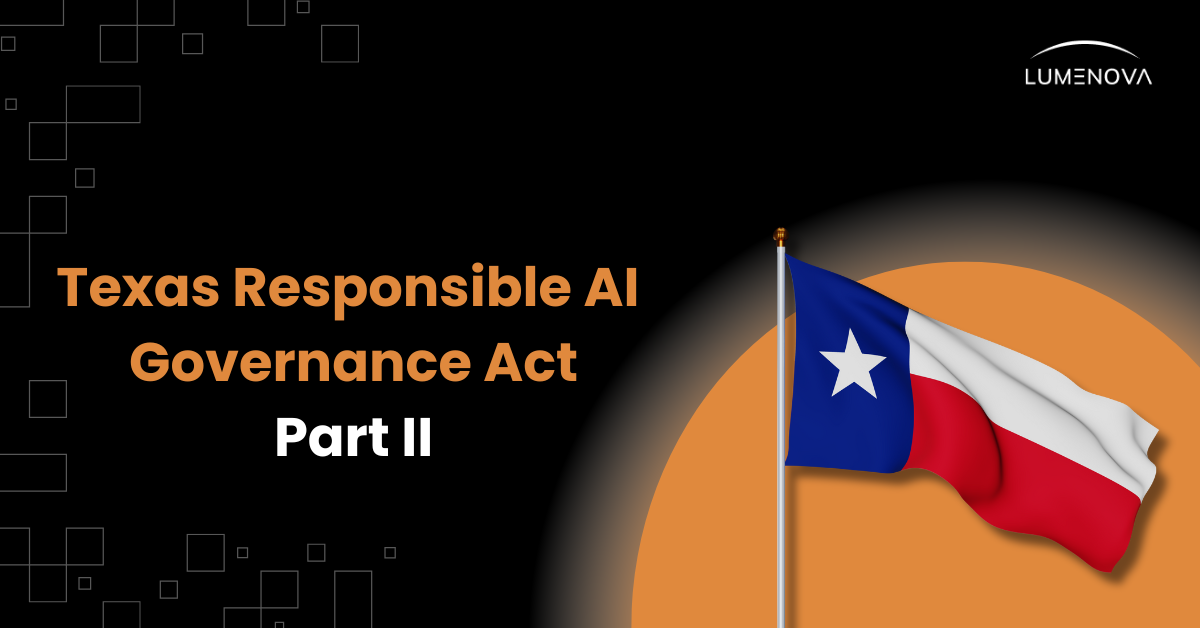
Contents
In part I of this series on the Texas Responsible AI Governance Act (TRAIGA), we conducted a detailed review and breakdown of the act’s core provisions. Here, we’ll examine TRAIGA from a critical perspective, identifying its key strengths and weaknesses, anticipating its impacts, and providing suggestions regarding potential future avenues for improvement.
However, before we get started, it’s important to understand why TRAIGA is positioned as legislation that, if enacted, could deeply influence the form, function, and scope of the US federal AI governance agenda. The reasons we’ll shortly examine are by no means exhaustive, but they do showcase, at a high level, why we would be wise to conceptualize TRAIGA’s potential approval and enactment as a nationally significant event.
First, the act has already inspired major controversy and harsh criticism from notable members of the AI community. While the sentiments behind these criticisms are valuable in their own right, what matters most in this context is quite literally the controversy itself. If the AI community didn’t have sufficient reason to believe that TRAIGA would materially and substantially affect the course of current and future AI innovation outside of Texas, the incentive to publicly support or oppose it would dissolve.
Second, it’s not uncommon for state-level regulations to set federal precedents, and even more so, cement the foundation for federal regulations. For instance, Massachusetts’ 2006 healthcare reform initiative—known as “Romneycare”—became the blueprint for Obama’s Affordable Care Act. Similarly, the California Consumer Privacy Act (CCPA), passed in 2018, clearly serves as the main source of inspiration behind the American Data Privacy and Protection Act. It’s also worth noting that the CCPA was modeled on the EU’s GDPR, which further highlights how seemingly localized regulations can generate large-scale regulatory impacts.
Third, while California is the US’ modern-day epicenter for technological innovation, other states are beginning to attract the attention of high-profile tech innovators, with Texas standing out as an early contender. In other words, there is a clear implication at play here: if Texas, given its newfound status as a blossoming tech innovation hub, develops a comprehensive technology-centric regulation, we should take it seriously. To this point, President Trump’s Project Stargate, a newly announced $500 billion private investment initiative in national AI infrastructure, will commence with the construction of a data center in Texas.
