements to account for novel AI impacts, risks, and use case scenarios, and organizations should resist the temptation to wait to implement AI governance frameworks until AI regulation catches up. Moreover, AI governance is a deeply complex issue, and if organizations don’t evaluate their strategy critically, thoughtfully, and regularly, they’ll soon find themselves in a position where they’re forced to play catch-up with an outdated framework, which could result in losing their competitive advantage or even incurring substantial legal and reputational costs.
- Lack of feedback and remediation mechanisms: To build on the point we made above, the pace at which AI innovates and proliferates necessitates that AI governance frameworks be updated and revised accordingly. But, these updates and revisions need to come from somewhere, most likely the teams and individuals leveraging AI within an organization and/or consumers/users, and possibly, external RAI, governance, and risk management consultancies. An AI governance strategy that doesn’t integrate clear mechanisms and procedures for stakeholder feedback and/or consultation risks implementing revisions and updates that are impractical or unfeasible, failing to align with an organization’s real-world AI use cases, risks, and impacts.
- One-size-fits-all approach: Recall the earlier point we made about AI’s value being rooted in its versatility. This is an obvious fact, and certain organizations may respond to it by trying to establish and implement a one-size-fits-all governance strategy. A comprehensive and ubiquitous AI governance model may look nice on the surface, but if it doesn’t take a targeted approach, whereby the specific AI risks, impacts, and use cases encountered by an organization aren’t clearly defined and accounted for, governance could prove to be more hurtful than beneficial in the long run. Specifically, it could lead to organizations suffering several types of negative consequences from reputational damages and compliance struggles to novel workflow bottlenecks and pain points.
- Compliance is the only thing that matters: AI regulations are quickly emerging, especially for AI systems classified as high-risk or high-impact. Compliance costs will likely be severe in many cases—under the EU AI Act, compliance penalties can range as high as 35 million euros—and this could push organizations to adopt a compliance-centric mindset in designing and implementing their AI governance strategies. However, as we mentioned before, AI regulations will always lag behind the current state of AI, meaning that they will rarely reflect an up-to-date understanding of all foreseeable and preventable AI risks and impacts. Organizations that adopt AI governance protocols exclusively for compliance purposes will always be responding to the latest AI regulations rather than proactively preparing themselves for them.
- Lack of adherence to industry best practices, standards, and guidelines: More recently, industry standards and guidelines like the NIST AI Risk Management Framework, California’s GenAI procurement guidelines, OECD AI Principles, ISO 42001, and ASEAN Guide for AI Governance, have emerged. All of these resources are beginning to lay the groundwork for a globally shared AI governance language, and many of them will soon become mandatory components of AI regulation. Organizations won’t have to adhere to each of these standards and guidelines in all cases, and will also likely have to fine-tune them to suit their particular objectives, needs, and resources. However, failure to adhere to these best practices will introduce easily avoidable complexities and hurdles to an AI governance strategy, and more importantly, compromise an organization’s ability to develop a consistent, interoperable, and agreed-upon governance language and terminology.
- Lack of AI talent or expertise: Organizations should build their AI governance strategies in consultation with AI governance experts, policymakers, RAI practitioners, industry specialists, and AI safety researchers. Attempting to design and implement an AI governance model in the absence of targeted AI talent and expertise is likely to result in a model that fails to grasp the nuances of AI deployment and integration, risk management, education, awareness, and upskilling, impact assessment, compliance reporting, and much more. Fortunately, the AI talent pool is steadily expanding, and soon organizations will have several resource-driven avenues they can draw from.
- Poor communication: Organizations must ensure that their AI governance protocols are clearly communicated to all relevant parties and stakeholders, and in cases where such entities require additional upskilling or education to make sense of governance protocols, services and solutions must be provided. From the C-suite to the general workforce, an organization’s AI governance strategy must be aligned, to reduce the risk that AI is used irresponsibly or in a way that severely compromises business operations and performance.
AI Governance: Do’s
Extrapolating from all the “don’ts” we’ve just highlighted, we’ll now investigate several AI governance “do’s” for organizations to consider when developing and implementing their AI governance strategy:
- Think proactively not reactively: AI risks can be divided into four categories: 1) known-knowns, 2) known-unknowns, 3) unknown-knowns, and 4) unknown-unknowns. Category 1 concerns risks whose probability and saliency are clearly and unambiguously understood. Category 2 concerns risks we understand but don’t know the probability of. Category 3 concerns risks that we should know given the available evidence, but don’t actually know about—these kinds of risks are sometimes referred to as “untapped knowledge” and are frequently a result of negligence. Category 4 refers to risks we are wholly unaware of. All of these risk profiles are highly important, but it’s the latter two categories that are crucial for an organization to understand in the context of AI governance. Simply put, to think proactively and ensure that their AI governance framework enables a holistic understanding of AI risks, organizations need to pay especially close attention to unknown-known and unknown-unknown AI risks.
