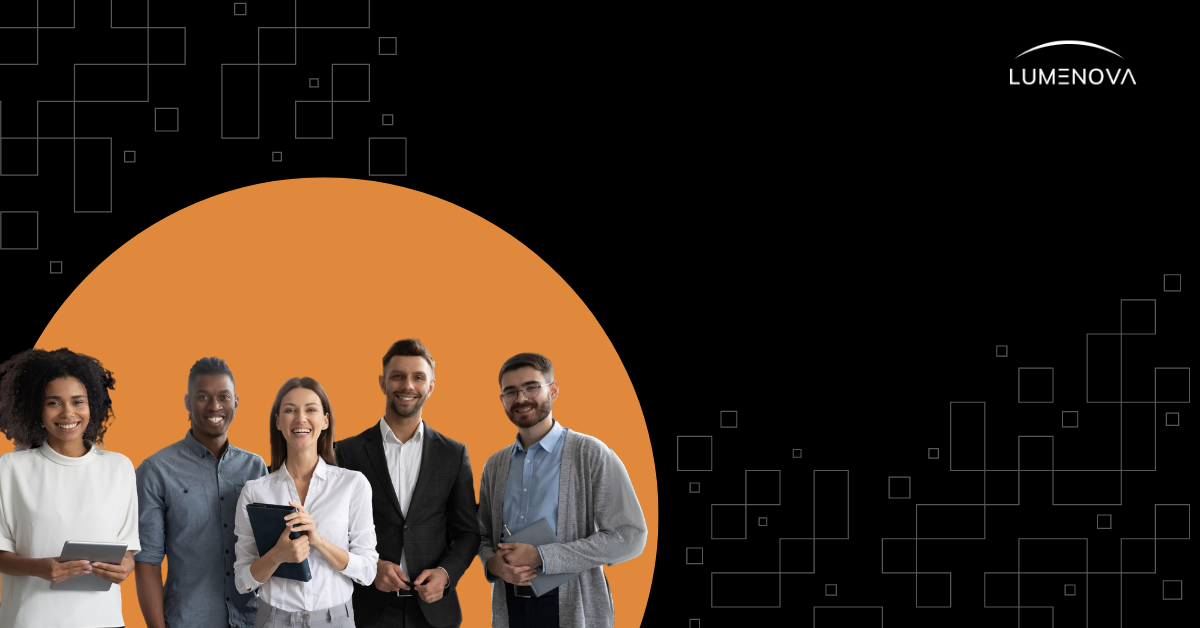
Contents
To shape AI systems that are both responsible and effective stakeholder engagement is crucial. This is because deploying safe AI means going past the technical intricacies of Machine Learning, and delving into identifying and addressing risks that are related to AI bias, fairness, and the broader social implications of AI technologies.
After all, AI systems can misstep in numerous ways, raising questions about responsibility and accountability.
It’s only by actively involving a diverse group of stakeholders throughout the entire AI lifecycle, that organizations can gain valuable insights to help them tackle challenges like AI bias, discriminatory outcomes, and societal impacts head-on.
Transparent stakeholder collaboration is the backbone of accountable and responsible AI, enabling AI strategies to be aligned with ethical guidelines and regulatory standards, and ultimately contributing to the creation of AI solutions that are both responsible and human-centered.
Because, the ultimate goal is not only to create and deploy state-of-the-art AI models, but to also ensure they are fair and less prone to risks. AI accountability is essential because it directly influences customer trust, brand reputation, legal responsibility, and ethical standards.
Who is Responsible for AI: Addressing AI Accountability
Ensuring the responsible deployment and management of AI systems requires the involvement of a broad range of stakeholders. But who exactly is accountable for AI models?
From system engineers, project and product managers, designers, developers, data scientists and AI/ML experts, to regulators, auditors, and users of AI technologies, all of these stakeholders play crucial roles in holding AI systems accountable.
In their paper, researchers Alun Preece and Dan Harborne define the following stakeholder communities, from the Explainable AI perspective, highlighting the important roles they play in AI accountability.
Developers
Stakeholder overview:
Primarily focused on building AI applications, this group usually includes professionals from large corporations, small and medium enterprises, the public sector, and academia.