Fourth, two key components of TRAIGA—the establishment of the Texas AI Council and the vague definition of high-risk AI systems—if applied at the scale of the federal government, could dramatically expand the scope of control federal regulators have over how AI systems are developed and deployed nationally. This isn’t to say that our government implicitly craves tight control over innovative technologies—in fact, historically loose tech regulation appears to directly contradict this point. However, we must entertain the possibility that AI’s potential impacts on critical domains like national security, military operations, and public health and infrastructure, will inspire a regulatory incentive structure that favors much tighter controls at the national scale.
Now that we’ve broadly explained why we expect that TRAIGA’s influence will range beyond Texas, we can dive into the heart of our discussion, taking a deeper look at the act’s key strengths, weaknesses, and related impacts.
Strengths, Weaknesses, and Impacts
When making the following value-based categorizations regarding TRAIGA’s strengths and weaknesses, we are doing so from a predominantly regulatory perspective—that of a governing body responsible for implementing, maintaining, enforcing, and/or modifying and updating compliance requirements. This is an important point to consider seeing as whether something classifies as a strength or weakness often depends on the frame of reference one adopts—this is one of the central reasons for which developing regulation, especially for an exponential technology like AI, is so challenging.
Once we’ve examined TRAIGA’s strong and weak points, we’ll venture into uncertainty, predicting a variety of real-world impacts we expect the act will produce. However, in the interest of fostering a more nuanced and expansive interpretation of these future trajectories, we’ll subdivide impacts into a selection of groups: 1) regulatory, compliance, and legal 2) economic, 3) technological, 4) social and ethical, and 5) long-term. In making these predictions, we will also assume that TRAIGA has been passed and enacted—this is not an endorsement of the act—to minimize constraints on how we think about the future.
Strengths
TRAIGA exhibits a comprehensive regulatory scope whereby concrete standards for ethical AI development and deployment, consumer protections, safe innovation practices, and workforce development are established. In terms of key strengths, TRAIGA:
- Covers a wide range of technical and non-technical AI governance issues throughout key stages of the AI lifecycle, fostering regulatory and AI risk management preparedness in the face of potential AI advancements.
- Supports core responsible AI (RAI) principles shared by standards such as the NIST AI Risk Management Framework, EU AI Act, and OECD AI Principles, implicitly driving alignment with internationally recognized AI governance best practices.
- Mandates, via rigorous risk assessment and impact monitoring, transparency provisions that ultimately aim to bolster public trust, safety, and proactive, as opposed to reactive, AI risk and impact management.
- Prioritizes high-risk AI systems, pushing regulators and AI companies to funnel their attention and resources to AI impact areas with the greatest potential for harm.
- Outlines robust consumer protections, ensuring that consumers can preserve and enact their civil rights when they are on the receiving end of consequential AI-driven decision-making outcomes or in the midst of AI interactions, whether conscious or unconscious.
- Assigns distinct responsibilities to key actors involved in the AI lifecycle, namely developers, distributors, and deployers, ensuring that accountability standards are applied uniformly throughout the AI lifecycle and that responsibility-based ambiguities are minimized.
- Balances the need for AI innovation with safety by affording eligible regulatory sandbox applicants the ability to conduct controlled pre-deployment safety testing of their AI systems while being temporarily exempt from compliance requirements.
- Envisions workforce development initiatives that strive to address pragmatic concerns pertinent to AI and the future of work, reducing automation-induced job displacement, lowering the probability of a digital divide, and equipping businesses and educational institutions with the resources and channels necessary for AI training, upskilling, and professional development.
- Creates the Texas AI Council as the central governing body regarding all things AI within the state of Texas, laying the groundwork for a state-level AI governance structure and strategy that remains consistent, forward-looking, agile, and transparent.
Weaknesses
Notwithstanding its comprehensive scope, TRAIGA is far from perfect, omitting important details throughout several areas, failing to provide tangible clarification on ambiguous requirements or concepts, excluding certain AI systems known to pose substantial risks, and supporting a heavy-handed AI governance approach that is both impractical and excessive. With respect to its key weaknesses, TRAIGA:
- Explicitly prohibits certain AI uses like social scoring and unlawful biometric surveillance, focusing on specific AI use cases when such practices, independent of AI, should be banned as a whole. This creates a massive regulatory loophole.