- Maintain an open mindset: Nailing down the right AI governance strategy will not happen overnight—an AI governance framework will have to undergo multiple iterations before it’s deemed sufficient. Future iterations, however, will, in large part, be informed by current AI governance shortcomings and failures. This means that organizations with a rigid mindset, who attribute problems in their AI governance approach not to the approach itself, but to other external factors like AI-driven changes or a shift in organizational culture, will spend time and resources “improving” an AI governance strategy only to find that it’s still flawed. Therefore, organizations would be wise to assume that things will get messy, and this mindset will allow those who adopt it to hold themselves accountable for AI governance failures while addressing them pragmatically and efficiently.
- Identify AI knowledge gaps: Once you figure out the scope of your AI governance framework—what it seeks to address and who will be subject to it—you need to identify potential AI knowledge gaps, particularly among company leadership. These knowledge gaps should be identified and closed before an AI governance strategy is established and implemented. Otherwise, even the most brilliant approach might be orchestrated in vain.
- Understand the AI risks your organization faces: AI safety researchers have identified an exhaustive variety of AI risks across numerous industries and domains, going so far as to isolate many of them by reference to specific use cases, impacts, and AI applications. Despite the incredible amount of work that’s gone into informing the AI risks repertoire, most AI risks will still undergo nuanced changes to their profile in a specific organizational context. For example, AI safety researchers and regulators are beginning to crack down on the use of AI for consequential decision-making in hiring contexts. But, one doesn’t just use AI for hiring—identifying which specific components of the hiring process you want to leverage AI for is critical. Leveraging AI for applicant screening procedures vs. candidate selection or assessment vs. interview evaluation will determine the boundaries and nuances of specific AI risks and their impacts.
- Assess your AI readiness levels: If an organization is seriously considering AI governance, chances are they’ve already integrated or plan to integrate AI—ideally, an AI governance strategy should be fleshed out before or in tandem with AI integration efforts. Nonetheless, the AI governance strategy that an organization settles on will be predicated upon and driven by the intended AI use cases the organization selects. If the organization in question doesn’t have a technological infrastructure that would support AI integration and/or sufficient AI expertise to enable organized and localized integration efforts, the AI governance strategy it establishes could prove to be disjointed, insufficiently targeted, and ultimately ineffective. When organizations are in the early stages of AI governance, they should carefully assess their AI readiness levels, to ensure that initial or ongoing AI integration efforts align with their real-world capacity to support a robust AI governance framework.
- Establish continuous learning as a central tenet of organizational culture: Continuous learning is an important cultural tenet in any organization independent of whether it plans to leverage AI or not. That being said, even though AI isn’t a new technology, it has reached a point where, today, it’s more accessible to the average person than it ever has been. This means that more people will have more opportunities to experiment with the technology and learn from it, discovering new and potentially lucrative sources of value. If organizations wish to capitalize on this value and ensure that any related impacts are managed responsibly, they should strive to develop AI governance strategies that emphasize continuous learning at an organization-wide scale. Still, AI governance protocols should set clear parameters around what constitutes responsible vs. irresponsible continuous learning practices, and establish resource-driven channels and “safe” experimentation environments in which employees can test and evaluate their AI skills.
- Evaluate alternative AI governance approaches: Compliance and competitive AI adoption pressures are pushing organizations to quickly develop and implement AI governance protocols—when it comes to AI governance, time is a salient factor, and this could motivate many organizations to rush their AI governance approach without appropriate scrutiny. Fortunately, organizations can lower the probability of this risk by evaluating alternatives to their AI governance strategy before implementing it. In fact, comparatively analyzing your organization’s AI governance tactics with others could directly streamline the development of a robust AI governance strategy, since it allows you to identify governance solutions and pitfalls without having to spend tons of time thinking about them.
- Align AI governance with business objectives, values, and culture: An AI governance strategy should easily map onto business objectives, values, and culture, to ensure the seamless integration of AI governance protocols where necessary. Moreover, when AI governance strategies are well-aligned, it becomes much easier to modify and update them in accordance with potential changes to business operations and overall mission.
- Be transparent and encourage feedback: AI governance feedback and remediation channels are profoundly useful governance tools, but only if people participate in them. In a business setting, it’s not uncommon for employees to resist providing feedback for fear that some retaliatory action will be taken in response (even if there are no grounds for it). Consequently, organizations should establish transparent reward-based incentive structures through which to motivate teams and employees to provide feedback as they see fit. Organizations should also communicate, in a clear and unambiguous fashion, that AI governance feedback falls within the realm of employee responsibility, and that retaliatory measures will never be taken in these cases.
Honing In On the Scope of Your AI Governance Framework
Now that we’ve disseminated the “do’s and don’ts” of AI governance, we’ll transition to a brief overview of what tactics and methods organizations can leverage to enact the “do’s” and avoid the “don’ts”. These approaches are fundamentally pragmatic, and while some of them may require more work than others, each one is suggested with real-world feasibility in mind. Moreover, while this isn’t strictly the case, we suggest that organizations implement these approaches before they’ve finalized their AI governance strategy, though many of them may also prove useful in cases where an AI governance strategy must be updated or revised. The tactics and methods we suggest are described below:
- Identify desirable AI skills that correspond with your organization’s AI objectives. This will also allow you to identify and isolate the key teams and personnel involved in or driving AI initiatives.