Key focus points:
Developers are deeply invested in the quality and reliability of AI models, often using the terms “explainability” and “interpretability” to describe their efforts. Their main goal is to ensure robust system testing, debugging, and evaluation to improve application performance. Developers frequently leverage open-source libraries for generating explanations, with popular tools including LIME, deep Taylor decomposition, influence functions, and Shapley Additive Explanations.
Theorists
Stakeholder overview:
Theorists are important actors, accountable for advancing AI theory, particularly in the area of deep neural networks. These individuals are typically found in academic or industrial research settings.
Key focus points:
Unlike developers, theorists are more focused on pushing the boundaries of AI knowledge rather than on practical applications. They tend to use the term “interpretability” more than “explainability,” reflecting their interest in the fundamental properties of AI models. Some interpretability research in this community has even been categorized as “artificial neuroscience,” highlighting their theoretical focus. ****Members of the theorist community are considered to be system creators. For example, theorists can perform research work on deep neural network technology, without actually building the system.
Ethicists
Stakeholder overview:
Ethicists are concerned with the fairness, accountability, and transparency of AI systems. This group includes policymakers, commentators, and critics from a wide range of disciplines, such as social science, law, journalism, economics, and politics. While many ethicists are also computer scientists or engineers, they bring an interdisciplinary approach to AI ethics.
Key focus points:
For ethicists, explanations need to extend beyond technical quality to encompass fairness, unbiased behavior, and transparency. These explanations are crucial for ensuring AI accountability, auditability, and legal compliance, particularly in light of regulations like the EU’s GDPR and the EU AI Act.
AI Users
Stakeholder overview:
The user community encompasses anyone who interacts with or is affected by AI systems.
Key focus points:
Unlike the previous groups, users generally do not contribute to the academic literature on AI explainability or interpretability. However, they require clear explanations to make informed decisions based on AI outputs and to justify their actions. This community includes both direct end-users and those involved in processes influenced by AI, such as a company director or clients in an insurance firm that relies on AI for policy decisions.
The Role of Responsible AI Team Collaboration and Its Influence on AI Accountability
The development,deployment and oversight of responsible and accountable AI systems hinge on the collective efforts of a diverse range of stakeholders, each bringing their unique expertise and perspective to the table.
When developers, theorists, ethicists, and users work together, they form a comprehensive ecosystem that addresses both the technical and ethical challenges of AI. After all, advancing accountable AI is a collective responsibility, not the task of a single individual.
Developers are accountable for ensuring that AI systems are robust and reliable, while theorists push the boundaries of AI capabilities. Ethicists provide the necessary checks on fairness and transparency, ensuring that AI systems are held accountable and aligned with societal values and legal standards. Meanwhile, users offer practical insights into how AI impacts real-world decisions and behaviors.
How Companies are Tackling the Challenge of AI Accountability
While technology companies often prioritize the impact of responsible AI systems on users, it’s crucial to recognize the equal importance of other responsible AI stakeholders like advocacy groups, policymakers, and community organizations.
As described by Advait Deshpande and Helen Sharp, these groups can play a significant role through soft power interventions—such as crafting manifestos or engaging in online activism—to elevate user awareness and drive AI accountability.
To effectively manage the impacts of responsible AI systems, technology companies must also focus on internal organizational changes.
Here are some key considerations:
- Establishing dedicated responsible AI teams and advisory boards to oversee and document AI-related decision-making. This might lead to adjustments in hiring practices, workforce composition, and required skill sets, as seen with companies like Microsoft, Google, and SAP, which have adapted their hiring strategies to better align with responsible AI principles. Additionally, it’s also essential to train employees on AI and responsible AI practices to ensure they are equipped to make informed, ethical decisions in the deployment and use of AI technologies.
- Allocating resources toward data collection, system testing, and research into the social impacts of their AI systems, as well as, when necessary, technical research to ensure their AI solutions are both effective and ethical.
- Adapting to the evolving legal and regulatory obligations for responsible and accountable AI. As legal and regulatory frameworks for responsible AI continue to evolve at both national and international levels, organizations may also need to rethink their corporate structures to manage liability risks effectively and ensure compliance. The legal obligations are expected to focus on issues such as data blind spots, biases in data collection, and the impact of these biases on AI decision-making processes.