- Exempts small businesses from compliance requirements, implicitly assuming that entities like startups will not have the resources and expertise to deploy and/or modify AI systems that could perpetuate widespread harmful impacts. This possibility already exists today and will only increase as AI systems become more powerful.
- Does not provide detailed descriptions of regulatory sandbox provisions, including eligibility criteria, evaluation metrics, and enforcement mechanisms, increasing the possibility of inconsistent oversight and/or potentially limited engagement.
- Circularly and vaguely defines high-risk AI systems, introducing ambiguities that regulators could exploit to enact stringent controls and penalties upon AI developers and deployers whose systems may not be as risky as they seem. By contrast, AI developers and deployers might also exploit these ambiguities for their benefit, finding ways to circumvent compliance requirements despite knowing their systems may classify as high-risk.
- Narrowly focuses on high-risk AI systems, deliberately overlooking the potential cumulative and/or scalable societal, political, and economic impacts of relatively low-risk but proliferous AI systems.
- Fails to cover general-purpose AI systems and AI agents, both of which represent the AI frontier and inspire a conglomeration of interconnected short and long-term risks, benefits, and impacts that can manifest at vastly different scales across multiple domains and timelines.
- Imposes a major compliance burden on developers and deployers which could significantly hinder adoption efforts, especially since developers only have 30 days to address violations via corrective action—for complex AI systems, this is not a realistic timeframe.
- Affords the Texas AI Council and Attorney General with enormous power, setting the stage for regulatory oversight and enforcement practices that could subvert businesses’ AI transformation efforts and preclude consumers from accessing AI benefits.
Impacts
Regulatory, Compliance & Legal
- Developers, particularly those who are smaller and/or entering the market, will have to manage high costs for documentation, testing, and compliance with risk frameworks. This will introduce administrative, operational, and infrastructure-related challenges that will require careful navigation to avoid potential compliance penalties, introducing major innovation delays.
- Deployers will be forced to handle recurring compliance burdens in the form of impact assessments, consumer disclosures, and post-deployment monitoring measures. For deployers that deploy multiple complex systems, addressing these requirements effectively and efficiently could become extremely difficult and convoluted.
- Distributors, being responsible for ensuring that AI systems available on the market remain compliant, could be required to develop due diligence processes like reviewing high-risk reports and monitoring product use. Doing so in the absence of federal and/or actionable standards could introduce indirect compliance challenges for which no clear resolutions yet exist.
- Core oversight bodies—the Texas AI Council and Attorney General—will find that emerging administrative needs, enforcement demands, and regulatory uncertainty strain their existing resources and talent. This will highlight the need for robust and well-funded interagency communication, resource, and talent acquisition channels.
- Aggressive compliance incentives, namely 30-day cure periods, the rebuttable presumption of care, and costly penalties will incentivize proactive compliance for stakeholders who have the necessary resources and expertise to manage compliance burdens.
Economic
- High compliance costs create a potent economic incentive for prioritizing ethical and transparent AI practices, however, smaller companies that struggle to afford these costs will either be crippled by them or absorbed by their larger competitors. This could drive a concentration of power at the top and hinder innovation among emerging businesses.
- Regulatory sandboxes will be moderately effective in promoting economic growth while maintaining innovation safety. However, the lack of detailed sandbox provisions could perpetuate entry barriers that introduce unexpected inefficiencies and continued oversight and validation challenges.
- Market differentiation dynamics will emerge, favoring compliant companies who will market themselves as trustworthy and ethical AI leaders to build upstanding reputations and attract customers and funding. However, these reputations will be more fragile than expected, particularly for deployers who face recurring compliance burdens.
- Workforce development initiatives will expand AI opportunities early on, but their effectiveness will be brought into question as AI-related demands quickly increase in the absence of explicitly defined and adequate funding and industry collaboration channels. Whether these initiatives effectively target underserved communities will also depend on program reach, which in a state as geographically expansive as Texas, represents a true pragmatic concern.
- High-risk and critical infrastructure sectors, such as healthcare and energy, being held to stringent regulatory standards, will see improvements in AI reliability and trust. However, sector-specific compliance requirements could decelerate AI-driven innovation across these sectors, particularly where TRAIGA provisions are misaligned with or contradict sector-specific requirements.
Technological
- Explicit alignment with AI risk management frameworks will support and uphold systematic approaches to risk prediction, identification, management, documentation, and reporting; transparency, accountability, and human oversight; bias mitigation, and performance testing and validation. However, complications throughout the development lifecycle could stifle smaller companies and independent developers, especially when disclosure requirements are perceived to create competitive disadvantages.