- Administer AI skills assessments to key teams and personnel to understand whether they possess the desirable AI skills you’ve identified. This will enable a quicker and more targeted evaluation of AI knowledge gaps, which will also streamline any necessary upskilling and reskilling procedures.
- Crowdsource AI knowledge internally to figure out what employees are using AI for and how. This will make identifying potential AI use cases easier while also fostering a more nuanced and context-specific understanding of the ideal parameters surrounding responsible AI use.
- Establish collaborative channels or partnerships with AI safety specialists, RAI practitioners, and policymakers to ensure that your AI governance framework maintains its relevance as AI-driven changes continue to alter the business landscape. These kinds of collaborative channels and partnerships will also increase your organization’s trustworthiness, which could result in a number of benefits.
- Build and run RAI awareness campaigns that establish a tangible link between RAI use and real-world benefits. Explaining to employees why RAI use is good for them, not just the business as a whole, will intrinsically motivate them to leverage AI responsibly.
- Encourage teamwork on AI projects to reduce the probability of AI silos and leverage diverse expertise to derive novel and innovative AI solutions. Teams also require oversight, and the more teams—as opposed to individuals—organizations have working on AI projects, the easier it will be to ensure that AI initiatives align with AI governance protocols.
- Identify what you don’t want your AI governance strategy to do. In addition to the “don’ts” we’ve mentioned, organizations may find that they have supplementary AI governance constraints. Moreover, in cases where an organization has limited resources and/or is unsure where to begin with AI governance, reverse-engineering an AI governance framework by reference to “don’ts” and context-specific constraints could prove effective during the early governance stages.
- Create an AI governance team whose sole purpose is to maintain, oversee, and update your organization’s AI governance strategy. This team should report directly to leadership, maintain robust relationships with key teams and personnel involved in AI initiatives, and possess near-full autonomy over your organization’s AI governance strategy. The team should be small—to avoid unnecessary bureaucracy—and be composed of verified experts from the AI policy, safety, and RAI spaces.
- Be careful with the AI governance “experts” you select. AI has been around for decades, but AI governance has only become a central feature of the AI landscape over the last few years. To put it bluntly, the AI governance landscape is saturated with self-proclaimed “experts” who are now aware of this recent but highly profitable value niche—don’t settle on the first “expert” that comes your way, and be sure to vet each one’s skills, background, and services appropriately.
- Push yourself to consistently take a step back and see the big picture. AI governance strategies need to be targeted, but this increases the risk of getting caught in the details and wasting valuable time and resources on narrow AI governance objectives that might, in reality, not be that important.
- Prioritize your AI governance objectives to ensure that your allocation of time and resources goes toward the most important objectives you’ve identified. Prioritization will also help streamline objective completion by enabling more targeted and localized efforts.
Conclusion
In this piece, we’ve disseminated the “do’s and don’ts” of AI governance along with some simple pragmatic recommendations organizations can follow to increase the likelihood that their AI governance strategy is both appropriate—with respect to their needs, objectives, and values—and effective—in terms of compliance, risk management, and RAI use. Consequently, and as an exercise, we challenge readers to ask themselves the following questions while reflecting on this material:
- What do you hope to achieve with AI governance?
- How will you design, establish, and support your AI governance strategy?
- Why is AI governance important to you?
- What aspects of AI governance worry or confuse you, and why?
- Who will be responsible for AI governance within your organization?
- If necessary, how will you identify and acquire AI governance support?
- How will you communicate and implement your AI governance strategy?
- Is your organization ready for AI governance?
AI governance is a genuinely complex issue that’s poised to evolve significantly over the coming years. We strongly encourage organizations to begin seriously exploring and implementing AI governance strategies, or at the very least, frameworks, now. You don’t want to be in a position where you have to play catch-up, especially when considering how fast AI moves. Also, just think how absurd it would be to not be able to capitalize on AI-inspired benefits simply because your organization doesn’t have a thoughtful and thorough understanding of AI governance.
Nonetheless, our next piece on this topic will be more philosophically driven, delving into the relationship and intersection between AI ethics and governance. It’s also worth noting that for those operating in the RAI space, our next piece may be of particular interest.
Still, for readers craving more information on the AI governance, policy, and risk management landscape, we invite you to follow Lumenova AI’s blog, where as we mentioned earlier, you’ll also find in-depth content on RAI and GenAI topics.
For readers who want to begin developing their AI governance and risk management strategies now, we recommend that you take a look at Lumenova’s RAI platform and book a product demo today.
Perspectives on AI Governance Series
Perspectives on AI Governance: AI Governance Frameworks