- Revising practices regarding how AI systems collect and use user data, including behavioral data, in order to address the power imbalance between AI systems and their users. It’s essential for companies to not only implement but also transparently communicate the mechanisms through which they inform users about the legal responsibilities tied to responsible AI systems. This approach will help ensure that users are well-informed and that organizations maintain trust and accountability in their AI operations.
For instance, Facebook has implemented a ‘red team’ approach to scrutinize and enhance the security and ethics of its AI systems. Similarly, major technology companies like Microsoft, Nvidia, IBM, and Google have either publicly released or developed internal frameworks and guidelines aimed at addressing research concerns and responsible AI product development. On the non-tech side, H&M Group stands out in the literature for its development of a checklist designed to ensure the responsible use of AI systems within its operations.
The Evolving Role of Stakeholders in Ensuring AI Accountability
While AI can drive significant momentum for businesses, the initial stakeholder input is crucial in determining whether the system’s impact will be positive or negative.
Responsible AI leaders face a pressing challenge: integrating stakeholder inclusion and oversight into AI systems and processes effectively. Current models of stakeholder engagement may wield considerable influence, but this leverage might not be as strong in the future.
Managing AI stakeholders is a delicate balancing act. Leaders must foster trust among employees, investors, partners, and other impacted stakeholders, each with their own sometimes conflicting interests and significant stakes. As AI and other technologies become increasingly integrated into workflows, traditional methods of building trust must evolve to keep pace with these advancements and to ensure AI accountability.
AI Policy: Key Approaches and Frameworks Emphasizing the Importance of Stakeholder Engagement for Accountable AI
Globally, both governmental bodies and respected research institutions recognize the essential role of stakeholder engagement throughout the AI system lifecycle. Below are some notable examples of how stakeholder involvement is embedded in various AI policy frameworks.
NIST’s AI Risk Management Framework (2023): The framework’s “Govern 5” function, along with its sub-functions, highlights the need for strong stakeholder engagement. Additionally, the “Map 5” function emphasizes assessing the impacts on individuals, groups, communities, and society through active involvement with stakeholders. Learn more about NIST’s AI Risk Management Framework.
The EU AI Act (2023): Article 15(2) of the EU AI Act underlines the importance of stakeholder engagement in addressing technical elements, such as measuring the accuracy and robustness of AI systems. Article 40 further advocates for multi-stakeholder governance to ensure balanced representation and meaningful participation. Learn more about the EU AI Act.
ISO/IEC 42001 (2023): This standard establishes concrete benchmarks for AI risk management, emphasizing the role of leadership in ensuring that AI systems align with organizational objectives, compliance, and responsible AI (RAI) practices. It requires organizations to assign roles for monitoring AI performance and ensuring compliance. Leaders must also provide resources and foster a culture of awareness, training, and continual improvement for personnel involved in AI development and integration. Learn more about ISO/IEC 42001.
Final Thoughts
Navigating stakeholder engagement in the dynamic world of AI systems can feel like walking a tightrope over shifting ground.
As AI capabilities expand, an increasing number of individuals will be affected by leadership decisions and will seek to have their voices heard. As such, moving towards AI accountability is essential. At the same time, the fast-paced nature of technological change may pressure leaders to make decisions swiftly and address concerns afterward.
To maintain meaningful and effective stakeholder engagement, organizations must proactively address these challenges and ensure their engagement strategies are both robust and adaptable. Equally important is integrating AI accountability into these strategies, ensuring that AI systems are used responsibly and transparently while considering the diverse interests and concerns of all stakeholders.
If you’re curious to dive deeper into AI risk management, governance, or other topics like generative AI and responsible AI (RAI), be sure to follow Lumenova AI’s blog. It’s a great place to stay updated on the latest insights and trends in these areas.
And for those actively working on AI governance and risk management—whether it’s through setting internal policies, creating protocols, or developing benchmarks—we encourage you to explore Lumenova AI’s Responsible AI platform. You can also book a demo to see how it all works.