- Deployed AI systems will become far more reliable and accountable as a result of robust impact assessment and post-deployment monitoring provisions. By contrast, early compliance attempts will quickly reveal insufficient AI infrastructure and personnel as the costs and complexities of ongoing assessments build, fueling substantial deployment delays.
- Support for open-source AI and regulatory sandboxes will encourage responsible AI innovation and experimentation, but to a lesser extent than expected. Indirect open-source compliance costs and unclear sandbox eligibility and testing criteria could perpetuate an inconsistent and unpredictable AI innovation ecosystem.
- Cybersecurity assessments, despite the necessary costs they introduce, will ensure that high-risk AI systems remain robust and secure in adversarial and/or changing conditions. These impacts will be most prominent among critical sectors like energy and water.
- Algorithmic fairness and bias mitigation requirements will support the development of AI systems that are inherently more equitable and just. However, in the attempt to comply with these requirements, stakeholders will frequently overcorrect, creating systems that although non-discriminatory, produce faulty and/or inconsistent outputs.
Social & Ethical
- Fairness and equity will become a cornerstone of AI development and deployment, protecting vulnerable groups from being exploited or discriminated against while continually fortifying bias mitigation efforts. Still, this emphasis could create a culture where equity and inclusion supersede merit, which could drive damaging decision-making in contexts like hiring, where competence is paramount.
- Early-stage consumer protections will chart the path toward a new class of civil rights, specific to advanced AI systems, and intended to help us navigate a future where our cultural and moral paradigms no longer capture society’s best interests. In navigating this path, we will find that our binary moral structures prove inadequate, forcing us to begin rebuilding the foundation of our moral reasoning to include more pluralistic and less normative value structures.
- Despite being entitled to AI-specific rights such as opting out of data processing and appealing AI decisions, most consumers will either be unaware that they have these rights or remain ignorant as to how to exercise them. This will push regulators to go beyond rudimentary and ambiguous requirements like “clear and conspicuous” disclosures, providing stakeholders with obvious examples of how to communicate AI information appropriately.
- By bridging the digital divide, TRAIGA will play a notable role in the democratization of AI knowledge, skills, and opportunities, subsidizing a citizenry that is more empowered, informed, prepared, and engaged with the complexities of AI innovation. Nonetheless, exercising this role successfully will require access to a wealth of in-depth and customized resources on crucial topics like AI literacy, most of which simply do not exist yet.
- Government AI initiatives will be held to the same standards as corporate AI initiatives, allowing citizens to hold both companies and governments accountable for their AI-related actions. However, we are currently in a period where collective trust and confidence in existing institutions is very low, so even if governments transparently adhere to ethical AI practices, citizens will be unlikely to accept this as proof of positive intentions—from a social perspective, governments will have a higher burden of proof threshold.
Long-Term
- By aligning with AI governance gold standards like the EU AI Act and NIST AI Risk Management Framework, TRAIGA encourages the standardization of globally recognized AI governance best practices, enabling enhanced inter-system consistency, interoperability, and assessment while also positioning Texas as a national AI governance leader.
- Long-term economic stability and reduced automation-induced job loss, due to the emergence of compliant AI industries, ethical AI as a selling point, and successful workforce development initiatives, could establish Texas as the US’ sustainable and responsible AI innovation hub. This status would attract numerous high-impact AI startups and denote Texas as an if not “the” national authority on AI governance.
- TRAIGA will incentivize research on AI ethics, governance, and safety, not only through its direct support for transparency, accountability, fairness, and risk management, but also through its establishment of the Texas AI Council, which, if it is to perform its role effectively, will require steady updates on AI advancements, agile and proactive governance practices, and emerging AI risks and impacts.
- General-purpose AI models and AI agents will remain largely unregulated, and even if some regulation is developed, it will target use cases not the advanced capabilities these systems possess, which could be easily exploited to instigate severe harm. AI agents will also proliferate throughout professional settings at an accelerated rate, becoming deeply embedded within the workforce before regulators have any chance to establish reliable compliance parameters.
- Focus on immediate AI risks will undermine interest in long-term risk trajectories, particularly those operating at systemic and existential scales. These risks are much closer than we think, especially as whispers of AGI continue to gain legitimacy among leading AI developers.
- AI governance research and development will stagnate as it becomes clear that binary value structures no longer holistically describe, categorize, or anticipate the risks and impacts posed by future AI systems, which will dwarf those we have today, even 6 months from now. AI governance, particularly at the level of actionable policy, will also struggle to account for the rapidly growing interconnectedness and interdependency cultivated by complex AI systems, even within localized environments like individual companies.
- Sustainable AI initiatives will be largely abandoned, not because AI won’t become more efficient, but because widespread adoption throughout critical sectors will reduce operational, supply chain, infrastructure, and manufacturing inefficiencies to such a degree that sustainability concerns will be outweighed. Continued advances in compute infrastructure will also drive the development of less energy-intensive AI systems, which although they still consume enormous amounts of energy, will be orders of magnitude more efficient than their predecessors.
- Large-scale data centers, such as those proposed by President Trump’s Project Stargate, which intends to support national AI infrastructure, will become a key national security risk. These data centers will likely be the first targets that foreign adversaries select in their attempts to beat the US in the race toward artificial general intelligence (AGI). Precise federal regulations and standards regarding the security and robustness of these data centers nationwide will need to be established as soon as possible.
Suggestions and Conclusion
Building on our discussion of TRAIGA’s strengths, weaknesses, and potential impacts, we offer the following suggestions for improving the act:
- Define a precise and comprehensive tiered risk classification framework that covers all kinds of AI systems that exist today in addition to those we expect will exist within the next decade. High-risk AI systems can’t be defined simply according to their role in consequential decision-making.
- Assure that general-purpose AI systems, AI agents, and AGI are explicitly defined and covered. The goal isn’t to get these definitions “right” but rather, to ensure that working definitions and governance practices exist so that when these systems advance and/or proliferate, a general framework for regulating them can be applied and then adapted accordingly.
- Instead of outlawing certain AI use cases, outlaw the very practices that these use cases would support, like unchecked surveillance, election manipulation, and social credit scoring. Outlawing such practices would also represent the first step in building governance structures and principles that help us safely navigate a future with AGI.
- Dramatically expand the scope of risks covered to include national security, systemic, and existential threats. Once more, the idea here isn’t to capture all possible AI-induced risk trajectories but to have certain foundational provisions in place when we do encounter them.
- Specify, in detail, regulatory sandbox criteria at every stage of participation, from application to exit. Specific criteria will encourage engagement and support consistent testing and evaluation processes during sandbox participation.
- Slightly lower the compliance burden that AI developers and deployers face, by moderately reducing compliance penalties, eliminating data reporting requirements, and extending the cure period for violations to a minimum of 90 days. All other risk and impact-related requirements should be maintained.
- Develop concrete mechanisms through which AI companies and citizens can provide direct feedback to the Texas AI Council, penalizing the council if it does not respond to this feedback appropriately.
- Require the Texas AI Council to publicly disclose the logic, information, intent, and individuals involved in AI governance-related decisions, updates, and recommendations to guarantee unobstructed transparency into council practices and appropriately manage its power and influence.
- To foster public trust and quality assurance, Texas AI Council members should not be selected by government officials, but by recognized and independent AI experts, who have pledged, under penalty of perjury, that they do not have any vested interest in their selection of certain council candidates. Government officials should remain responsible for approving and instating selected candidates.
- Set clear funding and resource channel priorities to ensure that workforce development initiatives are well subsidized. Funding and resource prioritization should be proactive and informed by demographically diverse demand forecasting analyses that look several years into the future.
- Develop and pilot AI literacy campaigns across multiple regions and subpopulations to probe their effectiveness and inform the content of workforce development initiatives. These AI literacy campaigns would also test whether outreach strategies are effective.
- Define and implement AI literacy metrics for measuring the success of workforce development initiatives. Generic metrics such as number of new jobs created or employee turnover rates will fail to capture the full picture.
- Create and establish funding and talent channels that are exclusively reserved for AI governance, ethics, and safety research to guarantee that further developments across these fields do not stagnate as AI advancements elevate the uncertainty and complexity of these issues.
We leave readers with these suggestions, though there are surely many more that could be made depending on how far into the future we’re willing to look. Regardless, for those interested in further exploring AI policies, governance, ethics, and safety, we suggest following Lumenova’s blog, where numerous resources examining an expansive array of both present and future-oriented topics are at your disposal.
On the other hand, if you’re already considering or engaged in developing AI governance and risk management frameworks, strategies, and/or policies, we invite you to check out Lumenova’s RAI platform and book a product demo today. For the curious ones, we further invite you to experiment with our AI policy analyzer and risk advisor.